Lightweight, Embeddings Based Storage and Model Construction Over Satellite Data Collections
2020 IEEE International Conference on Big Data (Big Data)(2020)
摘要
There has been a substantial growth in remotely sensed hyperspectral satellite imagery. These data offer opportunities to understand phenomena and inform decision making. The nature of these collections introduces challenges stemming from their volumes, variety, and spatiotemporal resolutions. The crux of this study is to facilitate effective training of deep learning models over satellite data collections. We describe our novel embeddings (multidimensional latent space representations) based approach to effectively support model training, refinement, and inferences. We rigorously explore several aspects relating to embeddings, including their dimensionality, single vs multiple bands, and preservation of inter-band metrics. We also incorporate support for transfer learning over spatiotemporal scopes to address issues relating to cold start and alleviate resource pressure. Our methodology addresses disk, network. CPU/GPU, and accuracy implications of several aspects relating to model construction. Our empirical benchmarks assess the suitability of our methodology using the MOMS and Sentinel-2 satellite data. We demonstrate that our methodology reduces storage requirements by more than 10,000x and reduces model construction times by 75%.
更多查看译文
关键词
hyperspectral satellite imagery,deep learning models,spatial computing,storage systems,spatiotemporal transfer learning
AI 理解论文
溯源树
样例
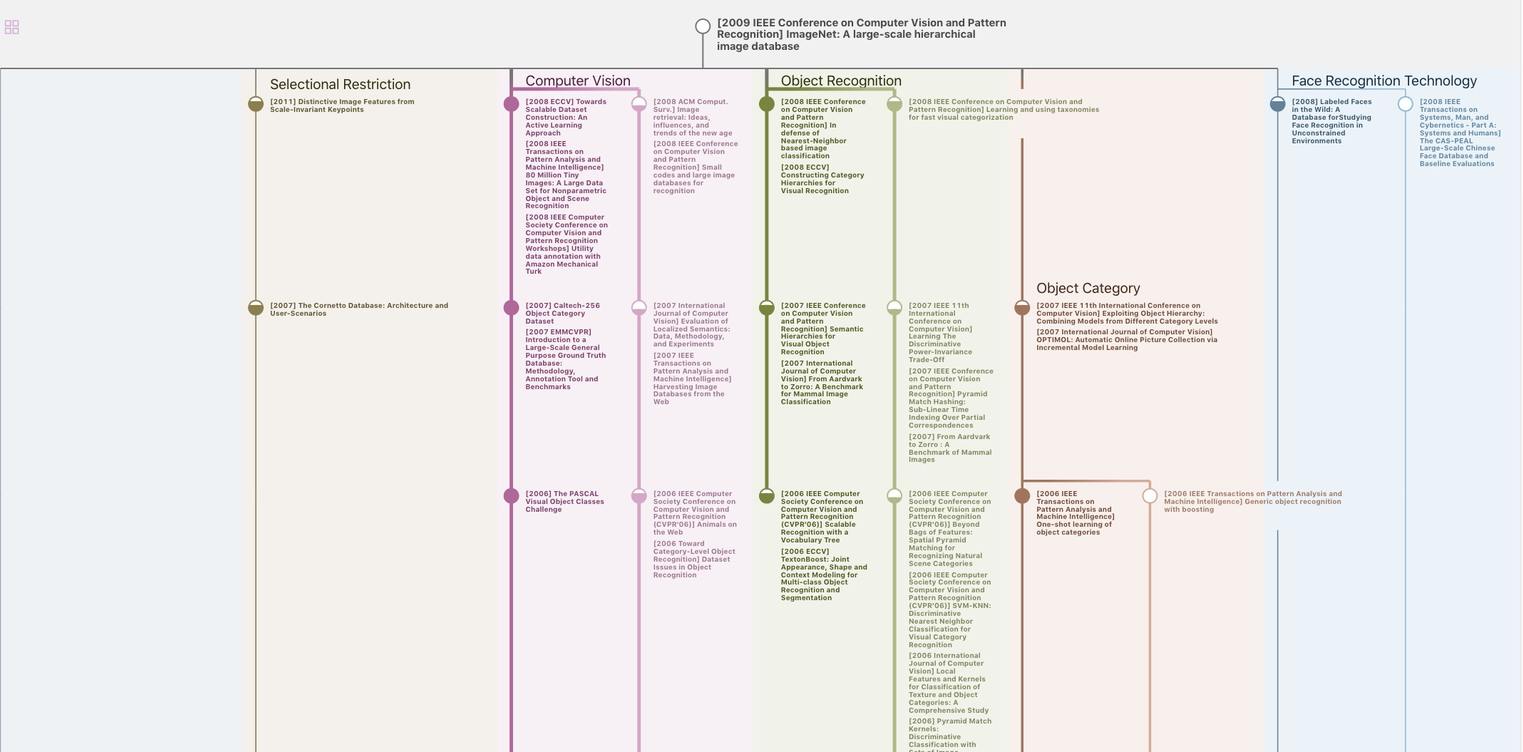
生成溯源树,研究论文发展脉络
Chat Paper
正在生成论文摘要