Pre-training Strategies and Datasets for Facial Representation Learning
Computer Vision – ECCV 2022(2022)
摘要
What is the best way to learn a universal face representation? Recent work on Deep Learning in the area of face analysis has focused on supervised learning for specific tasks of interest (e.g. face recognition, facial landmark localization etc.) but has overlooked the overarching question of how to find a facial representation that can be readily adapted to several facial analysis tasks and datasets. To this end, we make the following 4 contributions: (a) we introduce, for the first time, a comprehensive evaluation benchmark for facial representation learning consisting of 5 important face analysis tasks. (b) We systematically investigate two ways of large-scale representation learning applied to faces: supervised and unsupervised pre-training. Importantly, we focus our evaluations on the case of few-shot facial learning. (c) We investigate important properties of the training datasets including their size and quality (labelled, unlabelled or even uncurated). (d) To draw our conclusions, we conducted a very large number of experiments. Our main two findings are: (1) Unsupervised pre-training on completely in-the-wild, uncurated data provides consistent and, in some cases, significant accuracy improvements for all facial tasks considered. (2) Many existing facial video datasets seem to have a large amount of redundancy. We will release code, and pre-trained models to facilitate future research.
更多查看译文
关键词
Face recognition, Face alignment, Emotion recognition, 3D face reconstruction, Representation learning
AI 理解论文
溯源树
样例
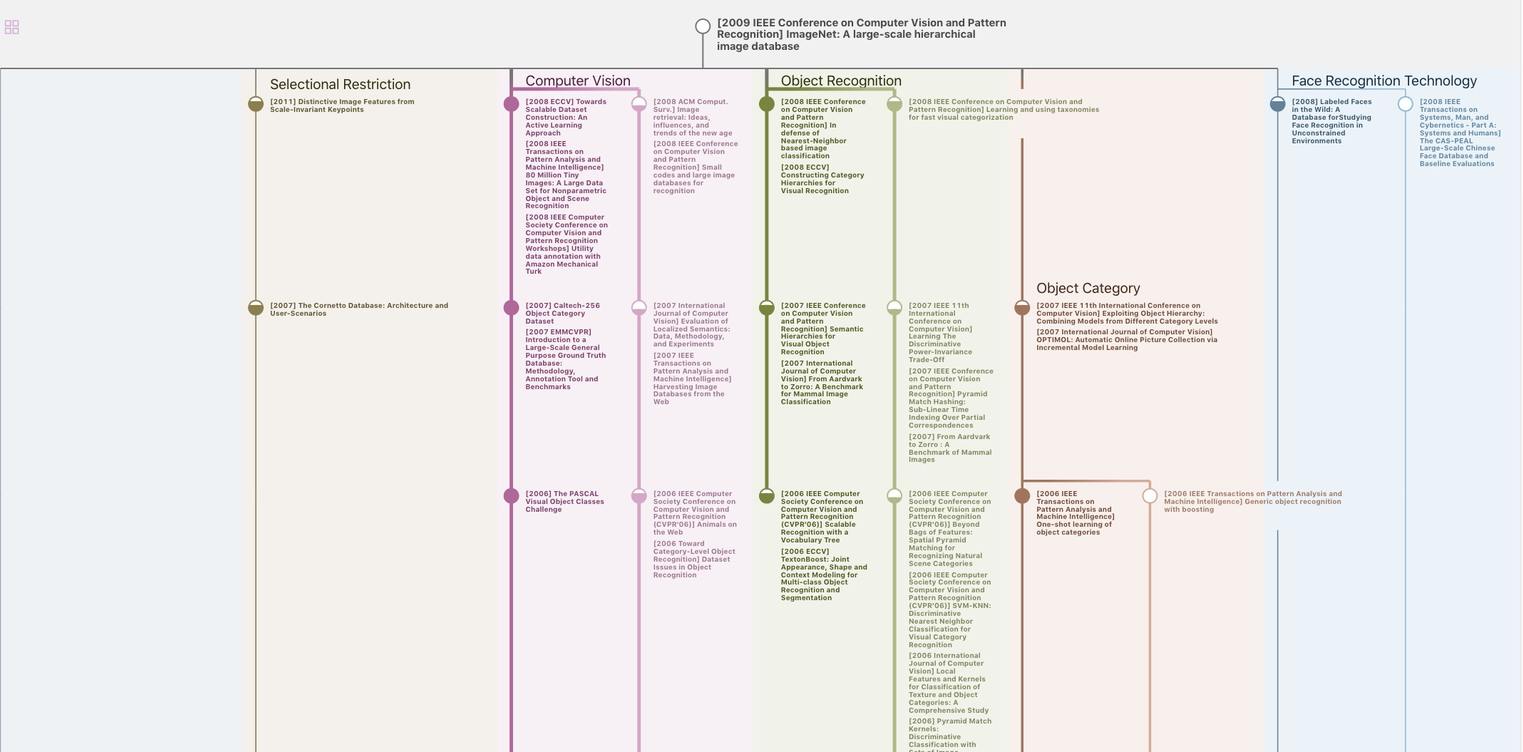
生成溯源树,研究论文发展脉络
Chat Paper
正在生成论文摘要