DR Detection Using Optical Coherence Tomography Angiography (OCTA): A Transfer Learning Approach with Robustness Analysis
OMIA@MICCAI(2020)
摘要
OCTA imaging is an emerging modality for the discovery of retinal biomarkers in systemic disease. Several studies have already shown the potential of deep learning algorithms in the medical domain. However, they generally require large amount of manually graded images which may not always be available. In our study, we aim to investigate whether transfer learning can help in identifying patient status from a relatively small dataset. Additionally, we explore if data augmentation may help in improving our classification accuracy. Finally, for the first time, we propose a validation of our model on OCTA images acquired with a different device. OCTA scans from three different groups of participants were analysed: diabetic with and without retinopathy (DR and NoDR, respectively) and healthy subjects. We used the convolutional neural network architecture VGG16 and achieved \(83.29\%\) accuracy when classifying DR, NoDR and Controls. Our results demonstrate how transfer learning enables fairly accurate OCTA scan classification and augmentation based on geometric transformations helps in improving the classification accuracy further. Finally, we show how our model maintains consistent performance across OCTA imaging devices, without any re-training.
更多查看译文
关键词
Optical coherence tomography angiography,Transfer learning,OCTA devices,Diabetic retinopathy
AI 理解论文
溯源树
样例
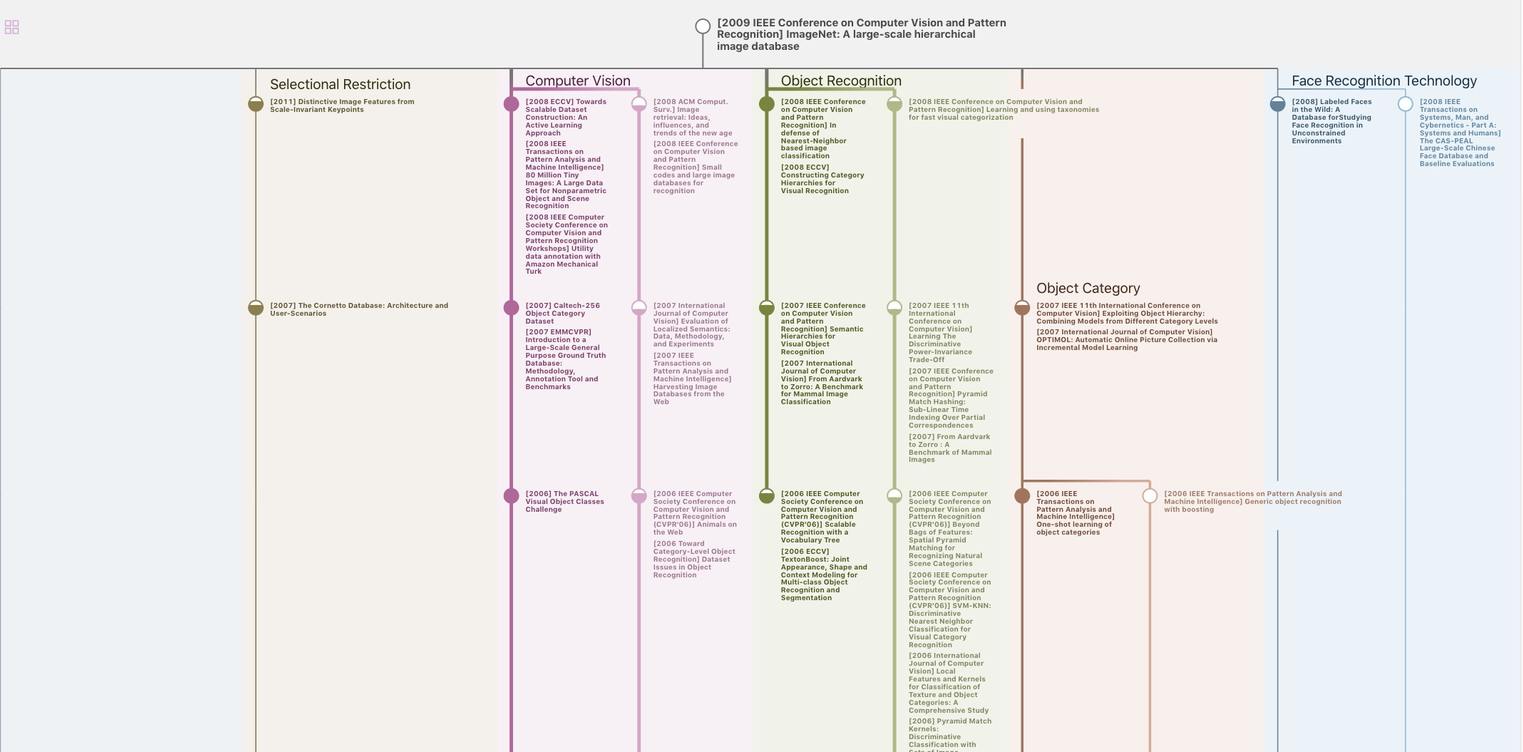
生成溯源树,研究论文发展脉络
Chat Paper
正在生成论文摘要