Continual Speaker Adaptation for Text-to-Speech Synthesis
arxiv(2021)
摘要
Training a multi-speaker Text-to-Speech (TTS) model from scratch is computationally expensive and adding new speakers to the dataset requires the model to be re-trained. The naive solution of sequential fine-tuning of a model for new speakers can cause the model to have poor performance on older speakers. This phenomenon is known as catastrophic forgetting. In this paper, we look at TTS modeling from a continual learning perspective where the goal is to add new speakers without forgetting previous speakers. Therefore, we first propose an experimental setup and show that serial fine-tuning for new speakers can result in the forgetting of the previous speakers. Then we exploit two well-known techniques for continual learning namely experience replay and weight regularization and we reveal how one can mitigate the effect of degradation in speech synthesis diversity in sequential training of new speakers using these methods. Finally, we present a simple extension to improve the results in extreme setups.
更多查看译文
关键词
adaptation,synthesis,text-to-speech
AI 理解论文
溯源树
样例
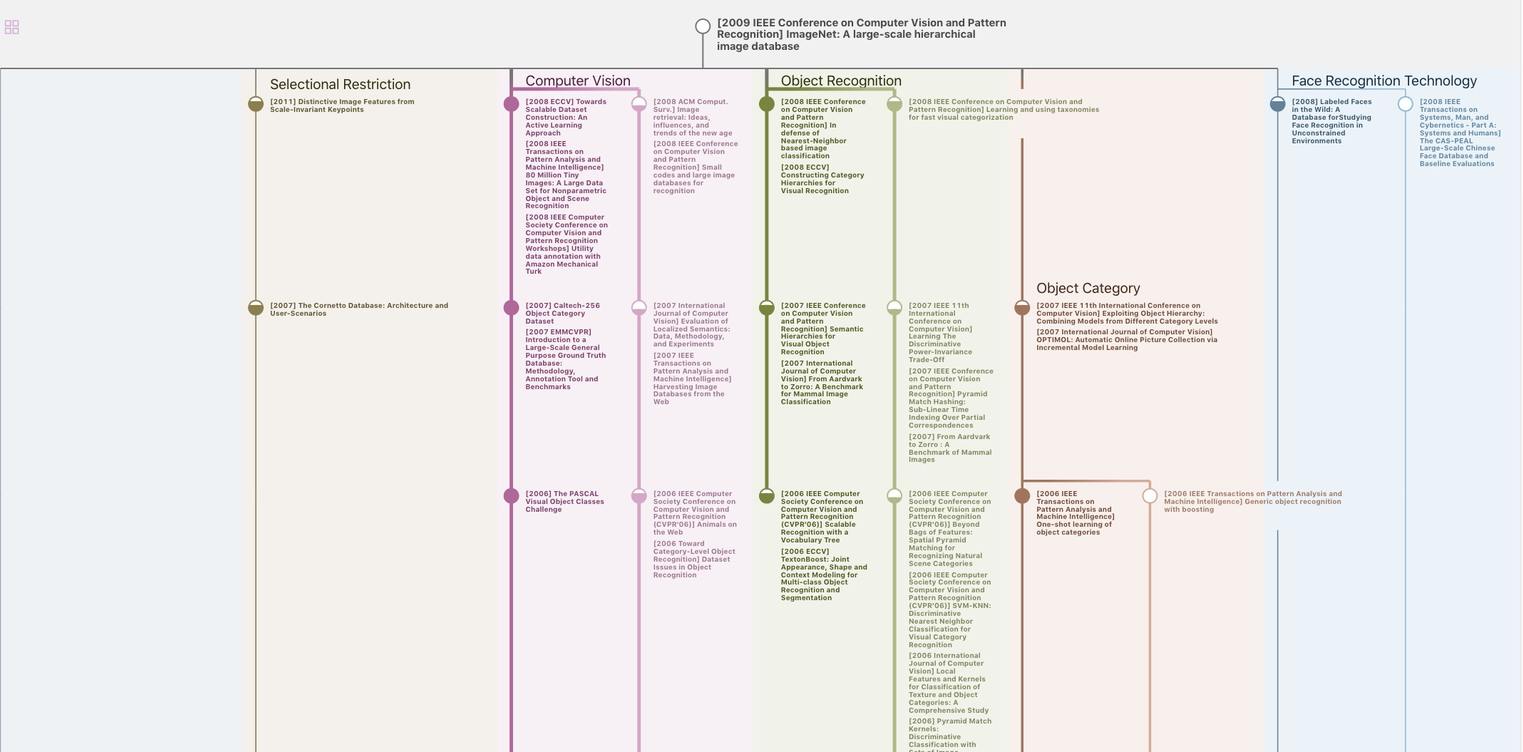
生成溯源树,研究论文发展脉络
Chat Paper
正在生成论文摘要