Deep Learning Approach To Skin Layers Segmentation In Inflammatory Dermatoses
ULTRASONICS(2021)
摘要
Monitoring skin layers with medical imaging is critical to diagnosing and treating patients with chronic inflammatory skin diseases. The high-frequency ultrasound (HFUS) makes it possible to monitor skin condition in different dermatoses. Accurate and reliable segmentation of skin layers in patients with atopic dermatitis or psoriasis enables the assessment of the treatment effect by the layer thickness measurements. The epidermis and the subepidermal low echogenic band (SLEB) are the most important for further diagnosis since their appearance is an indicator of different skin problems. In medical practice, the analysis, including segmentation, is usually performed manually by the physician with all drawbacks of such an approach, e.g., extensive time consumption and lack of repeatability. Recently, HFUS becomes common in dermatological practice, yet it is barely supported by the development of automated analysis tools. To meet the need for skin layer segmentation and measurement, we developed an automated segmentation method of both epidermis and SLEB layers. It consists of a fuzzy cmeans clustering-based preprocessing step followed by a U-shaped convolutional neural network. The network employs batch normalization layers adjusting and scaling the activation to make the segmentation more robust. The obtained segmentation results are verified and compared to the current state-of-the-art methods addressing the skin layer segmentation. The obtained Dice coefficient equal to 0.87 and 0.83 for the epidermis and SLEB, respectively, proves the developed framework?s efficiency, outperforming the other approaches.
更多查看译文
关键词
High frequency ultrasound, Skin layer segmentation, SLEB, Convolutional neural network, SegUNet
AI 理解论文
溯源树
样例
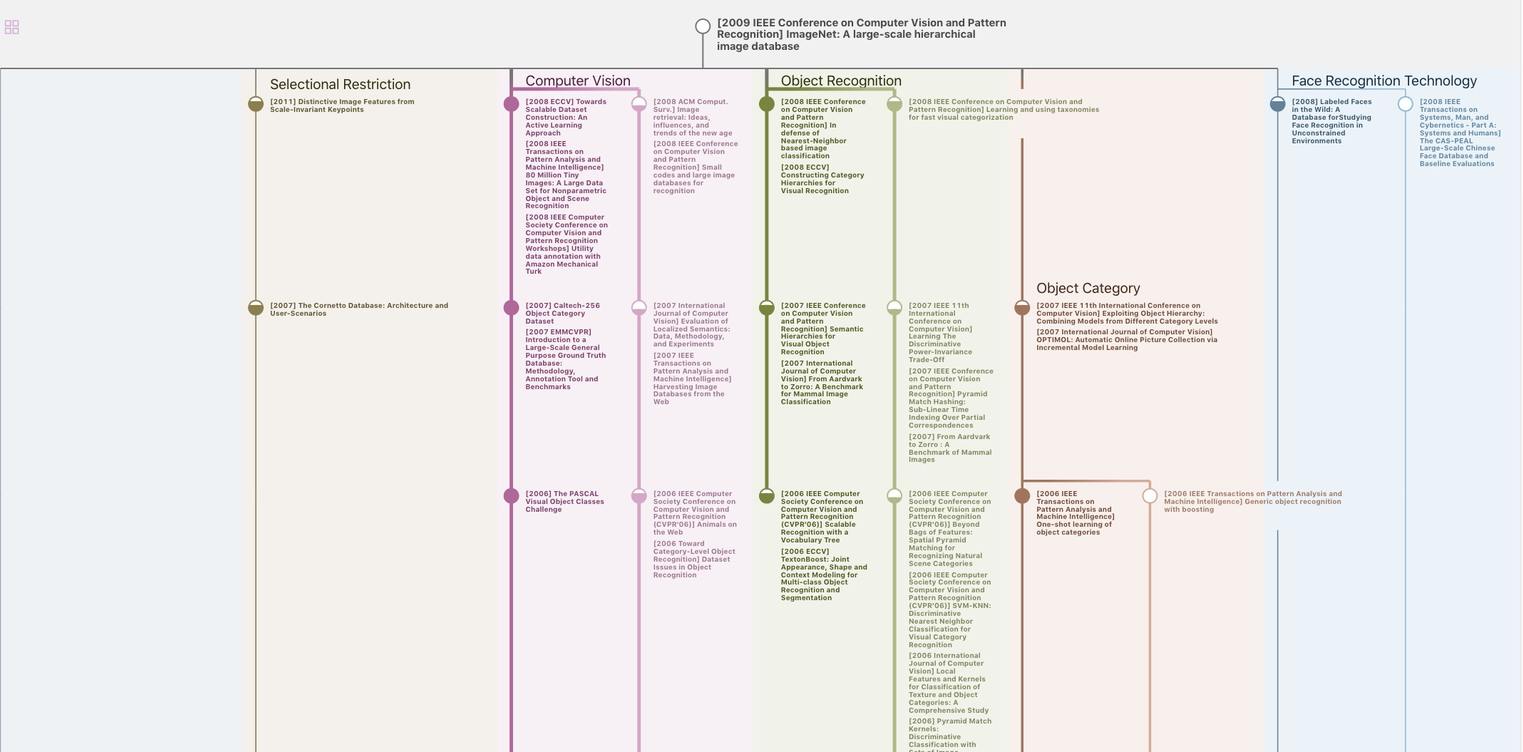
生成溯源树,研究论文发展脉络
Chat Paper
正在生成论文摘要