A Contributor-Focused Intrinsic Quality Assessment Of Openstreetmap In Mozambique Using Unsupervised Machine Learning
ISPRS INTERNATIONAL JOURNAL OF GEO-INFORMATION(2021)
摘要
Anyone can contribute geographic information to OpenStreetMap (OSM), regardless of their level of experience or skills, which has raised concerns about quality. When reference data is not available to assess the quality of OSM data, intrinsic methods that assess the data and its metadata can be used. In this study, we applied unsupervised machine learning for analysing OSM history data to get a better understanding of who contributed when and how in Mozambique. Even though no absolute statements can be made about the quality of the data, the results provide valuable insight into the quality. Most of the data in Mozambique (93%) was contributed by a small group of active contributors (25%). However, these were less active than the OSM Foundation's definition of active contributorship and the Humanitarian OpenStreetMap Team (HOT) definition for intermediate mappers. Compared to other contributor classifications, our results revealed a new class: contributors who were new in the area and most likely attracted by HOT mapping events during disaster relief operations in Mozambique in 2019. More studies in different parts of the world would establish whether the patterns observed here are typical for developing countries. Intrinsic methods cannot replace ground truthing or extrinsic methods, but provide alternative ways for gaining insight about quality, and they can also be used to inform efforts to further improve the quality. We provide suggestions for how contributor-focused intrinsic quality assessments could be further refined.
更多查看译文
关键词
volunteered geographic information, crowdsourcing, data quality, intrinsic quality assessment, contributors, OpenStreetMap, Mozambique
AI 理解论文
溯源树
样例
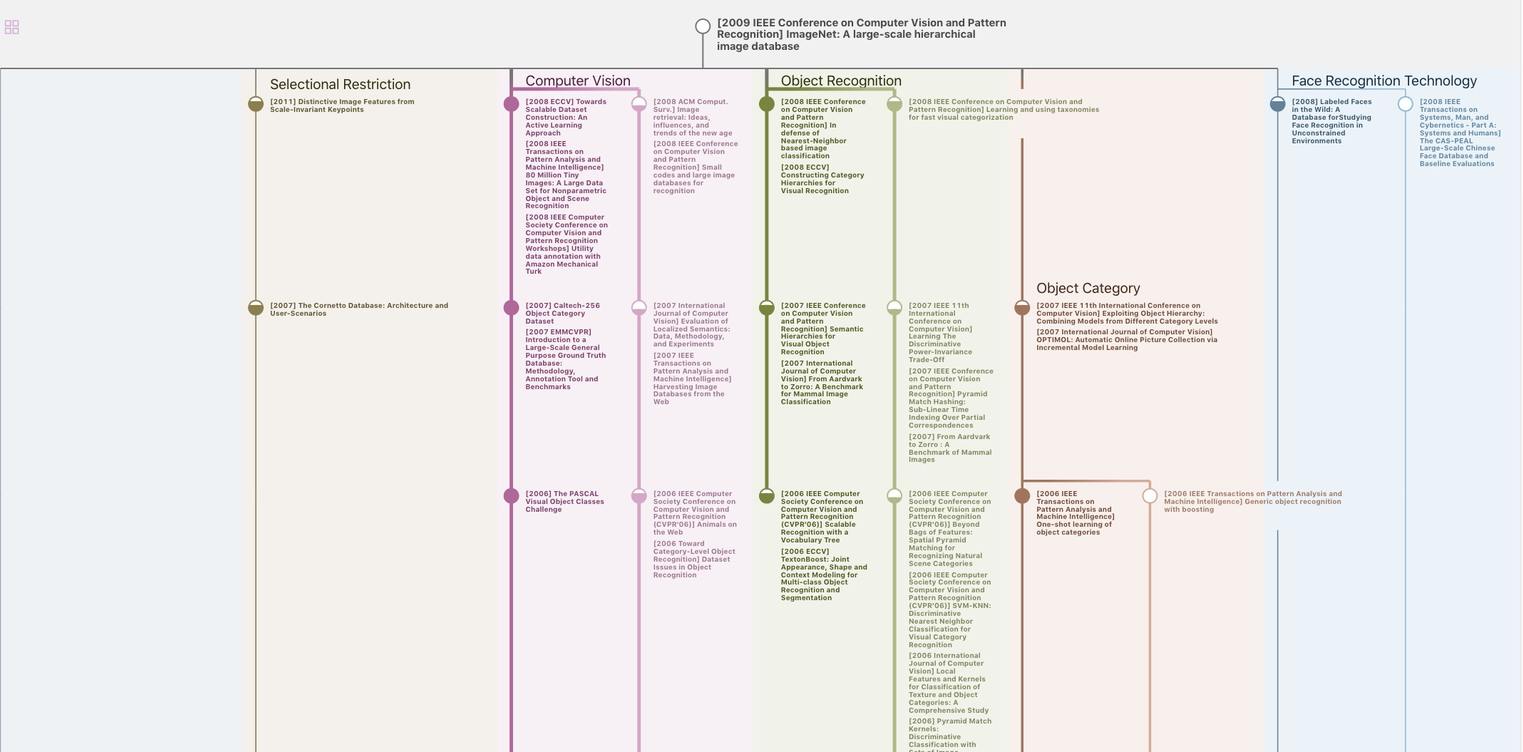
生成溯源树,研究论文发展脉络
Chat Paper
正在生成论文摘要