A Population Model for Academia: Case Study of the Computer Science Community Using DBLP Bibliography 1960-2016
IEEE Transactions on Emerging Topics in Computing(2018)SCI 2区
Chinese Univ Hong Kong | Virginia Tech
Abstract
The academic community has seen a tremendous growth in number of authors and publications in recent times. Most previous studies of the academic community, whether on individual activity, productivity and influence, or collaboration patterns and their implications, have been based on a flat and static view of the system. However, the academic community resembles a dynamic growing population, with entry and exit of authors. In this paper, we study this systemic inflation by proposing a population model for academia. We use a generalized branching process as an overarching framework, which enables us to describe the research community from an evolutionary and structural perspective. Further, the observed patterns allow us to shed light on researchers' life-cycle encompassing their arrival, academic life expectancy, activity, productivity and offspring distribution in the community. In our study, we used data from DBLP for a case study in the computer science community, although our methodology can be adopted in a systematic manner to any other research domain with sufficient publication records. We believe that the results can help academics and policy makers alike to better understand and evaluate the development and evolution of their respective academic communities.
MoreTranslated text
Key words
Computer science,Sociology,Statistics,Productivity,Databases,Special issues and sections,Big Data,Bibliographies,Branching processes,author life-cycle,academic community,population model
PDF
View via Publisher
AI Read Science
AI Summary
AI Summary is the key point extracted automatically understanding the full text of the paper, including the background, methods, results, conclusions, icons and other key content, so that you can get the outline of the paper at a glance.
Example
Background
Key content
Introduction
Methods
Results
Related work
Fund
Key content
- Pretraining has recently greatly promoted the development of natural language processing (NLP)
- We show that M6 outperforms the baselines in multimodal downstream tasks, and the large M6 with 10 parameters can reach a better performance
- We propose a method called M6 that is able to process information of multiple modalities and perform both single-modal and cross-modal understanding and generation
- The model is scaled to large model with 10 billion parameters with sophisticated deployment, and the 10 -parameter M6-large is the largest pretrained model in Chinese
- Experimental results show that our proposed M6 outperforms the baseline in a number of downstream tasks concerning both single modality and multiple modalities We will continue the pretraining of extremely large models by increasing data to explore the limit of its performance
Try using models to generate summary,it takes about 60s
Must-Reading Tree
Example
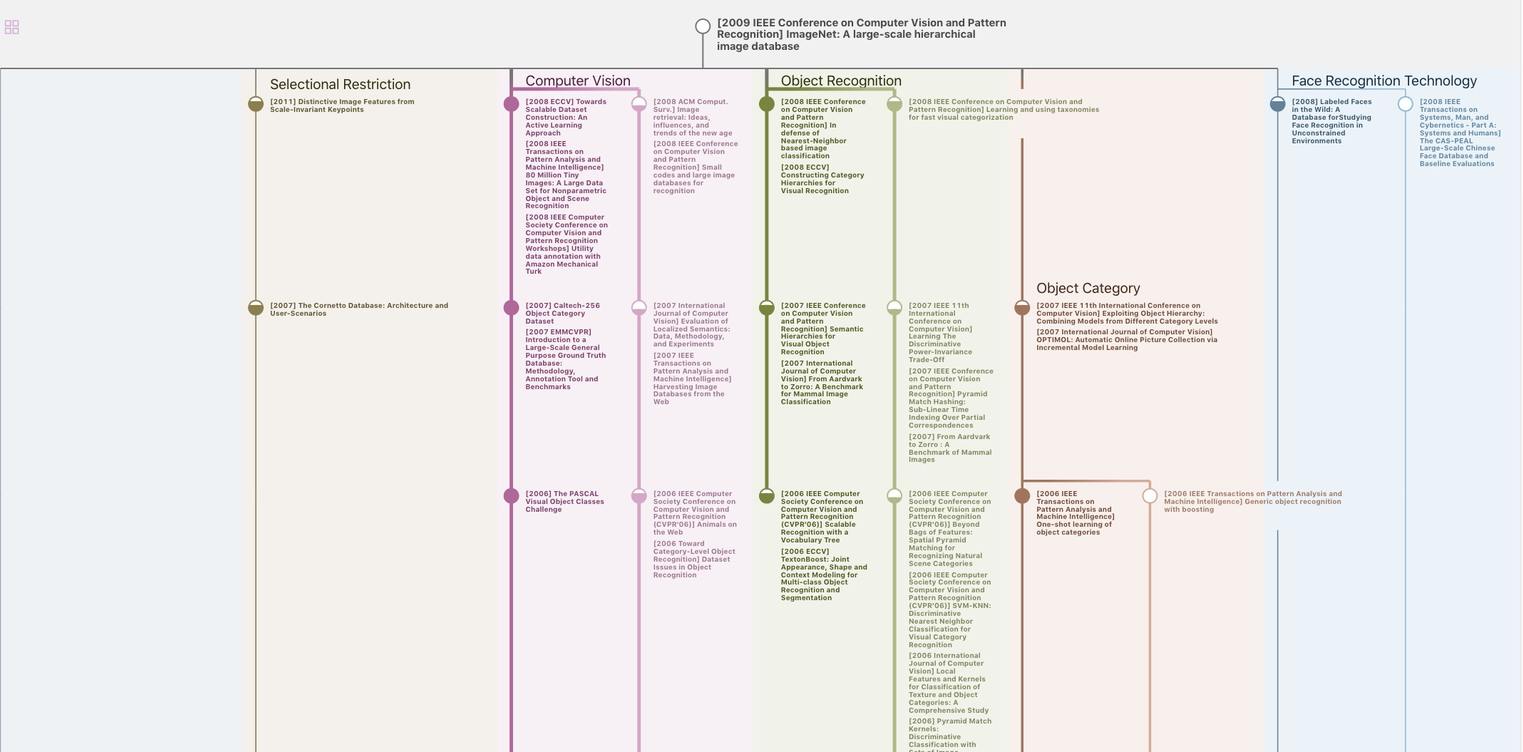
Generate MRT to find the research sequence of this paper
Related Papers
2019 IEEE 89th Vehicular Technology Conference (VTC2019-Spring) 2019
被引用12
Edge Learning Based Green Content Distribution for Information-Centric Internet of Things
2019 42ND INTERNATIONAL CONFERENCE ON TELECOMMUNICATIONS AND SIGNAL PROCESSING (TSP) 2019
被引用6
DICO: A Graph-DB Framework for Community Detection on Big Scholarly Data
IEEE Transactions on Emerging Topics in Computing 2019
被引用37
IEEE Access 2021
被引用2
Data Disclaimer
The page data are from open Internet sources, cooperative publishers and automatic analysis results through AI technology. We do not make any commitments and guarantees for the validity, accuracy, correctness, reliability, completeness and timeliness of the page data. If you have any questions, please contact us by email: report@aminer.cn
Chat Paper
GPU is busy, summary generation fails
Rerequest