Cycle consistent network for end-to-end style transfer TTS training
Neural Networks(2021)
摘要
In this paper, we propose a cycle consistent network based end-to-end TTS for speaking style transfer, including intra-speaker, inter-speaker, and unseen speaker style transfer for both parallel and unparallel transfers. The proposed approach is built upon a multi-speaker Variational Autoencoder (VAE) TTS model. The model is usually trained in a paired manner, which means the reference speech is totally paired with the output including speaker identity, text, and style. To achieve a better quality for style transfer, which for most cases is in an unpaired manner, we augment the model with an unpaired path with a separated variational style encoder. The unpaired path takes as input an unpaired reference speech and yields an unpaired output. The unpaired output, which lacks direct ground-truth target, is then successfully constrained by a delicately designed cycle consistent network. Specifically, the unpaired output of the forward transfer is fed into the model again as an unpaired reference input, and after the backward transfer yields an output expected to be the same as the original unpaired reference speech. Ablation study shows the effectiveness of the unpaired path, separated style encoders and cycle consistent network in the proposed model. The final evaluation demonstrates the proposed approach significantly outperforms the Global Style Token (GST) and VAE based systems for all the six style transfer categories, in metrics of naturalness, speech quality, similarity of speaker identity, and similarity of speaking style.
更多查看译文
关键词
Speech synthesis,End-to-end,Style transfer,Variational autoencoder,Cycle consistent
AI 理解论文
溯源树
样例
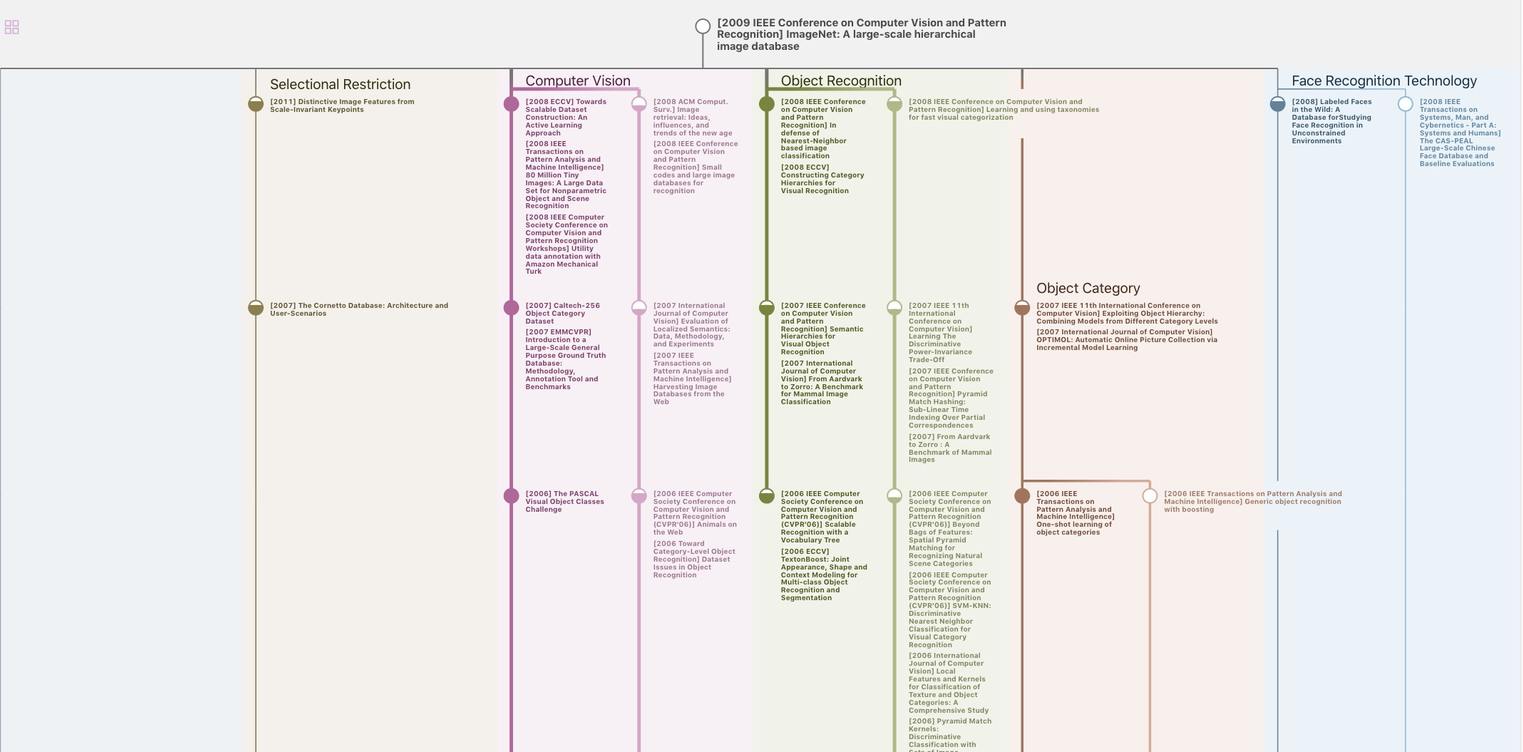
生成溯源树,研究论文发展脉络
Chat Paper
正在生成论文摘要