A Comparative Study of CNN Transfer Learning Classification Algorithms with Segmentation for COVID-19 Detection from CT Scan Images
PROCEEDINGS OF 2020 11TH INTERNATIONAL CONFERENCE ON ELECTRICAL AND COMPUTER ENGINEERING (ICECE)(2020)
摘要
After it's inception, COVID-19 has spread rapidly all across the globe. Considering this outbreak, by far, it is the most decisive task to detect early and isolate the patients quickly to contain the spread of this virus. In such cases, artificial intelligence and machine learning or deep learning methods can come to aid. For that purpose, we have conducted a qualitative investigation to inspect 12 off-the-shelf Convolution Neural Network (CNN) architectures in classifying COVID-19 from CT scan images. Furthermore, a segmentation algorithm for biomedical images - U-Net, is analyzed to evaluate the performance of the CNN models. A publicly available dataset (SARS-COV-2 CT-Scan) containing a total of 2481 CT scan images is employed for the performance evaluation. In terms of feature extraction by excluding the segmentation technique, a performance of 88.60% as the F1 Score and 89.31% as accuracy is achieved by training DenseNet169 architecture. Adopting the U-Net segmentation method, we accomplished the most optimal accuracy and F1 Scores as 89.92% and 89.67% respectively on DenseNet201 model. Furthermore, evaluating the performances, we can affirm that a combination of a Transfer Learning architecture with a segmentation technique (U-Net) enhances the performance of the classification model.
更多查看译文
关键词
COVID-19,Transfer learning,CNN,CT scan,DenseNet,U-Net
AI 理解论文
溯源树
样例
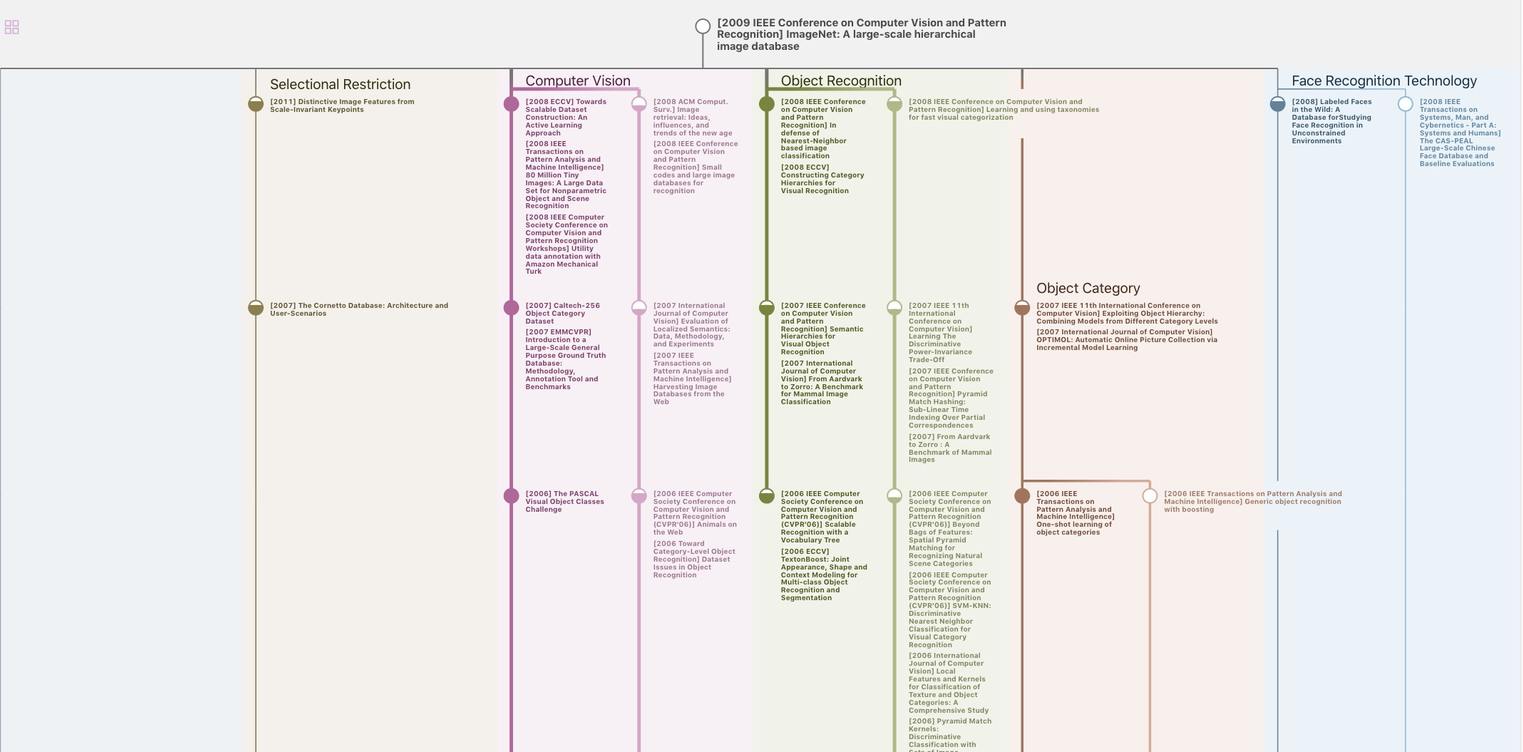
生成溯源树,研究论文发展脉络
Chat Paper
正在生成论文摘要