Learning From Pixel-Level Label Noise: A New Perspective for Semi-Supervised Semantic Segmentation
IEEE Transactions on Image Processing(2022)
摘要
This paper addresses semi-supervised semantic segmentation by exploiting a small set of images with pixel-level annotations (strong supervisions) and a large set of images with only image-level annotations (weak supervisions). Most existing approaches aim to generate accurate pixel-level labels from weak supervisions. However, we observe that those generated labels still inevitably contain noisy labels. Motivated by this observation, we present a novel perspective and formulate this task as a problem of learning with pixel-level label noise. Existing noisy label methods, nevertheless, mainly aim at image-level tasks, which can not capture the relationship between neighboring labels in one image. Therefore, we propose a graph-based label noise detection and correction framework to deal with pixel-level noisy labels. In particular, for the generated pixel-level noisy labels from weak supervisions by Class Activation Map (CAM), we train a clean segmentation model with strong supervisions to detect the clean labels from these noisy labels according to the cross-entropy loss. Then, we adopt a superpixel-based graph to represent the relations of spatial adjacency and semantic similarity between pixels in one image. Finally we correct the noisy labels using a Graph Attention Network (GAT) supervised by detected clean labels. We comprehensively conduct experiments on PASCAL VOC 2012, PASCAL-Context, MS-COCO and Cityscapes datasets. The experimental results show that our proposed semi-supervised method achieves the state-of-the-art performances and even outperforms the fully-supervised models on PASCAL VOC 2012 and MS-COCO datasets in some cases.
更多查看译文
关键词
Semi-supervised semantic segmentation,label noise,graph neural network
AI 理解论文
溯源树
样例
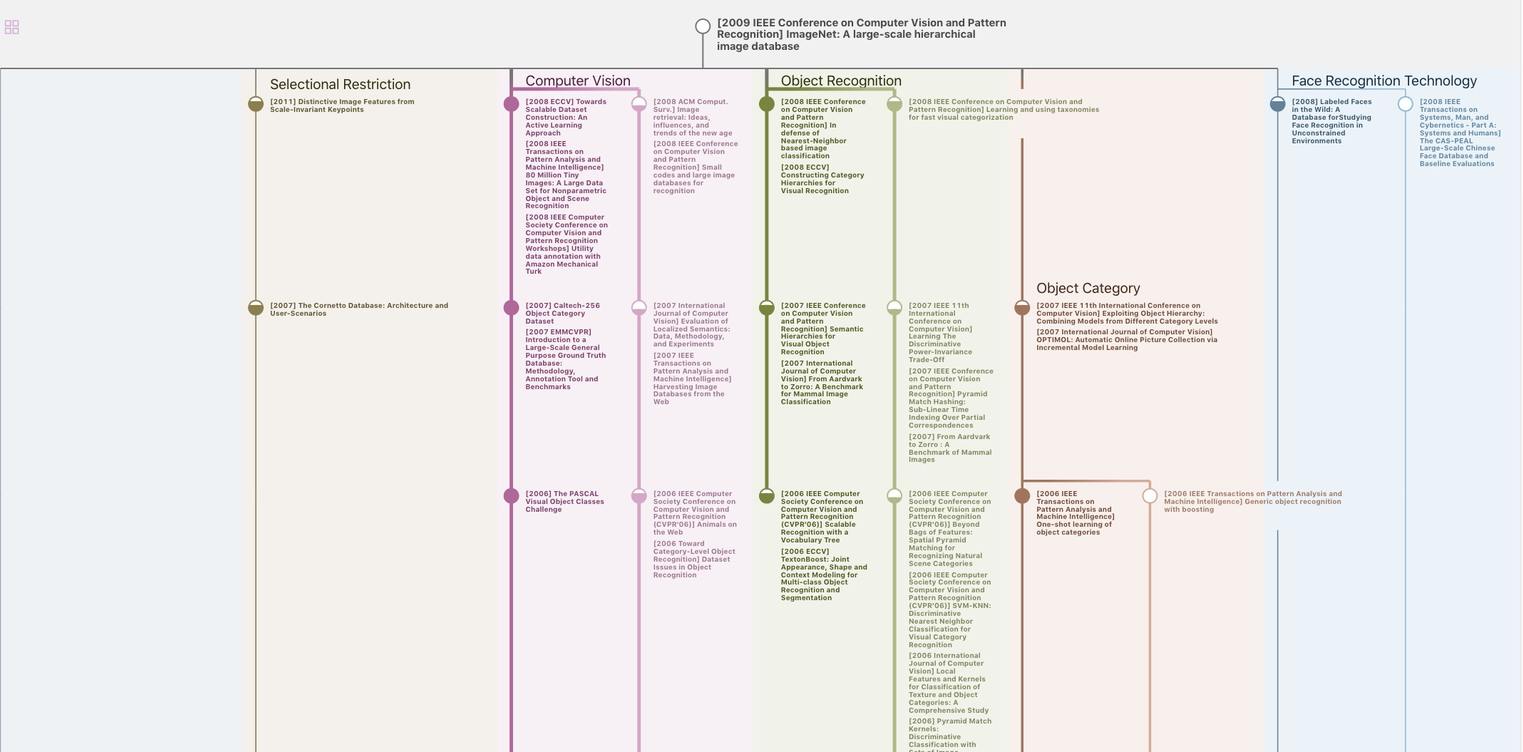
生成溯源树,研究论文发展脉络
Chat Paper
正在生成论文摘要