IronMask: Modular Architecture for Protecting Deep Face Template
2021 IEEE/CVF CONFERENCE ON COMPUTER VISION AND PATTERN RECOGNITION, CVPR 2021(2021)
摘要
Convolutional neural networks have made remarkable progress in the face recognition field. The more the technology of face recognition advances, the greater discriminative features into a face template. However, this increases the threat to user privacy in case the template is exposed. In this paper, we present a modular architecture for face template protection, called IronMask, that can be combined with any face recognition system using angular distance metric. We circumvent the need for binarization, which is the main cause of performance degradation in most existing face template protections, by proposing a new real-valued error-correcting-code that is compatible with real-valued templates and can therefore, minimize performance degradation. We evaluate the efficacy of IronMask by extensive experiments on two face recognitions, ArcFace and Cos-Face with three datasets, CMU-Multi-PIE, FEI, and Color-FERET. According to our experimental results, IronMask achieves a true accept rate (TAR) of 99.79% at a false accept rate (FAR) of 0.0005% when combined with ArcFace, and 95.78% TAR at 0% FAR with CosFace, while providing at least 115-bit security against known attacks.
更多查看译文
关键词
modular architecture,convolutional neural networks,face recognition field,discriminative features,user privacy,face template protection,face recognition system,angular distance metric,performance degradation,real-valued error-correcting-code,real-valued templates,IronMask,deep face template,CMU-Multi-PIE,FEI,Color-FERET,true accept rate,TAR,ArcFace,false accept rate,FAR,CosFace
AI 理解论文
溯源树
样例
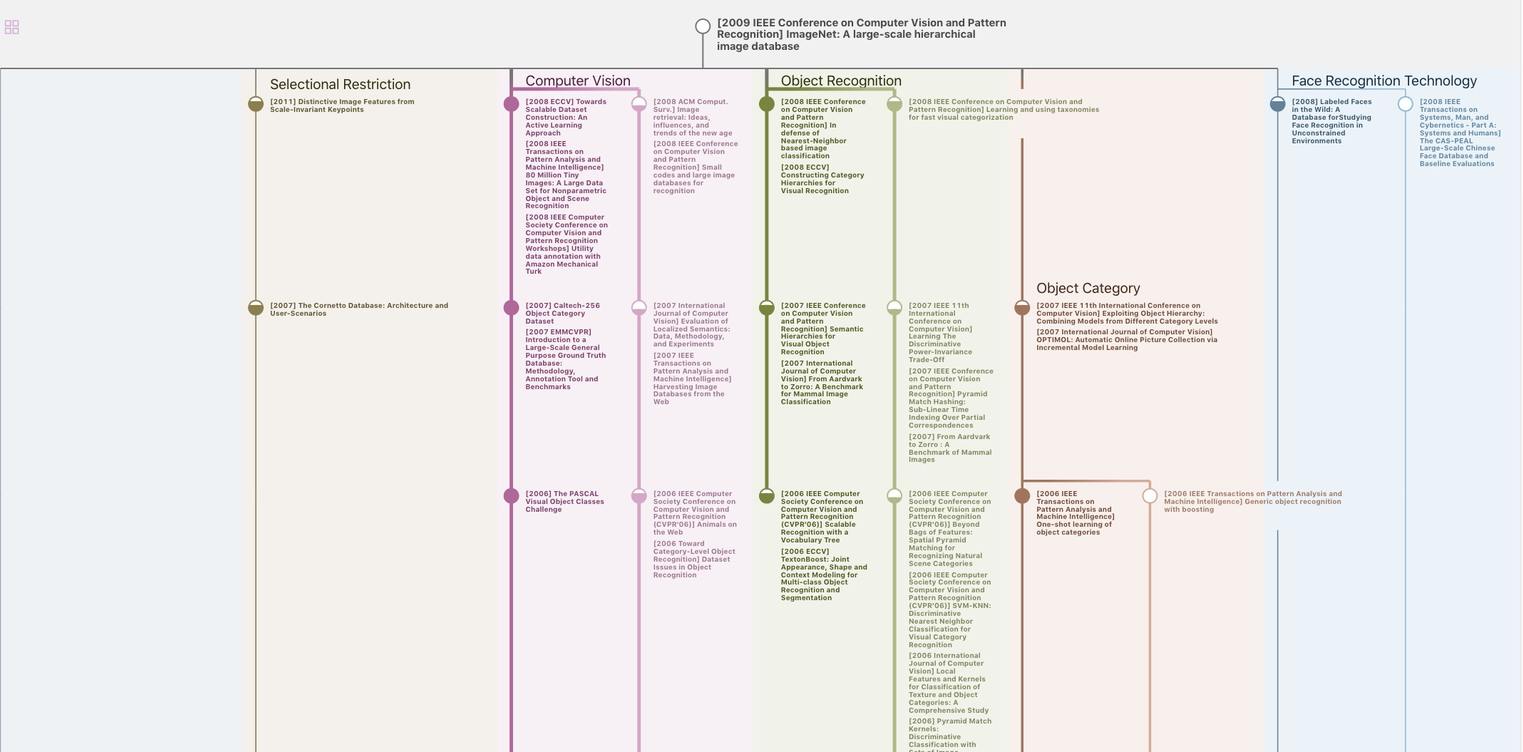
生成溯源树,研究论文发展脉络
Chat Paper
正在生成论文摘要