Machine learning approaches to constructing predictive models of vitamin D deficiency in a hypertensive population: a comparative study
INFORMATICS FOR HEALTH & SOCIAL CARE(2021)
摘要
Objective: Given the association between vitamin D deficiency and risk for cardiovascular disease, we used machine learning approaches to establish a model to predict the probability of deficiency. Determination of serum levels of 25-hydroxy vitamin D (25(OH)D) provided the best assessment of vitamin D status, but such tests are not always widely available or feasible. Thus, our study established predictive models with high sensitivity to identify patients either unlikely to have vitamin D deficiency or who should undergo 25(OH)D testing. Methods: We collected data from 1002 hypertensive patients from a Spanish university hospital. The elastic net regularization approach was applied to reduce the dimensionality of the dataset. The issue of determining vitamin D status was addressed as a classification problem; thus, the following classifiers were applied: logistic regression, support vector machine (SVM), random forest, naive Bayes, and Extreme Gradient Boost methods. Classification accuracy, sensitivity, specificity, and predictive values were computed to assess the performance of each method. Results: The SVM-based method with radial kernel performed better than the other algorithms in terms of sensitivity (98%), negative predictive value (71%), and classification accuracy (73%). Conclusion: The combination of a feature-selection method such as elastic net regularization and a classification approach produced well-fitted models. The SVM approach yielded better predictions than the other algorithms. This combination approach allowed us to develop a predictive model with high sensitivity but low specificity, to identify the population that could benefit from laboratory determination of serum levels of 25(OH)D.
更多查看译文
关键词
Vitamin D deficiency, cardiovascular risk assessment, predictive model, logistic regression, support vector machine, random forest, naive Bayes
AI 理解论文
溯源树
样例
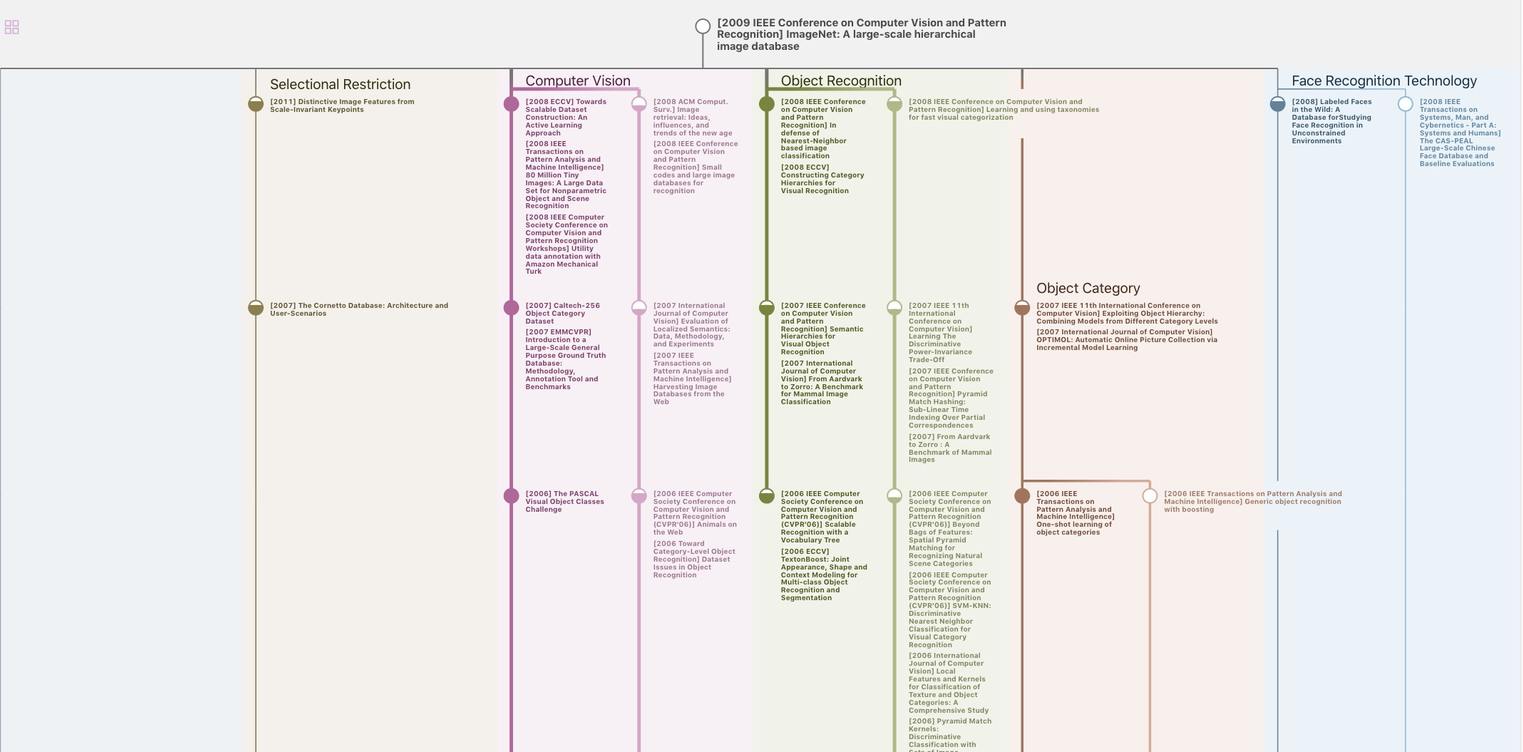
生成溯源树,研究论文发展脉络
Chat Paper
正在生成论文摘要