EnergyVis: Interactively Tracking and Exploring Energy Consumption for ML Models
Conference on Human Factors in Computing Systems(2021)
摘要
BSTRACT The advent of larger machine learning (ML) models have improved state-of-the-art (SOTA) performance in various modeling tasks, ranging from computer vision to natural language. As ML models continue increasing in size, so does their respective energy consumption and computational requirements. However, the methods for tracking, reporting, and comparing energy consumption remain limited. We present EnergyVis, an interactive energy consumption tracker for ML models. Consisting of multiple coordinated views, EnergyVis enables researchers to interactively track, visualize and compare model energy consumption across key energy consumption and carbon footprint metrics (kWh and CO2), helping users explore alternative deployment locations and hardware that may reduce carbon footprints. EnergyVis aims to raise awareness concerning computational sustainability by interactively highlighting excessive energy usage during model training; and by providing alternative training options to reduce energy usage.
更多查看译文
关键词
machine learning, environmental sustainability, interactive visualization, computational equity
AI 理解论文
溯源树
样例
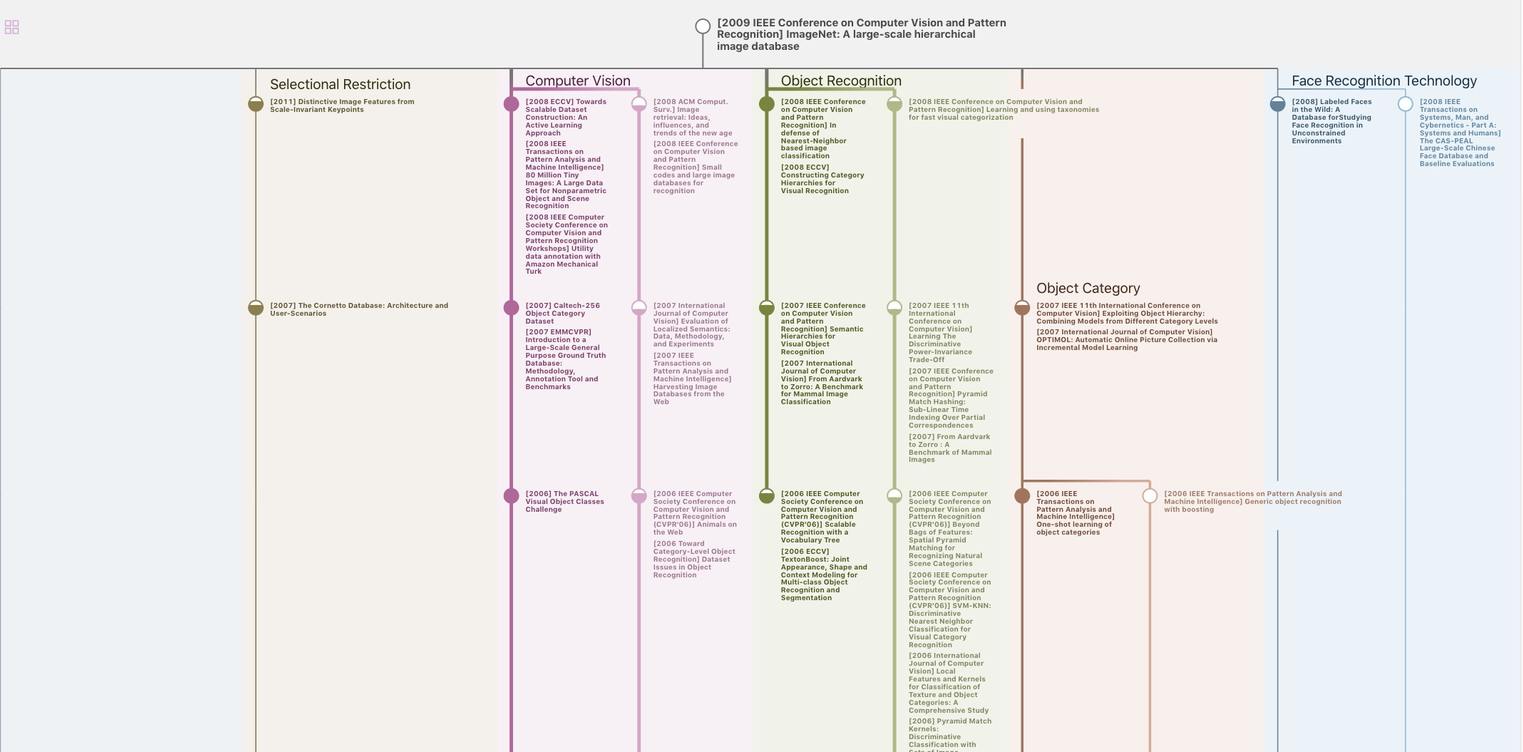
生成溯源树,研究论文发展脉络
Chat Paper
正在生成论文摘要