Tads: Transformation Of Anomalies In Data Streams
2020 IEEE INTERNATIONAL CONFERENCE ON BIG DATA (BIG DATA)(2020)
摘要
Detecting anomalies, in many cases, is only the first step. Often, it is merely the first step in a process that seeks to recover from an anomalous situation so that the system is back to a normal state. Manual analysis is a possible approach; however, this can be time consuming and error prone. Ideally, it would be desirable to have an automated means for resolving anomalies, which is invoked whenever one or more anomalies are detected. In this paper, we present a tool, TADS (Transformation of Anomalies in Data Streams), that creates automated recommendations based upon the observed streaming data. This is accomplished by utilizing the recently introduced concept of dynamic action rules with the Massive Online Analysis (MOA) data streaming platform. In experimental results, we demonstrate that TADS is able to correctly generate recommendations 100% of the time for over half the experiments and over 90% for all but two experimental conditions. In addition, only seconds are required to generate the personalized recommendation for each anomaly. Hence, the results indicate that TADS is a viable approach for correcting anomalies within a streaming environment.
更多查看译文
关键词
data streaming, anomaly recovery, anomaly detection, dynamic action rules
AI 理解论文
溯源树
样例
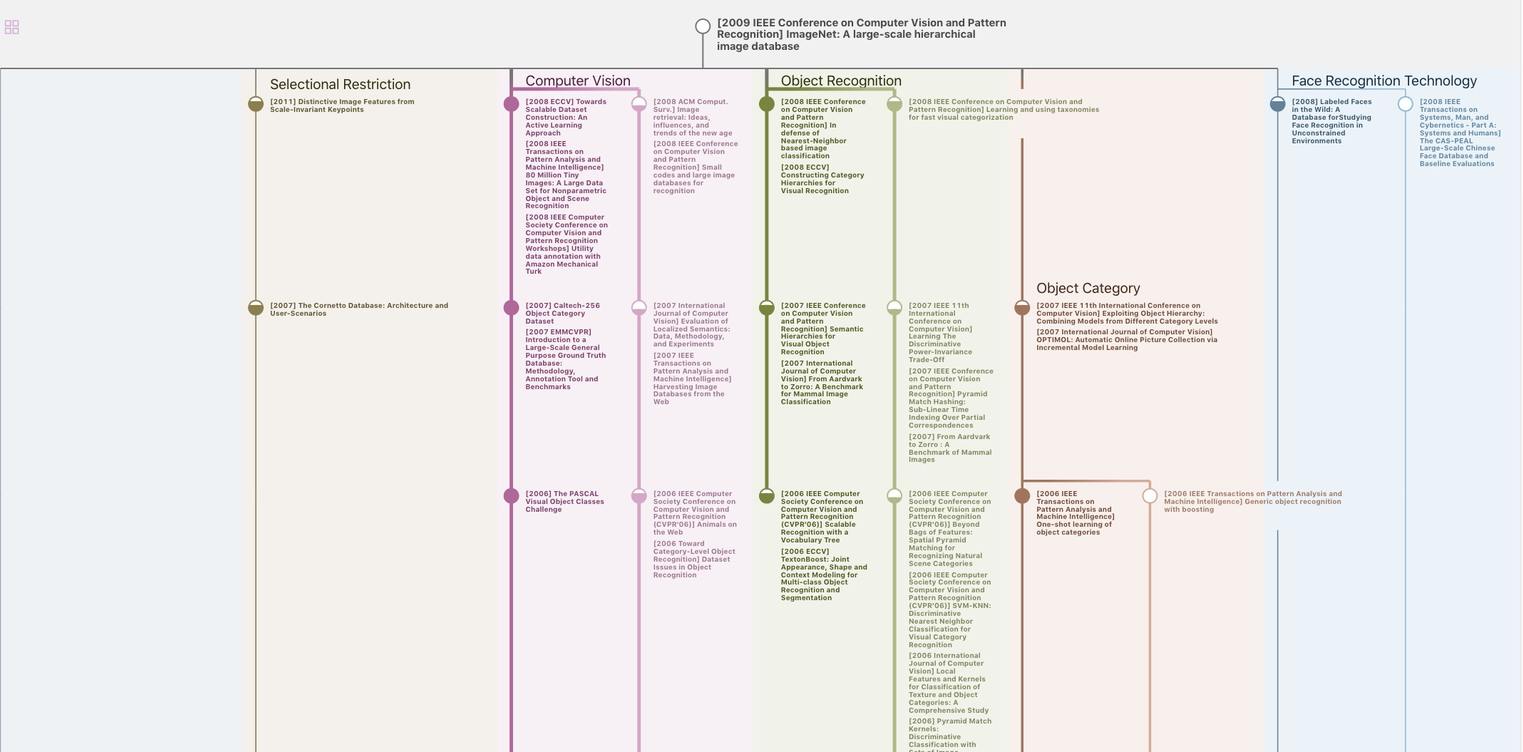
生成溯源树,研究论文发展脉络
Chat Paper
正在生成论文摘要