Consensus-based optimization methods converge globally in mean-field law
arxiv(2021)
摘要
In this paper we study consensus-based optimization (CBO), which is a metaheuristic derivative-free optimization method that can globally minimize nonconvex nonsmooth functions and is amenable to theoretical analysis. Based on an experimentally supported intuition that CBO performs a gradient descent on the convex envelope of a given objective, we derive a novel technique for proving the convergence to the global minimizer in mean-field law for a rich class of objective functions. Our results unveil internal mechanisms of CBO that are responsible for the success of the method. Furthermore, we improve prior analyses by requiring minimal assumptions about the initialization of the method and by covering objectives that are merely locally Lipschitz continuous. As a by-product of our analysis, we establish a quantitative nonasymptotic Laplace principle, which may be of independent interest.
更多查看译文
关键词
optimization methods,consensus-based
AI 理解论文
溯源树
样例
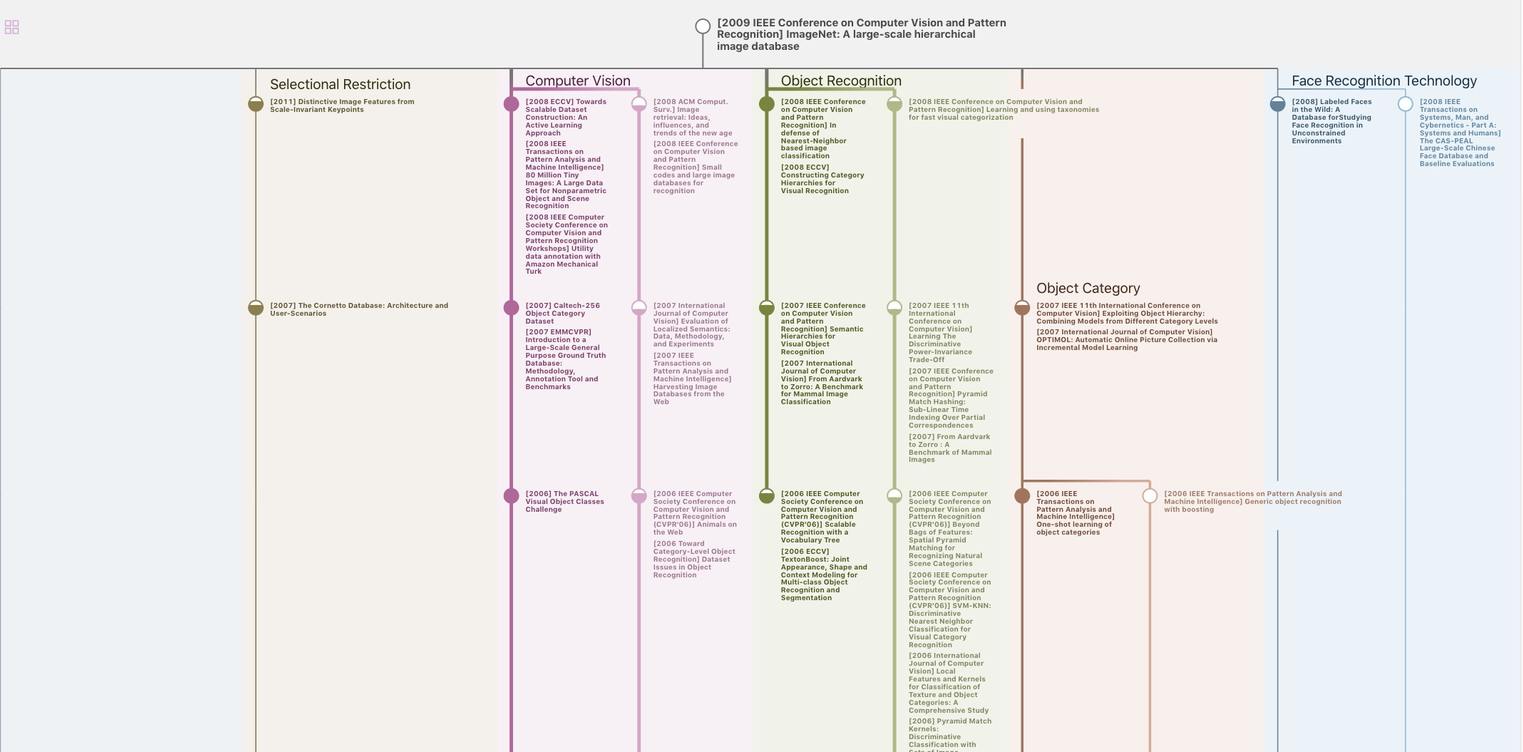
生成溯源树,研究论文发展脉络
Chat Paper
正在生成论文摘要