SST-GNN: Simplified Spatio-Temporal Traffic Forecasting Model Using Graph Neural Network
ADVANCES IN KNOWLEDGE DISCOVERY AND DATA MINING, PAKDD 2021, PT III(2021)
摘要
To capture spatial relationships and temporal dynamics in traffic data, spatio-temporal models for traffic forecasting have drawn significant attention in recent years. Most of the recent works employed graph neural networks(GNN) with multiple layers to capture the spatial dependency. However, road junctions with different hop-distance can carry distinct traffic information which should be exploited separately but existing multi-layer GNNs are incompetent to discriminate between their impact. Again, to capture the temporal interrelationship, recurrent neural networks are common in state-of-the-art approaches that often fail to capture long-range dependencies. Furthermore, traffic data shows repeated patterns in a daily or weekly period which should be addressed explicitly. To address these limitations, we have designed a Simplified Spatio-temporal Traffic forecasting GNN(SST-GNN) that effectively encodes the spatial dependency by separately aggregating different neighborhood representations rather than with multiple layers and capture the temporal dependency with a simple yet effective weighted spatio-temporal aggregation mechanism. We capture the periodic traffic patterns by using a novel position encoding scheme with historical and current data in two different models. With extensive experimental analysis, we have shown that our model (Code is available at github.com/AmitRoy7781/SST-GNN) has significantly outperformed the state-of-the-art models on three real-world traffic datasets from the Performance Measurement System (PeMS).
更多查看译文
关键词
Traffic forecasting,Spatio-temporal modeling,Graph neural network
AI 理解论文
溯源树
样例
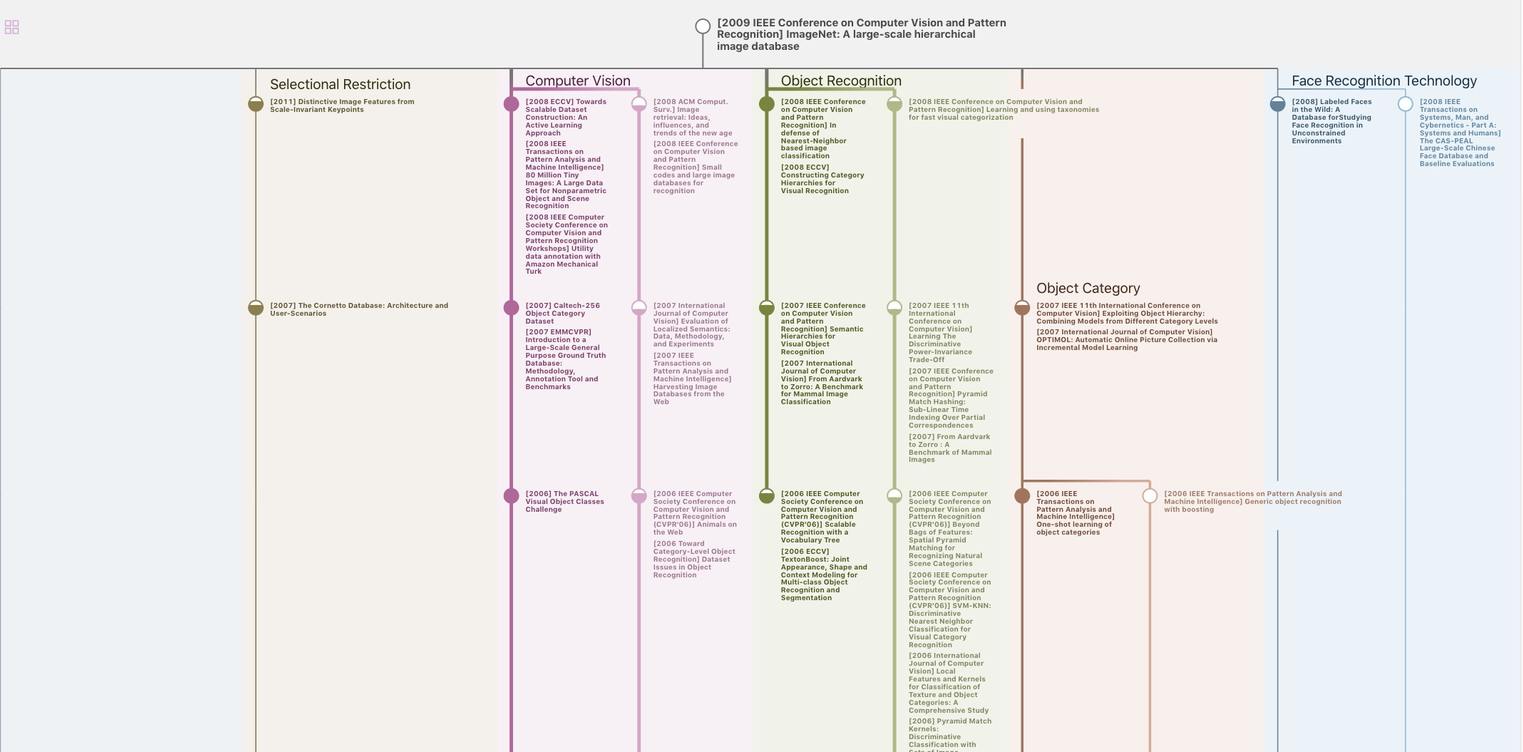
生成溯源树,研究论文发展脉络
Chat Paper
正在生成论文摘要