The Gradient Convergence Bound of Federated Multi-Agent Reinforcement Learning With Efficient Communication
IEEE TRANSACTIONS ON WIRELESS COMMUNICATIONS(2024)
摘要
The paper considers independent reinforcement learning (IRL) for multi-agent collaborative decision-making in the paradigm of federated learning (FL). However, FL generates excessive communication overheads between agents and a remote central server, especially when it involves a large number of agents or iterations. Besides, due to the heterogeneity of independent learning environments, multiple agents may undergo asynchronous Markov decision processes (MDPs), which will affect the training samples and the model's convergence performance. On top of the variation-aware periodic averaging (VPA) method and the policy-based deep reinforcement learning (DRL) algorithm (i.e., proximal policy optimization (PPO)), this paper proposes two advanced optimization schemes orienting to stochastic gradient descent (SGD): 1) A decay-based scheme gradually decays the weights of a model's local gradients with the progress of successive local updates, and 2) By representing the agents as a graph, a consensus-based scheme studies the impact of exchanging a model's local gradients among nearby agents from an algebraic connectivity perspective. This paper also provides novel convergence guarantees for both developed schemes, and demonstrates their superior effectiveness and efficiency in improving the system's utility value through theoretical analyses and simulation results.
更多查看译文
关键词
Independent reinforcement learning,federated learning,consensus algorithm,communication overheads
AI 理解论文
溯源树
样例
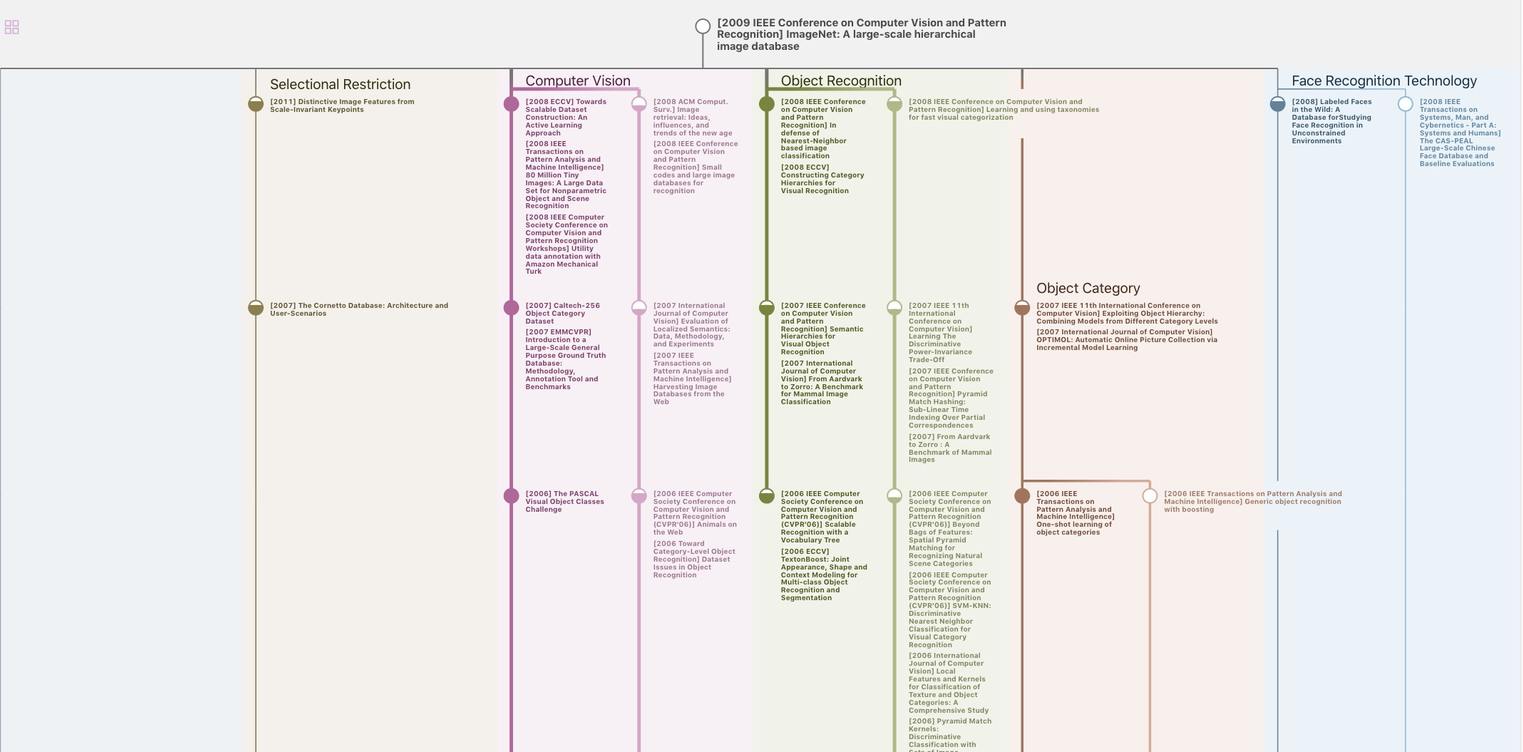
生成溯源树,研究论文发展脉络
Chat Paper
正在生成论文摘要