Linear systems with neural network nonlinearities - Improved stability analysis via acausal Zames-Falb multipliers.
CDC(2021)
摘要
In this paper, we analyze the stability of feedback interconnections of a linear time-invariant system with a neural network nonlinearity in discrete time. Our analysis is based on abstracting neural networks using integral quadratic constraints (IQCs), exploiting the sector-bounded and slope-restricted structure of the underlying activation functions. In contrast to existing approaches, we leverage the full potential of dynamic IQCs to describe the nonlinear activation functions in a less conservative fashion. To be precise, we consider multipliers based on the full-block Yakubovich / circle criterion in combination with acausal Zames-Falb multipliers, leading to linear matrix inequality based stability certificates. Our approach provides a flexible and versatile framework for stability analysis of feedback interconnections with neural network nonlinearities, allowing to trade off computational efficiency and conservatism. Finally, we provide numerical examples that demonstrate the applicability of the proposed framework and the achievable improvements over previous approaches.
更多查看译文
关键词
neural network nonlinearity,acausal Zames-Falb multipliers,feedback interconnections,linear time-invariant system,discrete time,nonlinear activation functions,matrix inequality based stability certificates,full-block Yakubovich-circle criterion
AI 理解论文
溯源树
样例
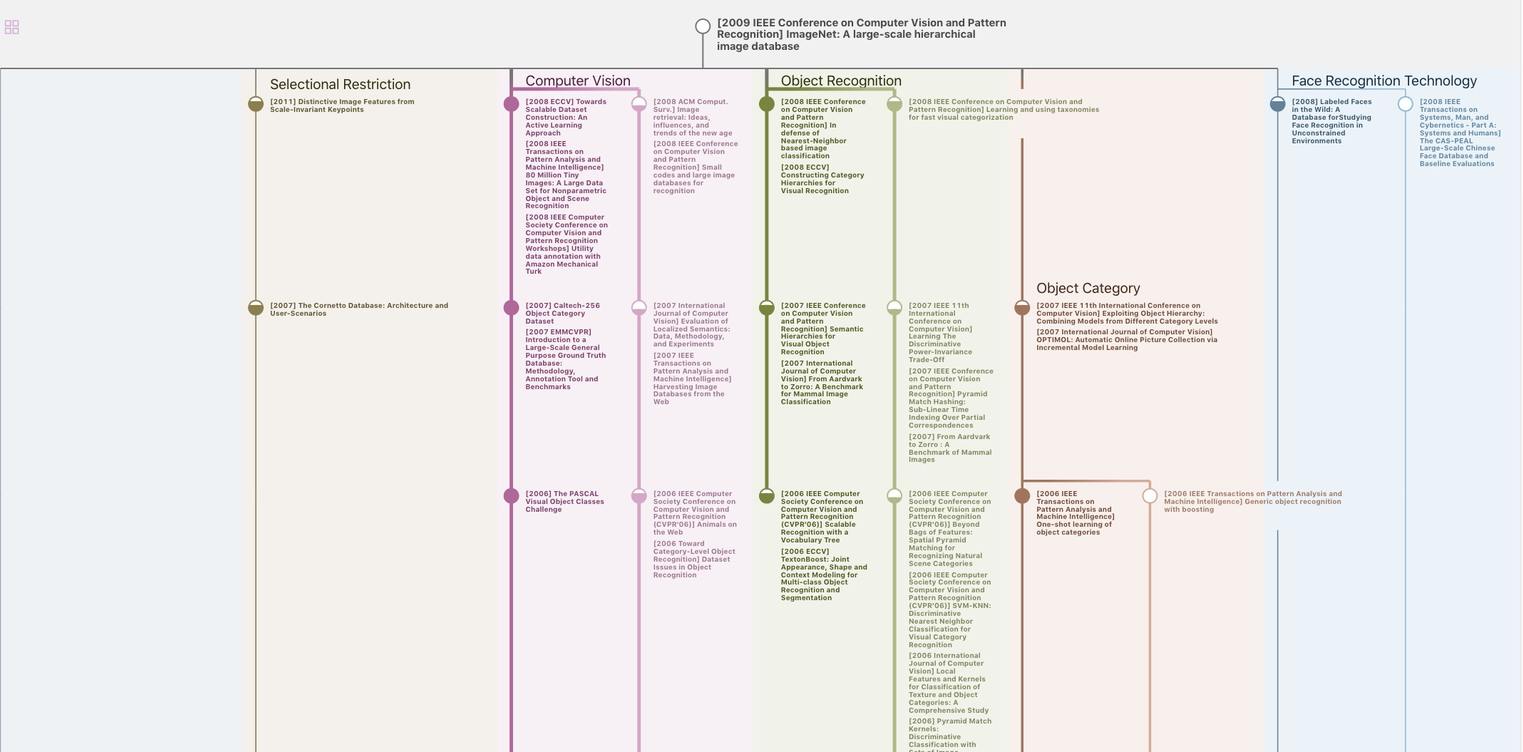
生成溯源树,研究论文发展脉络
Chat Paper
正在生成论文摘要