Residual Neural Network Architectures to Improve Prediction Accuracy of Properties of Materials
2020 IEEE INTERNATIONAL CONFERENCE ON BIG DATA (BIG DATA)(2020)
摘要
Properties in material composition and crystal structures have been explored by density functional theory (DFT) calculations, using databases such as the Open Quantum Materials Database (OQMD). Databases like these have been used currently for the training of advanced machine learning and deep neural network models, the latter providing higher performance when predicting properties of materials. However, current alternatives have shown a deterioration in accuracy when increasing the number of layers in their architecture (over-fitting problem). As an alternative method to address this problem, we have implemented residual neural network architectures based on Merge and Run Networks, IRNet and UNet to improve performance while relaxing the observed network depth limitation. The evaluation of the proposed architectures include a 9:1 ratio to train and test as well as 10 fold cross validation. In the experiments we found that our proposed architectures based on IRNet and UNet are able to obtain a lower Mean Absolute Error (MAE) than current strategies. The full implementation (Python, Tensorflow and Keras) and the trained networks will be available online for community validation and advancing the state of the art from our findings.
更多查看译文
关键词
material properties, elemental compositions, residual neural networks
AI 理解论文
溯源树
样例
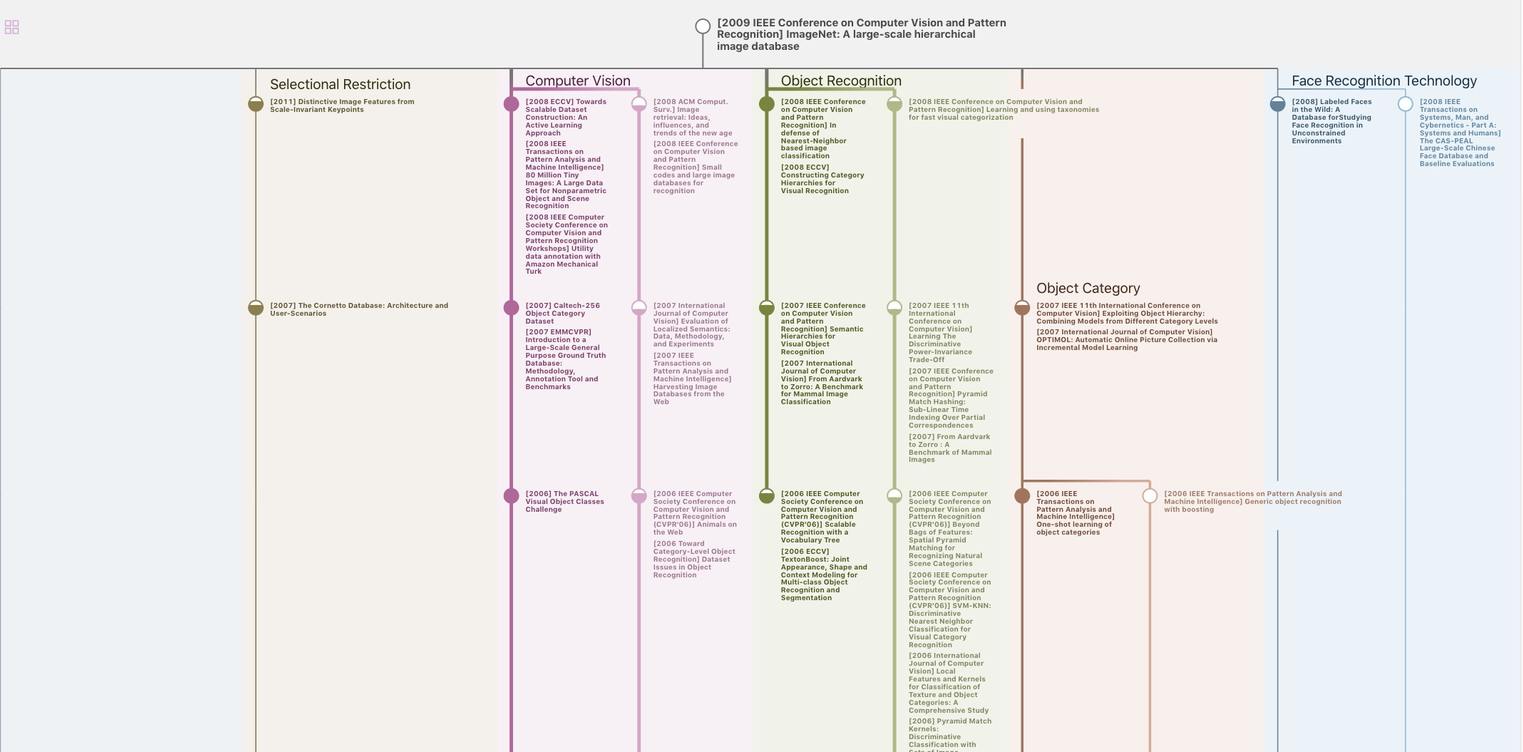
生成溯源树,研究论文发展脉络
Chat Paper
正在生成论文摘要