AUTOMATIC DIFFERENTIATION FOR RIEMANNIAN OPTIMIZATION ON LOW-RANK MATRIX AND TENSOR-TRAIN MANIFOLDS
SIAM JOURNAL ON SCIENTIFIC COMPUTING(2022)
摘要
In scientific computing and machine learning applications, matrices and more general multidimensional arrays (tensors) can often be approximated with the help of low-rank decompositions. Since matrices and tensors of fixed rank form smooth Riemannian manifolds, one of the popular tools for finding low-rank approximations is to use Riemannian optimization. Nevertheless, efficient implementation of Riemannian gradients and Hessians, required in Riemannian optimization algorithms, can be a nontrivial task in practice. Moreover, in some cases, analytic formulas are not even available. In this paper, we build upon automatic differentiation and propose a method that, given an implementation of the function to be minimized, efficiently computes Riemannian gradients and matrix-by-vector products between an approximate Riemannian Hessian and a given vector.
更多查看译文
关键词
automatic differentiation, Riemannian optimization, low-rank approximation, tensor-train decomposition
AI 理解论文
溯源树
样例
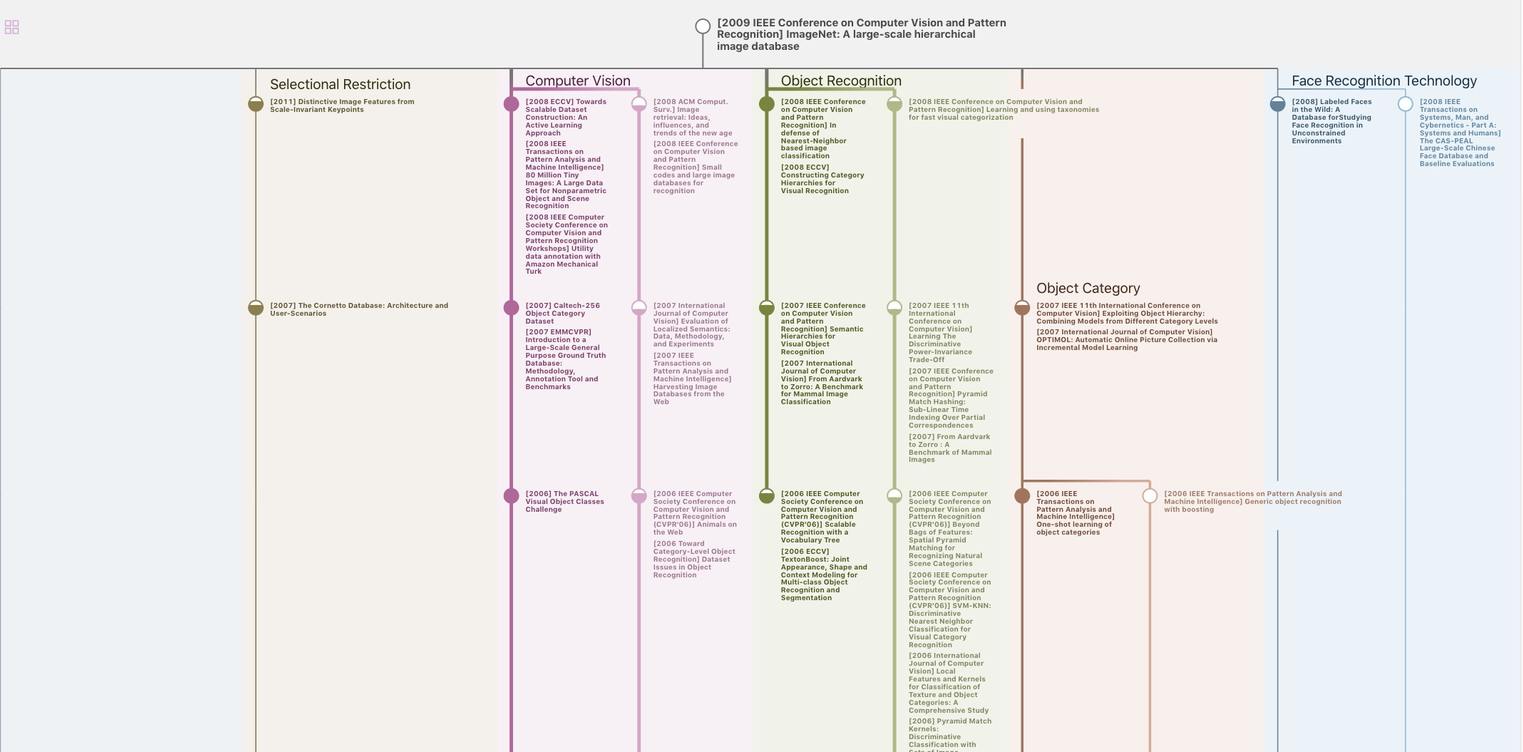
生成溯源树,研究论文发展脉络
Chat Paper
正在生成论文摘要