GLIMA: Global and Local Time Series Imputation with Multi-directional Attention Learning
2020 IEEE International Conference on Big Data (Big Data)(2020)
摘要
Missing data, which commonly appears in multivariate time series, has been widely recognized as a key challenge in time series analysis. Many commonly used imputation methods either ignore the temporal dependencies of time series data, or do not adequately utilize the relationships among variables. State-ofthe-art methods on time series imputation are built on Recurrent Neural Networks (RNNs), which utilize the historical information to estimate current values sequentially. However, RNNs rely heavily on the output of nearby timestamps, which may lead to important information lost for long sequences. Moreover, individual variables typically present different dynamics and missingness patterns, which is neglected by the global RNN hidden states. In this paper, we propose an imputation framework to learn both global and local dependencies of multivariate time series, as well as a multi-dimensional self-attention to learn capture distant correlations across both time and feature. Extensive experiments show that the proposed framework outperforms the state-of-the-art methods in the imputation task, and benefits the downstream task.
更多查看译文
关键词
Time Series,Missing Data,Recurrent Imputation,Self-Attention
AI 理解论文
溯源树
样例
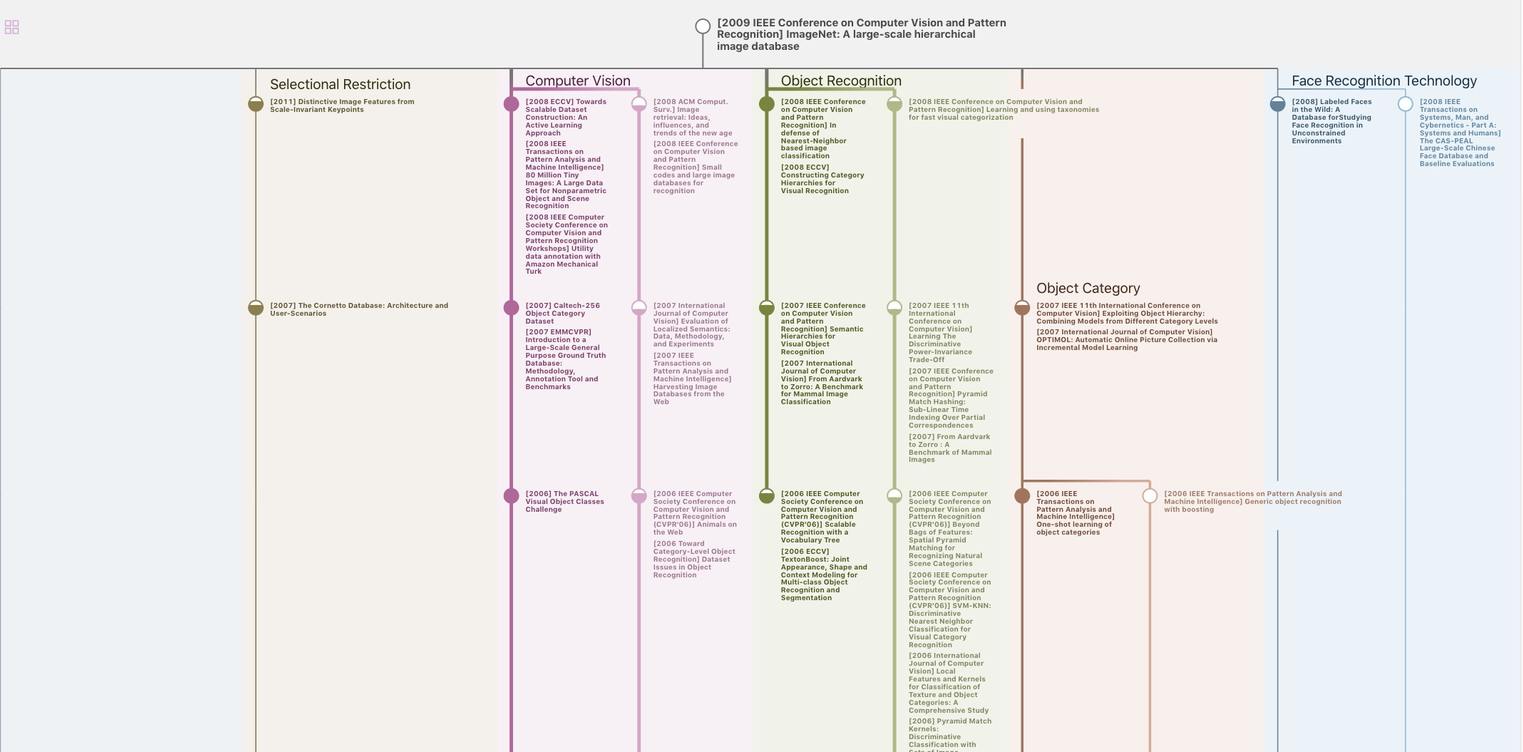
生成溯源树,研究论文发展脉络
Chat Paper
正在生成论文摘要