Identifying metal binding amino acids based on backbone geometries as a tool for metalloprotein engineering.
PROTEIN SCIENCE(2021)
摘要
Metal cofactors within proteins perform a versatile set of essential cellular functions. In order to take advantage of the diverse functionality of metalloproteins, researchers have been working to design or modify metal binding sites in proteins to rationally tune the function or activity of the metal cofactor. This study has performed an analysis on the backbone atom geometries of metal-binding amino acids among 10 different metal binding sites within the entire protein data bank. A set of 13 geometric parameters (features) was identified that is capable of predicting the presence of a metal cofactor in the protein structure with overall accuracies of up to 97% given only the relative positions of their backbone atoms. The decision tree machine-learning algorithm used can quickly analyze an entire protein structure for the presence of sets of primary metal coordination spheres upon mutagenesis, independent of their original amino acid identities. The methodology was designed for application in the field of metalloprotein engineering. A cluster analysis using the data set was also performed and demonstrated that the features chosen are useful for identifying clusters of structurally similar metal-binding sites.
更多查看译文
关键词
machine learning, metal binding, metalloproteins, protein engineering
AI 理解论文
溯源树
样例
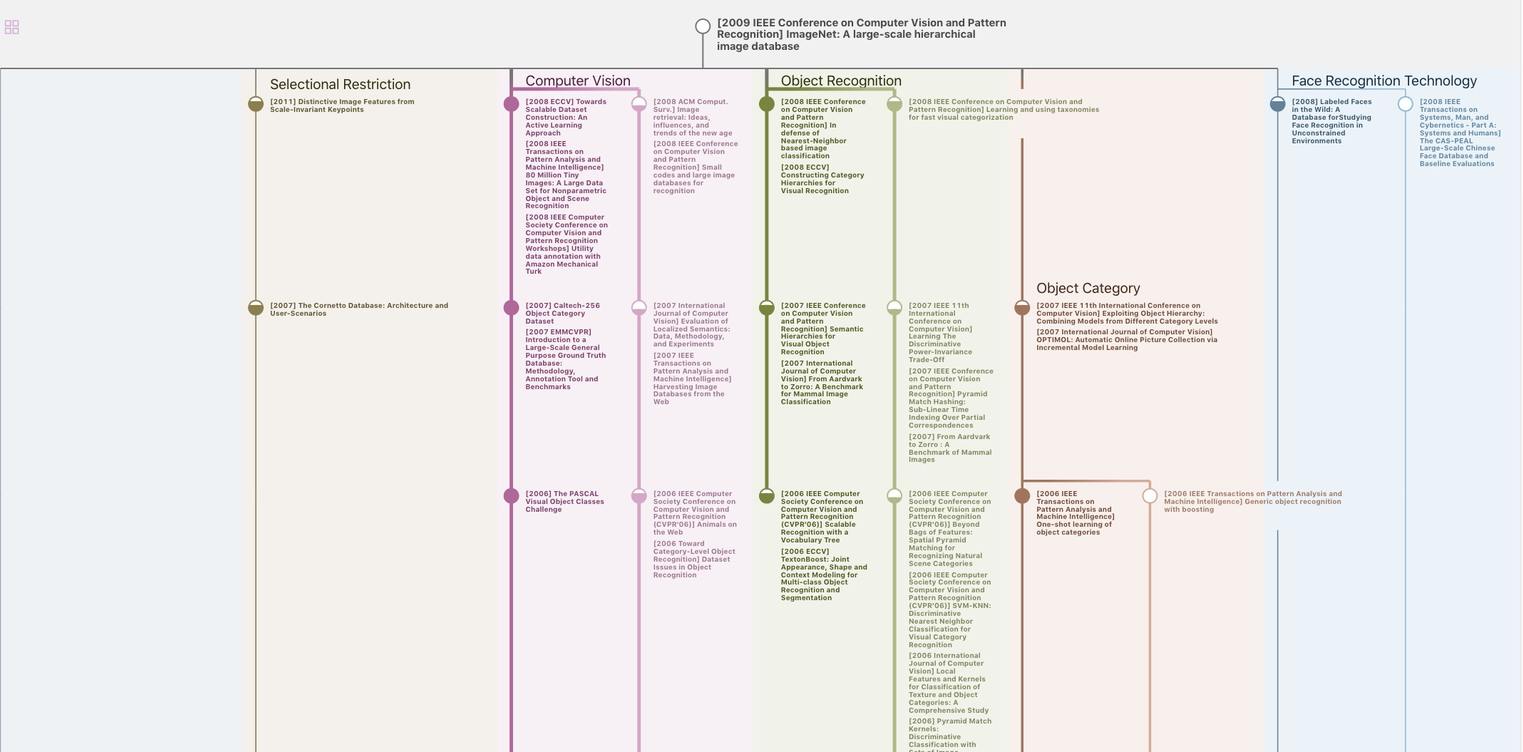
生成溯源树,研究论文发展脉络
Chat Paper
正在生成论文摘要