An Effective Learning Scheme for Weighted-BP with Parallel Permutation Decoding
2020 International Symposium on Information Theory and Its Applications (ISITA)(2020)
摘要
Weighted-BP is a method to improve the decoding performance of belief-propagation (BP) by learning its appropriate message weights using deep learning technique. For binary primitive BCH codes, it has been reported in literature that their performance can be enhanced more through parallel decoding which is achieved by exploiting the automorphism property of the codes. In this paper, an effective learning scheme for the parallel weighted-BP is proposed. While in the conventional work the weights preliminarily learned for a single weighted-BP were utilized as is even in the scenario of parallel decoding, we show that some gain can be further obtained if we take the parallel structure into account in the learning phase.
更多查看译文
关键词
weighted-BP,parallel permutation decoding,decoding performance,appropriate message weights,deep learning technique,binary primitive BCH codes,parallel decoding,effective learning scheme
AI 理解论文
溯源树
样例
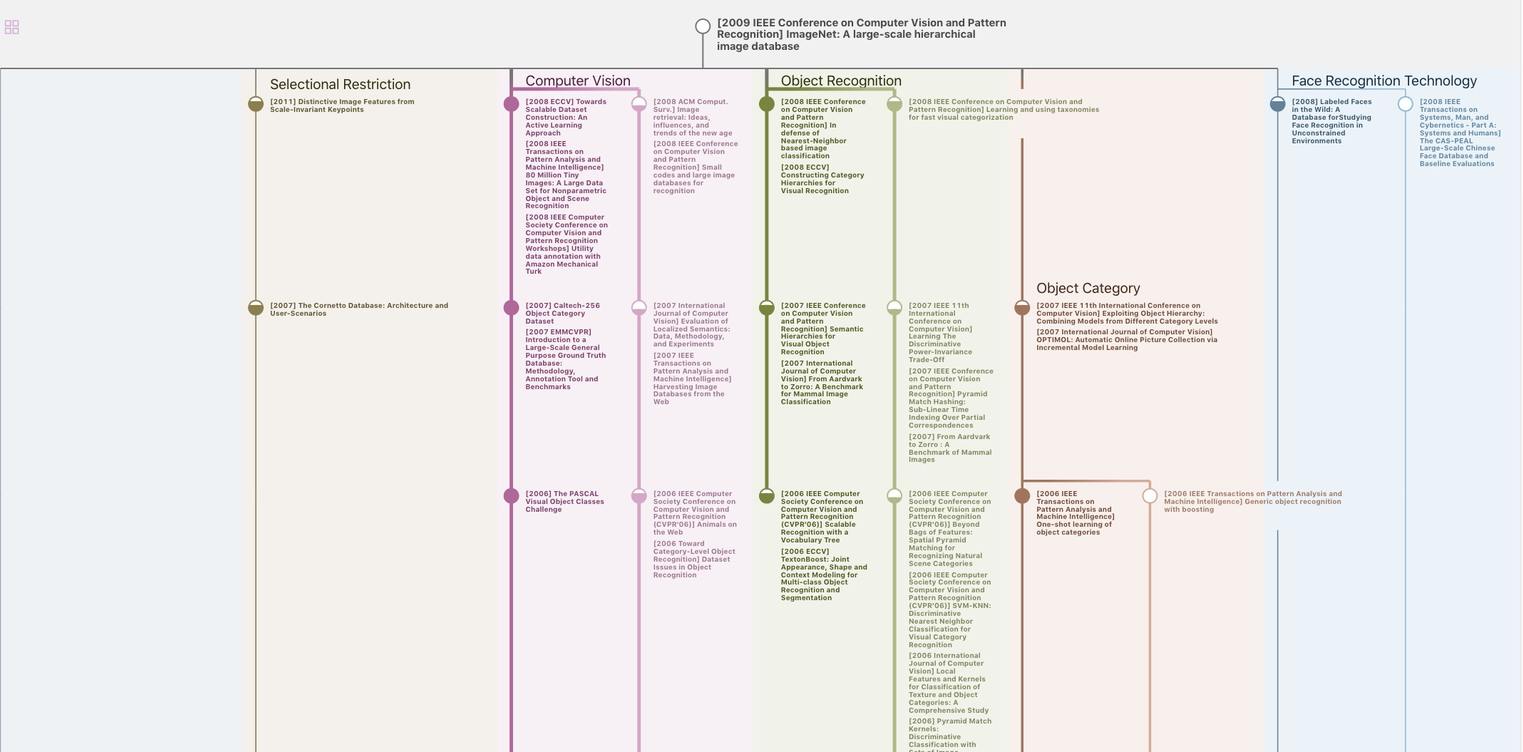
生成溯源树,研究论文发展脉络
Chat Paper
正在生成论文摘要