Instance Level Affinity-Based Transfer for Unsupervised Domain Adaptation
2021 IEEE/CVF CONFERENCE ON COMPUTER VISION AND PATTERN RECOGNITION, CVPR 2021(2021)
摘要
Domain adaptation deals with training models using large scale labeled data from a specific source domain and then adapting the knowledge to certain target domains that have few or no labels. Many prior works learn domain agnostic feature representations for this purpose using a global distribution alignment objective which does not take into account the finer class specific structure in the source and target domains. We address this issue in our work and propose an instance affinity based criterion for source to target transfer during adaptation, called ILA-DA. We first propose a reliable and efficient method to extract similar and dissimilar samples across source and target, and utilize a multi-sample contrastive loss to drive the domain alignment process. ILA-DA simultaneously accounts for intra-class clustering as well as inter-class separation among the categories, resulting in less noisy classifier boundaries, improved transferability and increased accuracy. We verify the effectiveness of ILA-DA by observing consistent improvements in accuracy over popular domain adaptation approaches on a variety of benchmark datasets and provide insights into the proposed alignment approach. Code will be made publicly available at https://github.com/astuti/ILA-DA.
更多查看译文
关键词
instance level affinity-based transfer,unsupervised domain adaptation,domain adaptation deals,training models,specific source domain,target domains,domain agnostic feature representations,global distribution alignment objective,finer class specific structure,instance affinity,called ILA-DA,similar samples,dissimilar samples,multisample contrastive loss,domain alignment process,intra-class clustering,inter-class separation,improved transferability,popular domain adaptation approaches
AI 理解论文
溯源树
样例
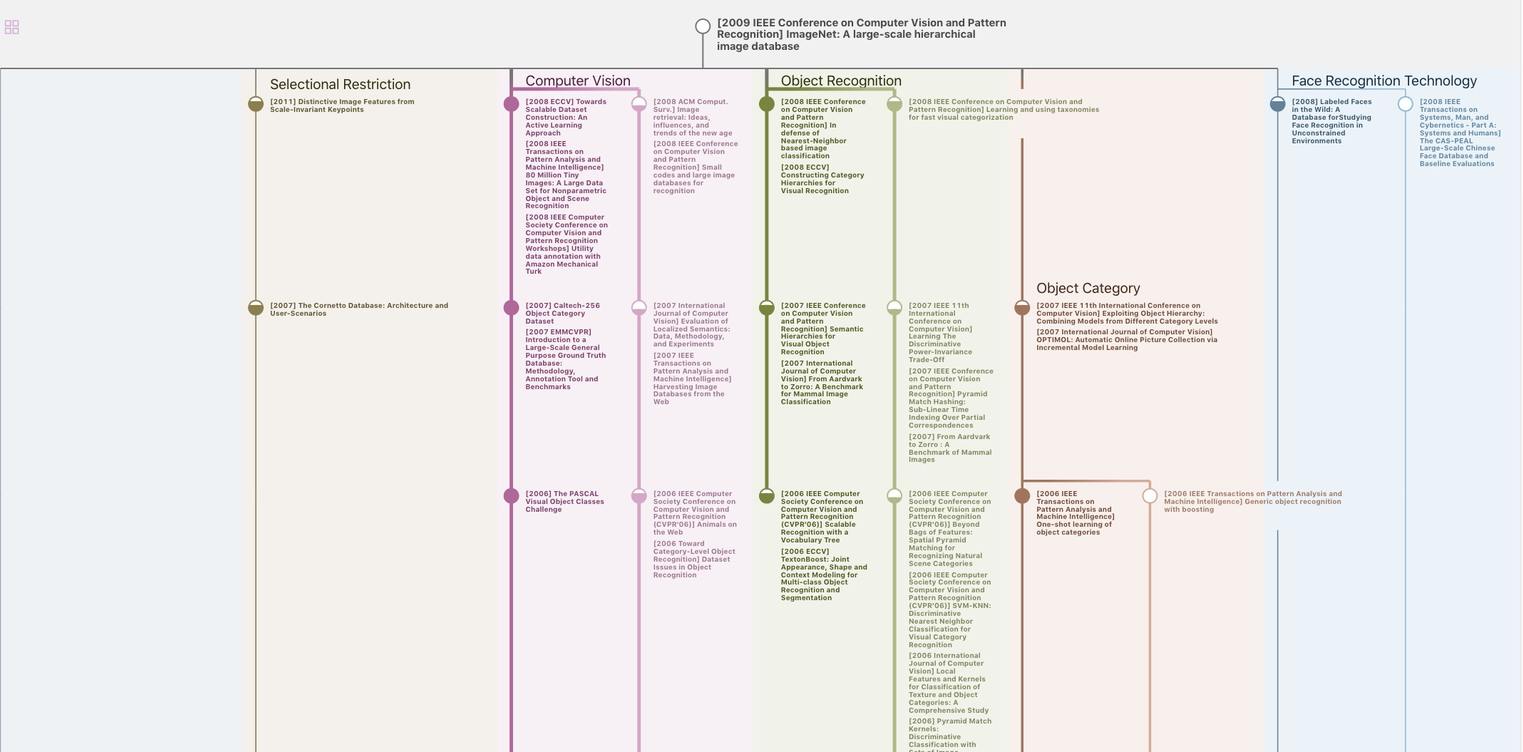
生成溯源树,研究论文发展脉络
Chat Paper
正在生成论文摘要