OTCE: A Transferability Metric for Cross-Domain Cross-Task Representations
2021 IEEE/CVF CONFERENCE ON COMPUTER VISION AND PATTERN RECOGNITION, CVPR 2021(2021)
摘要
Transfer learning across heterogeneous data distributions (a.k.a. domains) and distinct tasks is a more general and challenging problem than conventional transfer learning, where either domains or tasks are assumed to be the same. While neural network based feature transfer is widely used in transfer learning applications, finding the optimal transfer strategy still requires time-consuming experiments and domain knowledge. We propose a transferability metric called Optimal Transport based Conditional Entropy (OTCE), to analytically predict the transfer performance for supervised classification tasks in such cross-domain and cross-task feature transfer settings. Our OTCE score characterizes transferability as a combination of domain difference and task difference, and explicitly evaluates them from data in a unified framework. Specifically, we use optimal transport to estimate domain difference and the optimal coupling between source and target distributions, which is then used to derive the conditional entropy of the target task (task difference). Experiments on the largest cross-domain dataset DomainNet and Office31 demonstrate that OTCE shows an average of 21% gain in the correlation with the ground truth transfer accuracy compared to state-of-the-art methods. We also investigate two applications of the OTCE score including source model selection and multi-source feature fusion.
更多查看译文
关键词
target distributions,ground truth transfer accuracy,transferability metric,cross-domain cross-task representations,heterogeneous data distributions,transfer learning,neural network,supervised classification tasks,OTCE score,DomainNet,optimal transport based conditional entropy
AI 理解论文
溯源树
样例
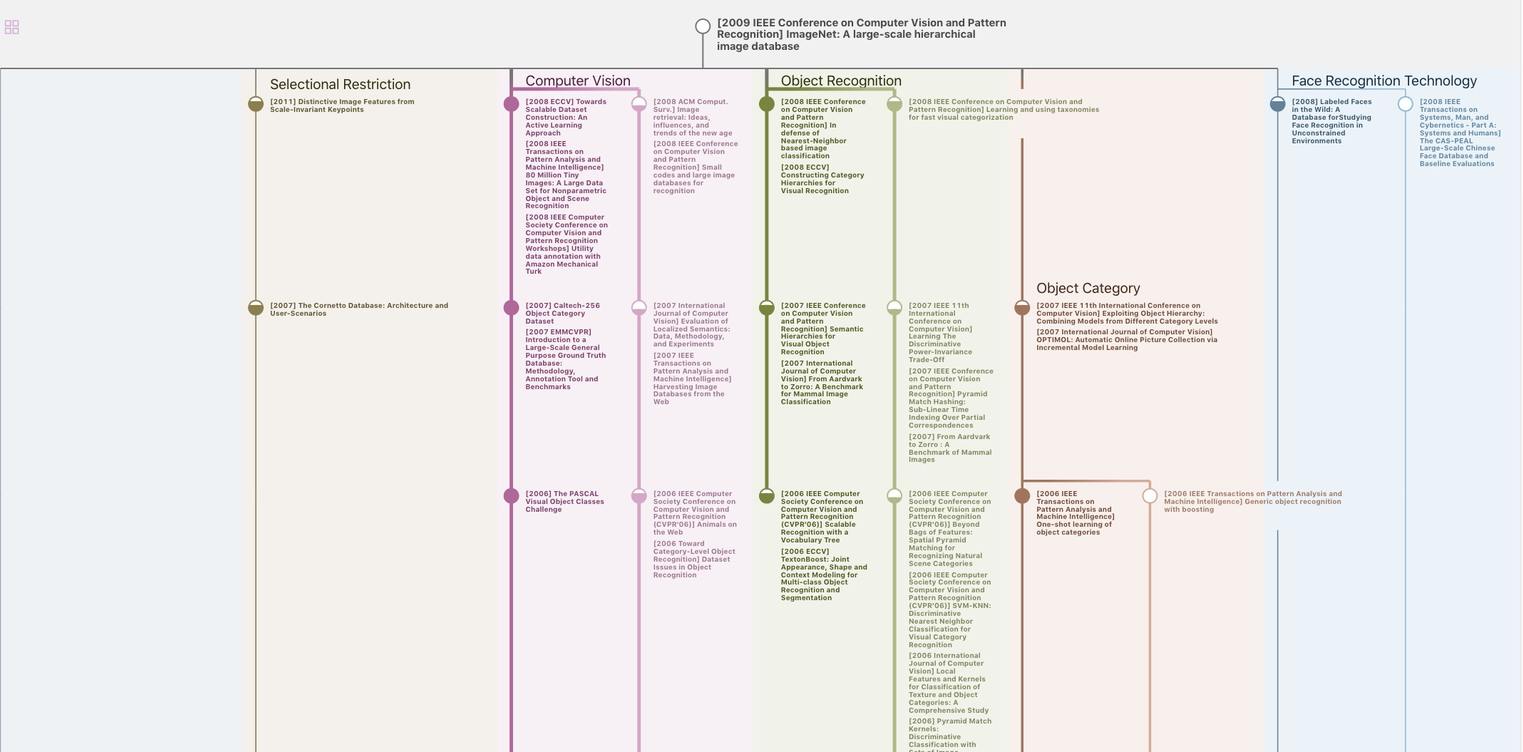
生成溯源树,研究论文发展脉络
Chat Paper
正在生成论文摘要