GSECnet: Ground Segmentation of Point Clouds for Edge Computing
arXiv (Cornell University)(2021)
摘要
Ground segmentation of point clouds remains challenging because of the sparse and unordered data structure. This paper proposes the GSECnet - Ground Segmentation network for Edge Computing, an efficient ground segmentation framework of point clouds specifically designed to be deployable on a low-power edge computing unit. First, raw point clouds are converted into a discretization representation by pillarization. Afterward, features of points within pillars are fed into PointNet to get the corresponding pillars feature map. Then, a depthwise-separable U-Net with the attention module learns the classification from the pillars feature map with an enormously diminished model parameter size. Our proposed framework is evaluated on SemanticKITTI against both point-based and discretization-based state-of-the-art learning approaches, and achieves an excellent balance between high accuracy and low computing complexity. Remarkably, our framework achieves the inference runtime of 135.2 Hz on a desktop platform. Moreover, experiments verify that it is deployable on a low-power edge computing unit powered 10 watts only.
更多查看译文
关键词
edge computing,ground segmentation,point clouds
AI 理解论文
溯源树
样例
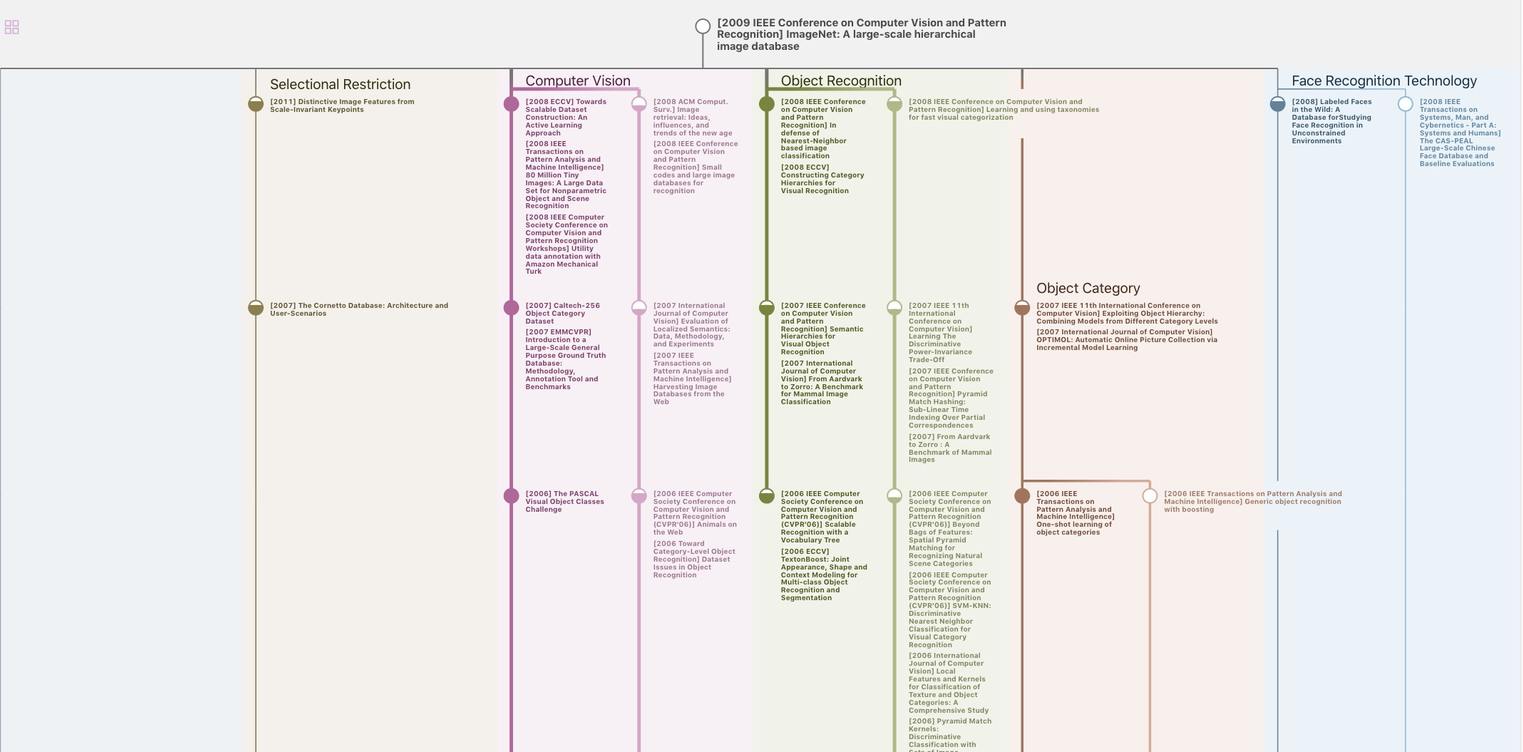
生成溯源树,研究论文发展脉络
Chat Paper
正在生成论文摘要