Simultaneous Reconstruction and Uncertainty Quantification for Tomography
arxiv(2023)
摘要
Tomographic reconstruction, despite its revolutionary impact on a wide range of applications, suffers from its ill-posed nature in that there is no unique solution because of limited and noisy measurements. Therefore, in the absence of ground truth, quantifying the solution quality is highly desirable but under-explored. In this work, we address this challenge through Gaussian process modeling to flexibly and explicitly incorporate prior knowledge of sample features and experimental noises through the choices of the kernels and noise models. Our proposed method yields not only comparable reconstruction to existing practical reconstruction methods (e.g., regularized iterative solver for inverse problem) but also an efficient way of quantifying solution uncertainties. We demonstrate the capabilities of the proposed approach on various images and show its unique capability of uncertainty quantification in the presence of various noises.
更多查看译文
关键词
tomography,simultaneous reconstruction,uncertainty quantification
AI 理解论文
溯源树
样例
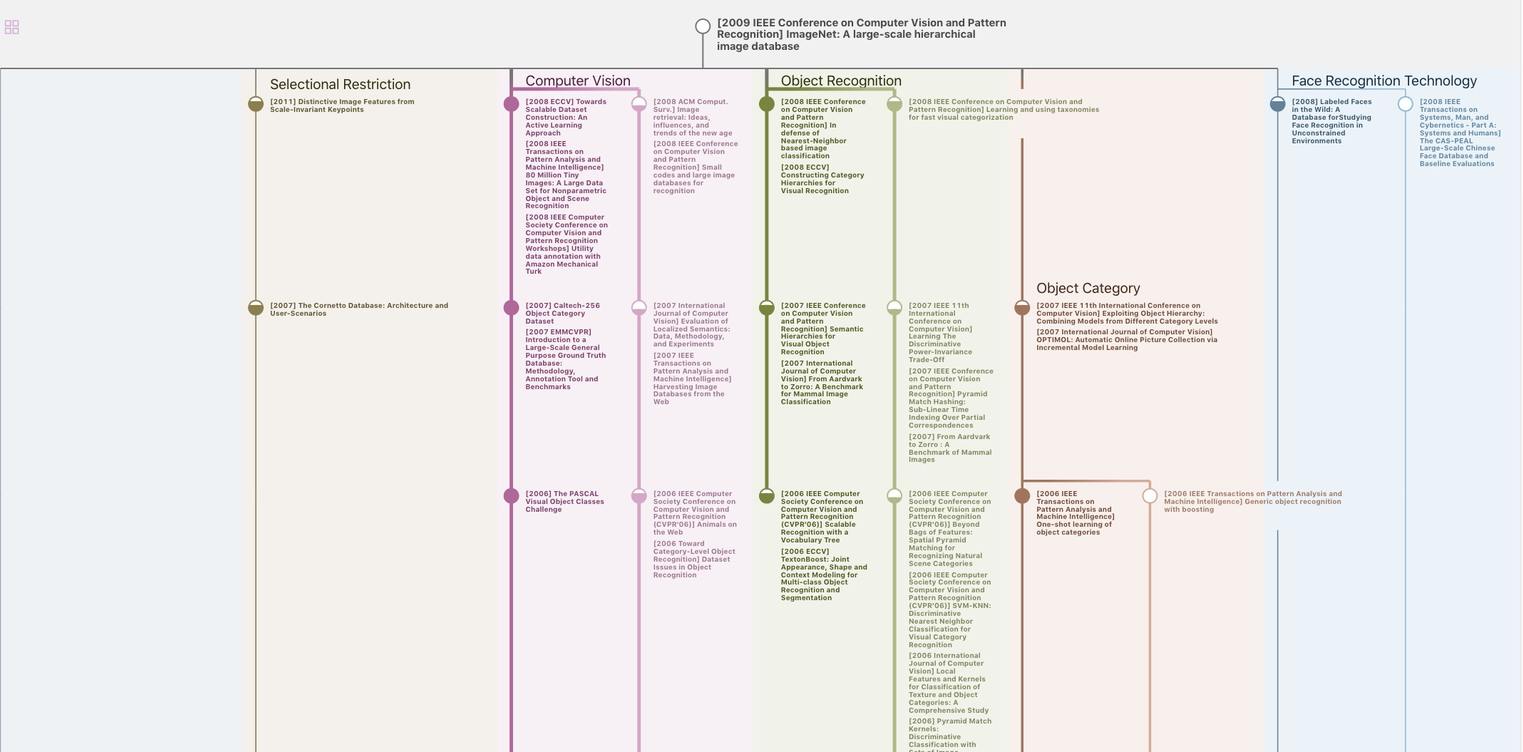
生成溯源树,研究论文发展脉络
Chat Paper
正在生成论文摘要