Multimodal Object Detection via Bayesian Fusion.
CoRR(2021)
摘要
Object detection with multimodal inputs can improve many safety-critical perception systems such as autonomous vehicles (AVs). Motivated by AVs that operate in both day and night, we study multimodal object detection with RGB and thermal cameras, since the latter can provide much stronger object signatures under poor illumination. We explore strategies for fusing information from different modalities. Our key contribution is a non-learned late-fusion method that fuses together bounding box detections from different modalities via a simple probabilistic model derived from first principles. Our simple approach, which we call Bayesian Fusion, is readily derived from conditional independence assumptions across different modalities. We apply our approach to benchmarks containing both aligned (KAIST) and unaligned (FLIR) multimodal sensor data. Our Bayesian Fusion outperforms prior work by more than 13% in relative performance.
更多查看译文
关键词
bayesian fusion,object detection
AI 理解论文
溯源树
样例
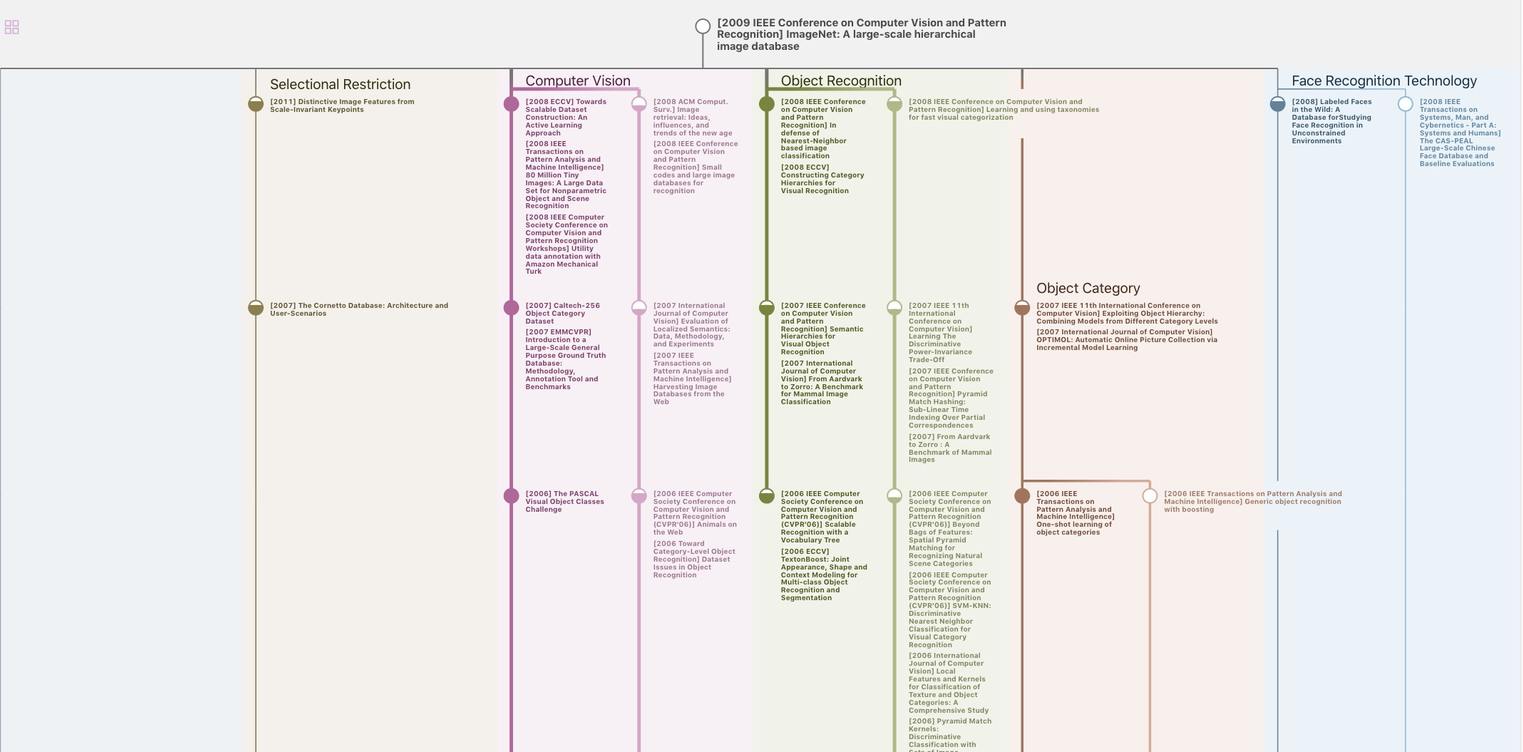
生成溯源树,研究论文发展脉络
Chat Paper
正在生成论文摘要