Exemplar-model account of categorization and recognition when training instances never repeat.
Journal of experimental psychology. Learning, memory, and cognition(2022)
摘要
In a novel version of the classic dot-pattern prototype-distortion paradigm of category learning, Homa et al. (2019) tested a condition in which individual training instances never repeated, and observed results that they claimed severely challenged exemplar models of classification and recognition. Among the results was a dissociation in which participants classified transfer items with high accuracy in the no-repeat condition, yet in old-new recognition tests showed no ability to discriminate between old and new items of the same level of distortion from the prototype. In addition, speed of classification learning was no faster in a condition in which a small set of training instances was repeated continuously compared with the no-repeat condition. Here we show through computer-simulation modeling that exemplar models naturally capture the classification-recognition dissociation in the no-repeat condition, as well as a wide variety of other qualitative effects reported by Homa et al. (2019). We also conduct new conceptual-replication experiments to investigate their reported null effect of repeated versus nonrepeated training instances on speed of classification learning. In contrast to Homa et al. (2019) we find that speed of learning is substantially faster in the repeat condition than in the no-repeat condition, precisely as exemplar models predict. The exemplar model also captures a wide variety of transfer effects observed following the completion of category learning, including the classification-recognition dissociation observed across the repeat and no-repeat conditions. (PsycInfo Database Record (c) 2023 APA, all rights reserved).
更多查看译文
关键词
categorization,recognition,exemplars,prototypes,computational modeling
AI 理解论文
溯源树
样例
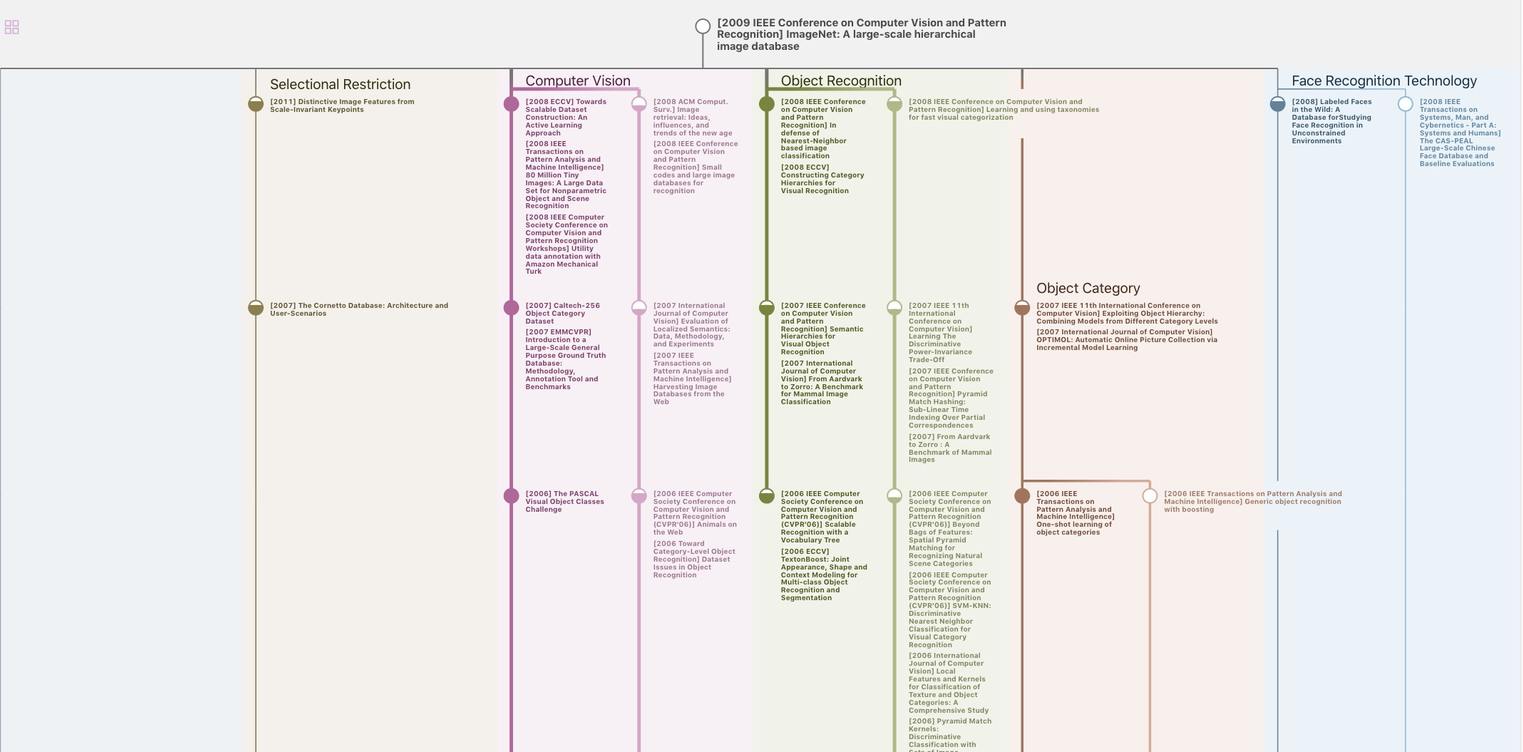
生成溯源树,研究论文发展脉络
Chat Paper
正在生成论文摘要