Neuro-Symbolic Constraint Programming for Structured Prediction
NESY 2021: NEURAL-SYMBOLIC LEARNING AND REASONING(2021)
摘要
We propose Nester, a method for injecting neural networks into constrained structured predictors. Nester first uses a neural network to compute an initial prediction that may or may not satisfy the constraints, and then applies a constraint-based structured predictor to refine the raw predictions according to hard and soft constraints. Nester combines the advantages of its two components: the network can learn complex representations from low-level data while the constraint program on top reasons about the high-level properties and requirements of the prediction task. An empirical evaluation on handwritten equation recognition shows that Nester achieves better performance than both the either component in isolation, especially when training examples are scarce, while scaling to more complex problems than other neuro-programming approaches. Nester proves especially useful to reduce errors at the semantic level of the problem, which is particularly challenging for neural network architectures.
更多查看译文
关键词
Machine Learning, Neuro-Symbolic Integration, Structured Prediction, Constraint Programming
AI 理解论文
溯源树
样例
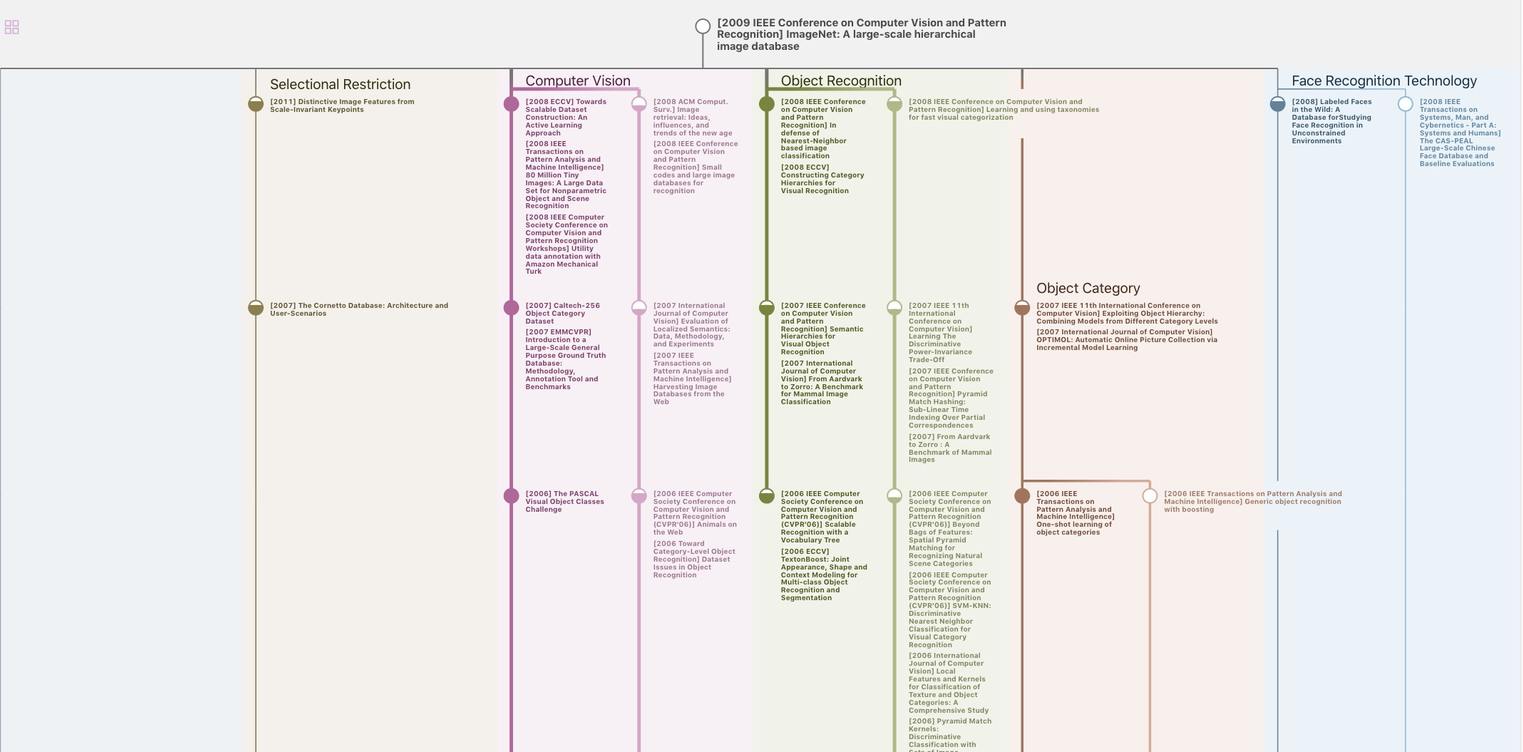
生成溯源树,研究论文发展脉络
Chat Paper
正在生成论文摘要