Automated Identification Of Glomeruli And Synchronised Review Of Special Stains In Renal Biopsies By Machine Learning And Slide Registration: A Cross-Institutional Study
HISTOPATHOLOGY(2021)
摘要
Aims Machine learning in digital pathology can improve efficiency and accuracy via prescreening with automated feature identification. Studies using uniform histological material have shown promise. Generalised application requires validation on slides from multiple institutions. We used machine learning to identify glomeruli on renal biopsies and compared performance between single and multiple institutions. Methods and results Randomly selected, adequately sampled renal core biopsy cases (71) consisting of four stains each (haematoxylin and eosin, trichrome, silver, periodic acid Schiff) from three institutions were digitised at x40. Glomeruli were manually annotated by three renal pathologists using a digital tool. Cases were divided into training/validation (n = 52) and evaluation (n = 19) cohorts. An algorithm was trained to develop three convolutional neural network (CNN) models which tested case cohorts intra- and inter-institutionally. Raw CNN search data from each of the four slides per case were merged into composite regions of interest containing putative glomeruli. The sensitivity and modified specificity of glomerulus detection (versus annotated truth) were calculated for each model/cohort. Intra-institutional (3) sensitivity ranged from 90 to 93%, with modified specificity from 86 to 98%. Interinstitutional (1) sensitivity was 77%, with modified specificity 97%. Combined intra- and inter-institutional (1) sensitivity was 86%, with modified specificity 92%. Conclusions Feature detection sensitivity degrades when training and test material originate from different sites. Training using a combined set of digital slides from three institutions improves performance. Differing histology methods probably account for algorithm performance contrasts. Our data highlight the need for diverse training sets for the development of generalisable machine learning histology algorithms.
更多查看译文
关键词
glomerulus, image registration, machine learning, renal pathology, whole slide imaging
AI 理解论文
溯源树
样例
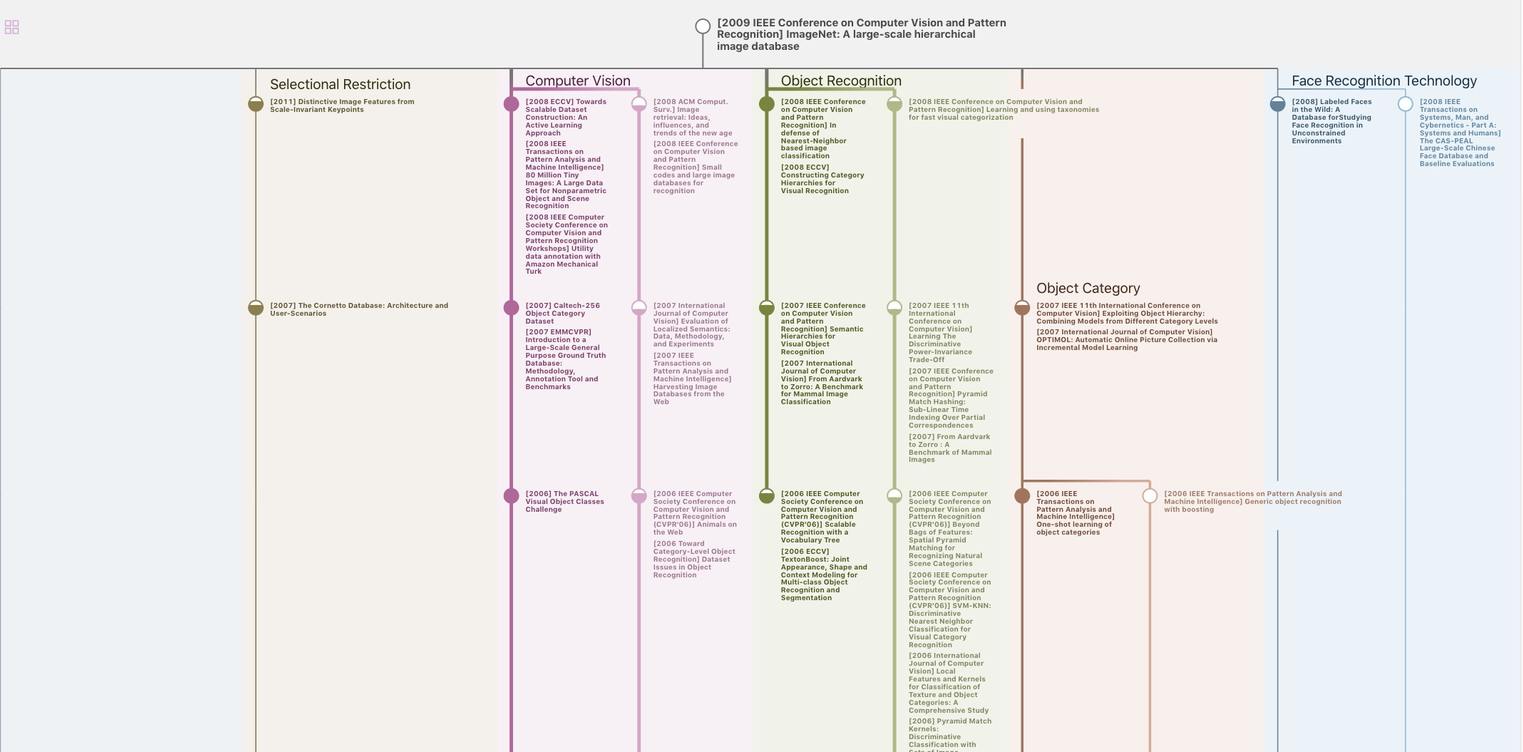
生成溯源树,研究论文发展脉络
Chat Paper
正在生成论文摘要