FeTaQA: Free-form Table Question Answering
Transactions of the Association for Computational Linguistics(2022)
摘要
Existing table question answering datasets contain abundant factual questions that primarily evaluate the query and schema comprehension capability of a system, but they fail to include questions that require complex reasoning and integration of information due to the constraint of the associated short-form answers. To address these issues and to demonstrate the full challenge of table question answering, we introduce FeTaQA, a new dataset with 10K Wikipedia-based {table, question, free-form answer, supporting table cells} pairs. FeTaQA yields a more challenging table question answering setting because it requires generating free-form text answers after retrieval, inference, and integration of multiple discontinuous facts from a structured knowledge source. Unlike datasets of generative QA over text in which answers are prevalent with copies of short text spans from the source, answers in our dataset are human-generated explanations involving entities and their high-level relations. We provide two benchmark methods for the proposed task: a pipeline method based on semantic-parsing-based QA systems and an end-to-end method based on large pretrained text generation models, and show that FeTaQA poses a challenge for both methods.
更多查看译文
关键词
table,fetaqa,free-form
AI 理解论文
溯源树
样例
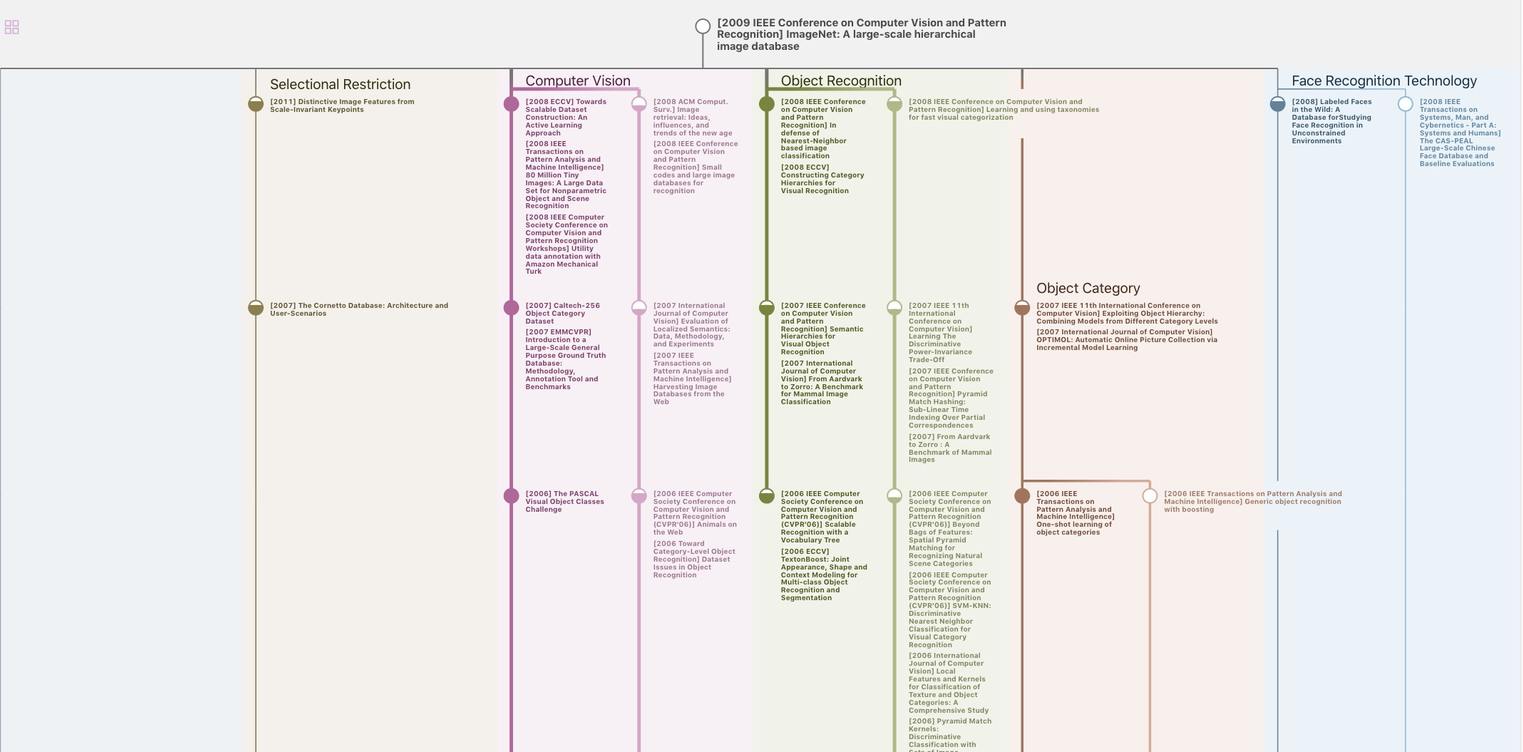
生成溯源树,研究论文发展脉络
Chat Paper
正在生成论文摘要