A Pre-training Approach for Stance Classification in Online Forums
2020 IEEE/ACM International Conference on Advances in Social Networks Analysis and Mining (ASONAM)(2020)
摘要
Stance detection is the task of automatically determining whether the author of a piece of text is in favor of, against, or neutral towards a target such as a topic, entity, or claim. In this paper, we propose a method based on RoBERTa to classify stances by capturing the context of the discussion through the examination of pairs of stances and relational structures of debates specific to each topic within the defined window of each forum participant's interventions. Furthermore, we examine the degree of disagreement and neutrality in various debate topics to measure divergence of opinion in the course of the debate and estimate the emotional state manifested in different debate topics. We conduct extensive experiments using two publicly available datasets and demonstrate that our method considers more stance classes, provides better results and yields statistical improvements over existing techniques. Our quantitative analysis of model performance yields F-1 scores of over 0.745. Interestingly, we obtained the highest F-1 score, 0.814, on a stance class which was not taken into consideration in prior work. We report that none of the metrics utilized to measure divergence of opinion yield values exceeding 50 % and the correlations between the same topics over 10-fold cross-validation are statistically significant for the majority of them (p <; 0.005). Several future research avenues are proposed.
更多查看译文
关键词
opinion,sentence-pair,divergence of opinion
AI 理解论文
溯源树
样例
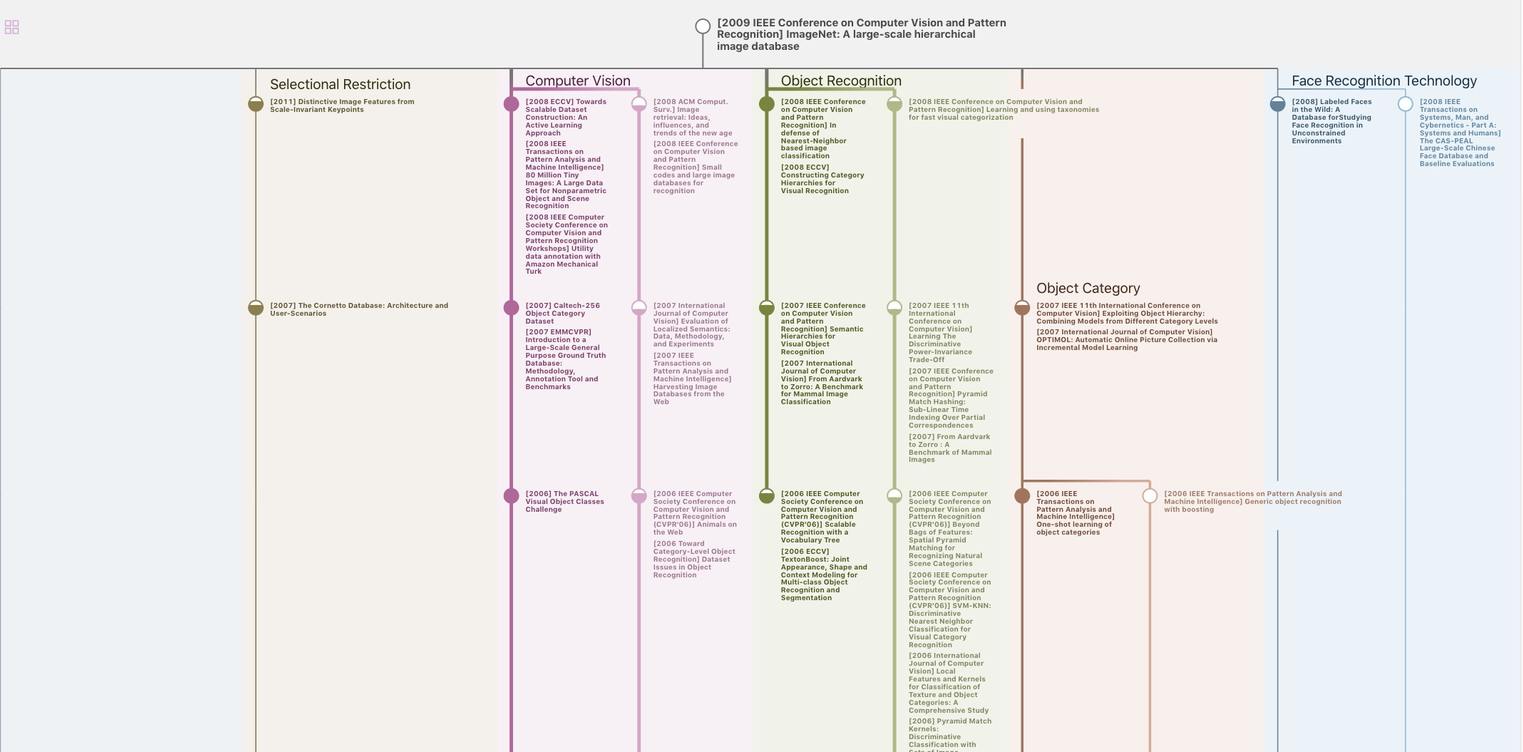
生成溯源树,研究论文发展脉络
Chat Paper
正在生成论文摘要