Pairwise Adjusted Mutual Information
arxiv(2021)
摘要
A well-known metric for quantifying the similarity between two clusterings is the adjusted mutual information. Compared to mutual information, a corrective term based on random permutations of the labels is introduced, preventing two clusterings being similar by chance. Unfortunately, this adjustment makes the metric computationally expensive. In this paper, we propose a novel adjustment based on {pairwise} label permutations instead of full label permutations. Specifically, we consider permutations where only two samples, selected uniformly at random, exchange their labels. We show that the corresponding adjusted metric, which can be expressed explicitly, behaves similarly to the standard adjusted mutual information for assessing the quality of a clustering, while having a much lower time complexity. Both metrics are compared in terms of quality and performance on experiments based on synthetic and real data.
更多查看译文
关键词
mutual information
AI 理解论文
溯源树
样例
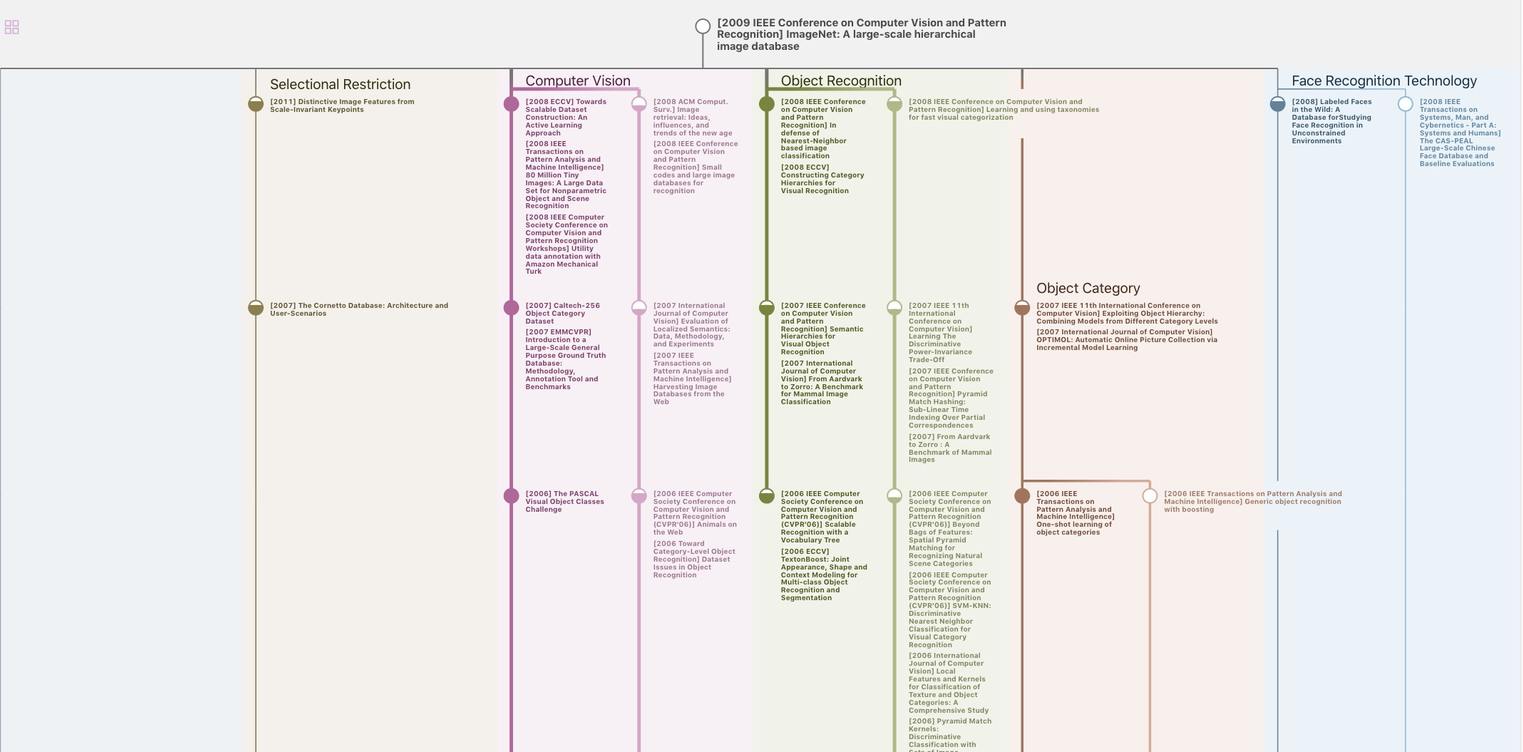
生成溯源树,研究论文发展脉络
Chat Paper
正在生成论文摘要