Beyond Impute-Then-Regress: Adapting Prediction to Missing Data
arXiv (Cornell University)(2022)
Abstract
Missing values are a common issue in real-world datasets. The gold standard for dealing with missing data in inference is to assume that the data is missing at random and apply an impute-then-estimate procedure. In this paper, we evaluate the relevance of the assumptions and methods developed in inference for prediction tasks. We first} provide a theoretical analysis of impute-then-regress methods and highlight their successes and failures in making accurate predictions. We propose adaptive linear regression, a new class of models that adapt to the set of available features and can be applied on partially observed data directly. We show that adaptive linear regression can be equivalent to impute-then-regress methods where the imputation and the linear regression models are learned simultaneously instead of sequentially. We leverage this joint-impute-then-regress interpretation to generalize our framework to non-linear models. We validate our theoretical findings and adaptive regression approaches with extensive numerical results on synthetic, semi-synthetic, and real-world datasets. Among others, in settings where data is strongly not missing at random, our methods achieve a 6\% improvement in out-of-sample accuracy.
MoreTranslated text
Key words
prediction,data,impute-then-regress
AI Read Science
Must-Reading Tree
Example
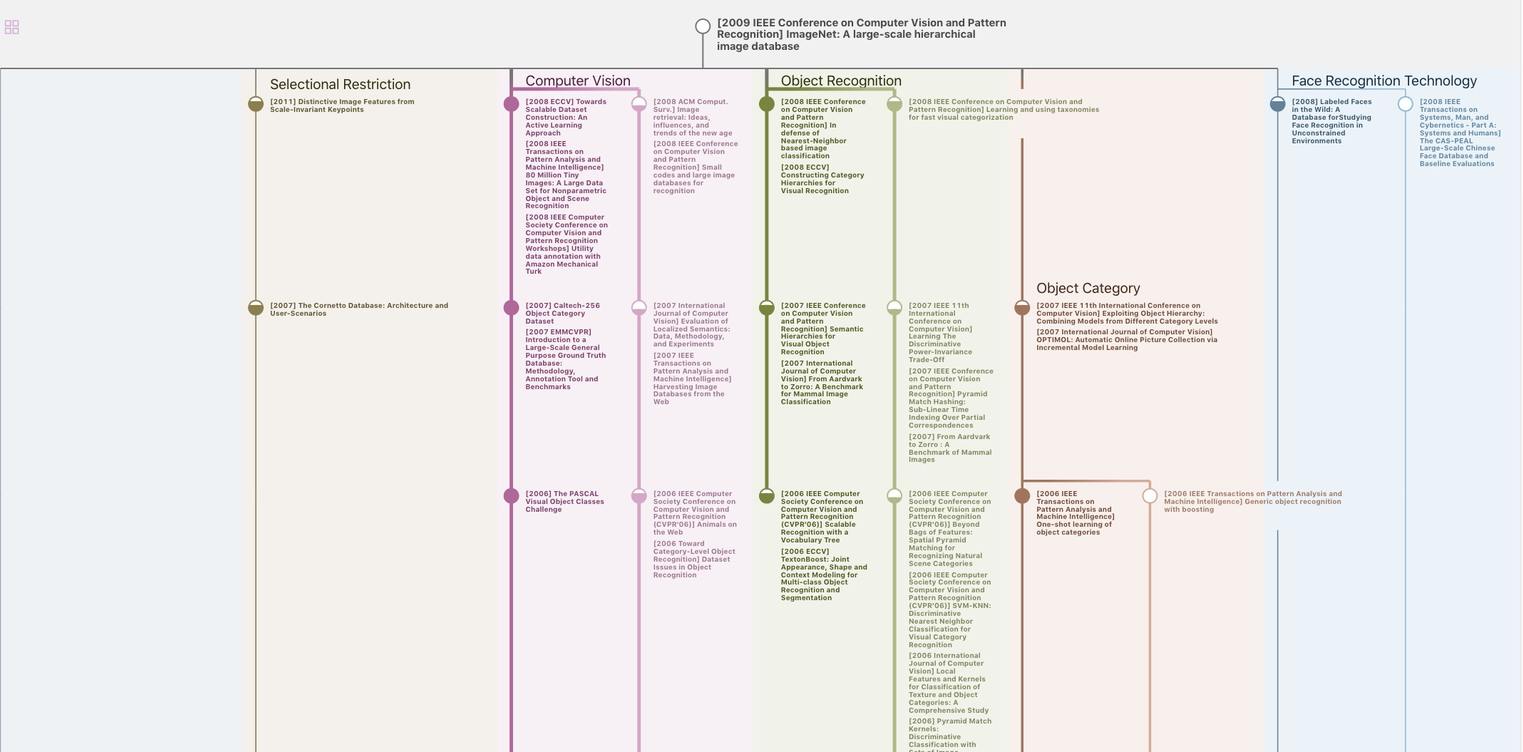
Generate MRT to find the research sequence of this paper
Chat Paper
Summary is being generated by the instructions you defined