ODE Transformer: An Ordinary Differential Equation-Inspired Model for Neural Machine Translation
arXiv (Cornell University)(2021)
摘要
It has been found that residual networks are an Euler discretization of solutions to Ordinary Differential Equations (ODEs). In this paper, we explore a deeper relationship between Transformer and numerical methods of ODEs. We show that a residual block of layers in Transformer can be described as a higher-order solution to ODEs. This leads us to design a new architecture (call it ODE Transformer) analogous to the Runge-Kutta method that is well motivated in ODEs. As a natural extension to Transformer, ODE Transformer is easy to implement and parameter efficient. Our experiments on three WMT tasks demonstrate the genericity of this model, and large improvements in performance over several strong baselines. It achieves 30.76 and 44.11 BLEU scores on the WMT'14 En-De and En-Fr test data. This sets a new state-of-the-art on the WMT'14 En-Fr task.
更多查看译文
关键词
neural machine translation,transformer,equation-inspired
AI 理解论文
溯源树
样例
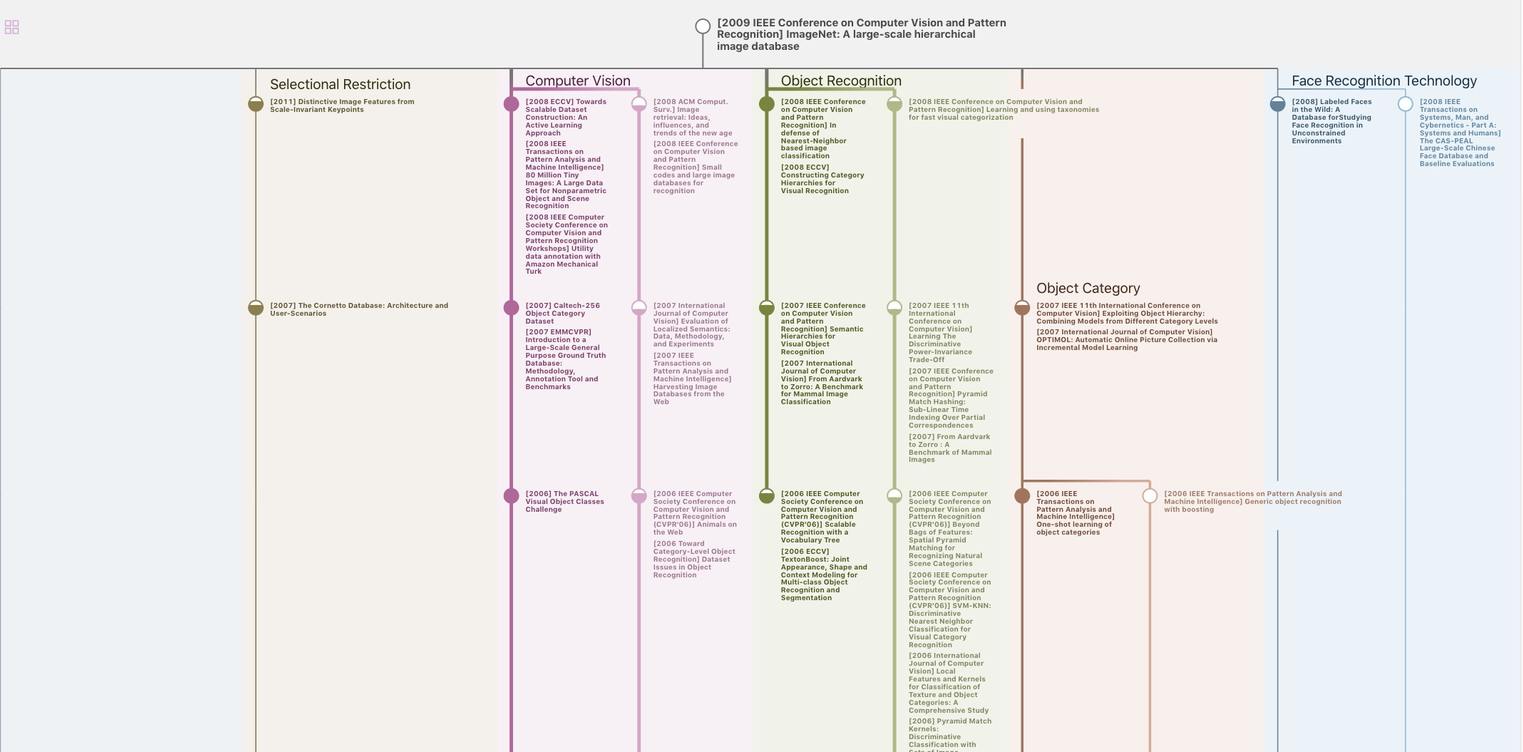
生成溯源树,研究论文发展脉络
Chat Paper
正在生成论文摘要