How Accurate Is An Unmanned Aerial Vehicle Data-Based Model Applied On Satellite Imagery For Chlorophyll-A Estimation In Freshwater Bodies?
REMOTE SENSING(2021)
摘要
Optical sensors are increasingly sought to estimate the amount of chlorophyll a (chl_a) in freshwater bodies. Most, whether empirical or semi-empirical, are data-oriented. Two main limitations are often encountered in the development of such models. The availability of data needed for model calibration, validation, and testing and the locality of the model developed-the majority need a re-parameterization from lake to lake. An Unmanned aerial vehicle (UAV) data-based model for chl_a estimation is developed in this work and tested on Sentinel-2 imagery without any re-parametrization. The Ensemble-based system (EBS) algorithm was used to train the model. The leave-one-out cross validation technique was applied to evaluate the EBS, at a local scale, where results were satisfactory (R-2 = Nash = 0.94 and RMSE = 5.6 mu g chl_a L-1). A blind database (collected over 89 lakes) was used to challenge the EBS' Sentine-2-derived chl_a estimates at a regional scale. Results were relatively less good, yet satisfactory (R-2 = 0.85, RMSE= 2.4 mu g chl_a L-1, and Nash = 0.79). However, the EBS has shown some failure to correctly retrieve chl_a concentration in highly turbid waterbodies. This particularity nonetheless does not affect EBS performance, since turbid waters can easily be pre-recognized and masked before the chl_a modeling.
更多查看译文
关键词
Sentinel-2, unmanned aerial vehicle, remote sensing, chlorophyll-a, machine learning, ensemble-based system, freshwaters, water quality
AI 理解论文
溯源树
样例
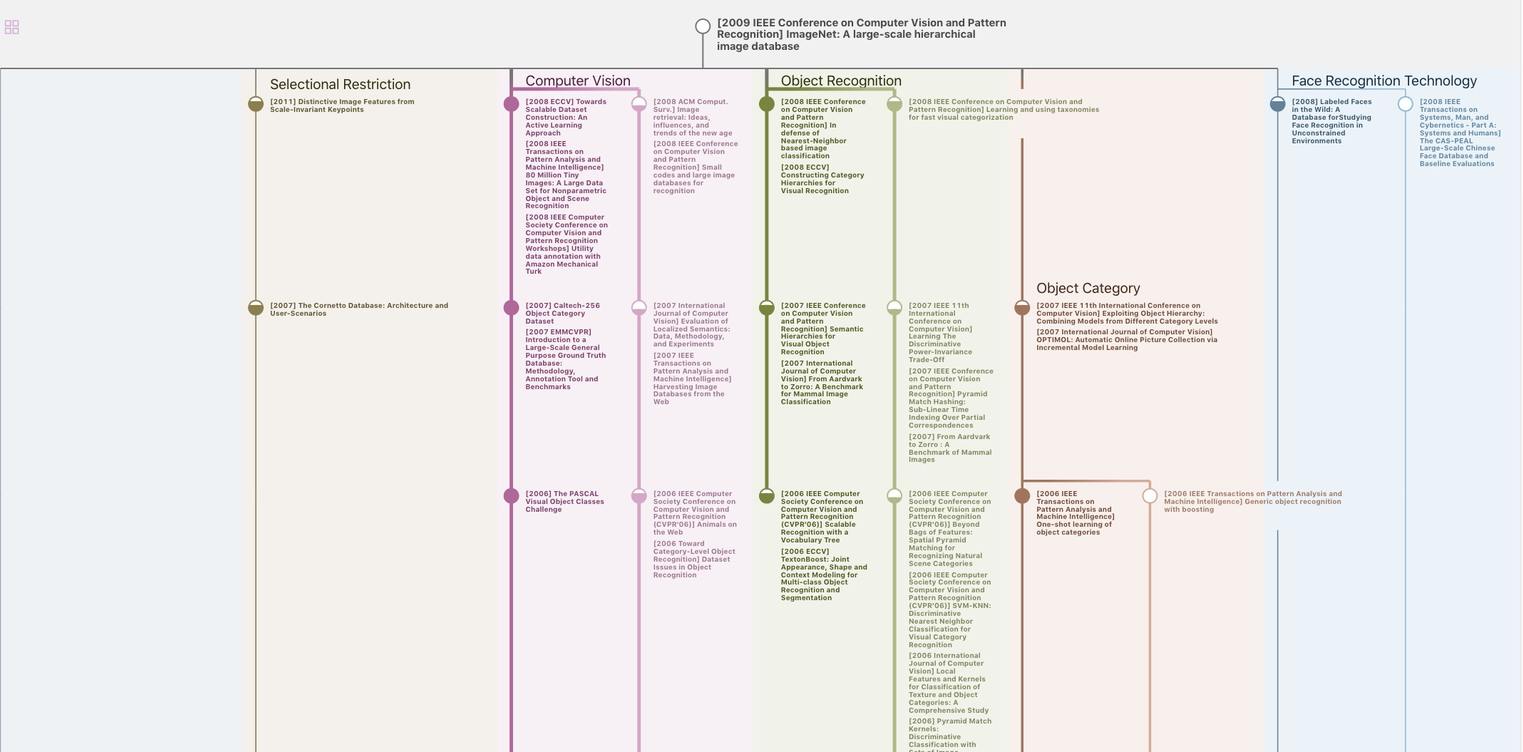
生成溯源树,研究论文发展脉络
Chat Paper
正在生成论文摘要