Attention-guided Multi-step Fusion: A Hierarchical Fusion Network for Multimodal Recommendation.
PROCEEDINGS OF THE 46TH INTERNATIONAL ACM SIGIR CONFERENCE ON RESEARCH AND DEVELOPMENT IN INFORMATION RETRIEVAL, SIGIR 2023(2023)
摘要
The main idea of multimodal recommendation is the rational utilization of the item's multimodal information to improve the recommendation performance. Previous works directly integrate item multimodal features with item ID embeddings, ignoring the inherent semantic relations contained in the multimodal features. In this paper, we propose a novel and effective aTtention-guided Multi-step FUsion Network for multimodal recommendation, named TMFUN. Specifically, our model first constructs modality feature graph and item feature graph to model the latent item-item semantic structures. Then, we use the attention module to identify inherent connections between user-item interaction data and multimodal data, evaluate the impact of multimodal data on different interactions, and achieve early-step fusion of item features. Furthermore, our model optimizes item representation through the attention-guided multi-step fusion strategy and contrastive learning to improve recommendation performance. The extensive experiments on three real-world datasets show that our model has superior performance compared to the state-of-the-art models.
更多查看译文
关键词
Feature graph,Attention,Multi-step fusion,Multimodal recommendation
AI 理解论文
溯源树
样例
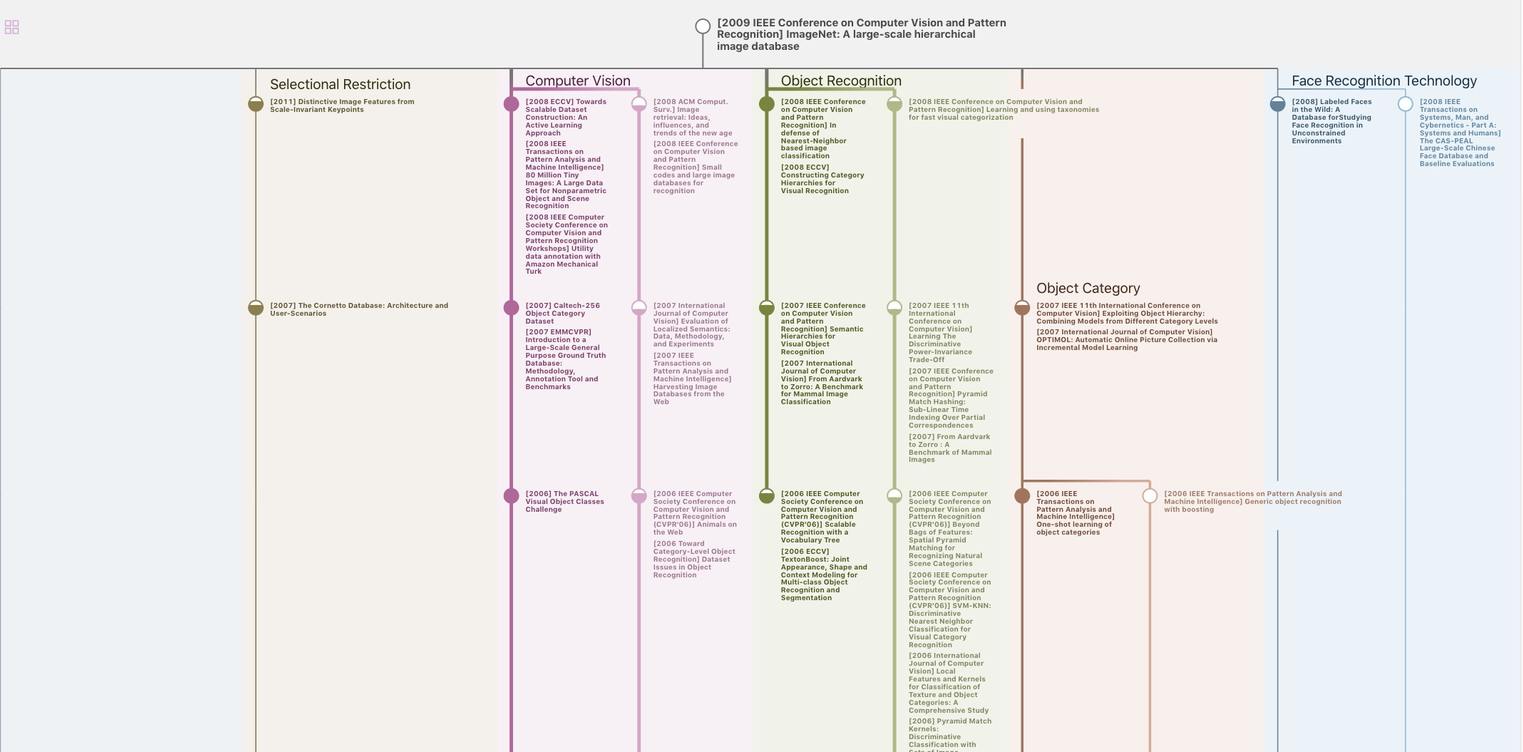
生成溯源树,研究论文发展脉络
Chat Paper
正在生成论文摘要