Learning-based Incast Performance Inference in Software-Defined Data Centers
2021 24th Conference on Innovation in Clouds, Internet and Networks and Workshops (ICIN)(2021)
摘要
Incast traffic is a many-to-one communication pattern used in many applications, including distributed storage, web-search with partition/aggregation design pattern, and MapReduce, commonly in data centers. It is generally composed of short-lived flows that may be queued behind large flows' packets in congested switches where performance degradation is observed. Smart buffering at the switch level is sensed to mitigate this issue by automatically and dynamically adapting to traffic conditions changes in the highly dynamic data center environment. But for this dynamic and smart buffer management to become effectively beneficial for all the traffic, and especially for incast the most critical one, incast performance models that provide insights on how various factors affect it are needed. The literature lacks these types of models. The existing ones are analytical models, which are either tightly coupled with a particular protocol version or specific to certain empirical data. Motivated by this observation, we propose a machine-learning-based incast performance inference. With this prediction capability, smart buffering scheme or other QoS optimization algorithms could anticipate and efficiently optimize system parameters adjustment to achieve optimal performance. Since applying machine learning to networks managed in a distributed fashion is hard, the prediction mechanism will be deployed on an SDN control plane. We could then take advantage of SDN's centralized global view, its telemetry capabilities, and its management flexibility.
更多查看译文
关键词
TCP Incast,Datacenters,SDN,Machine Learning,Performance Prediction,QoS
AI 理解论文
溯源树
样例
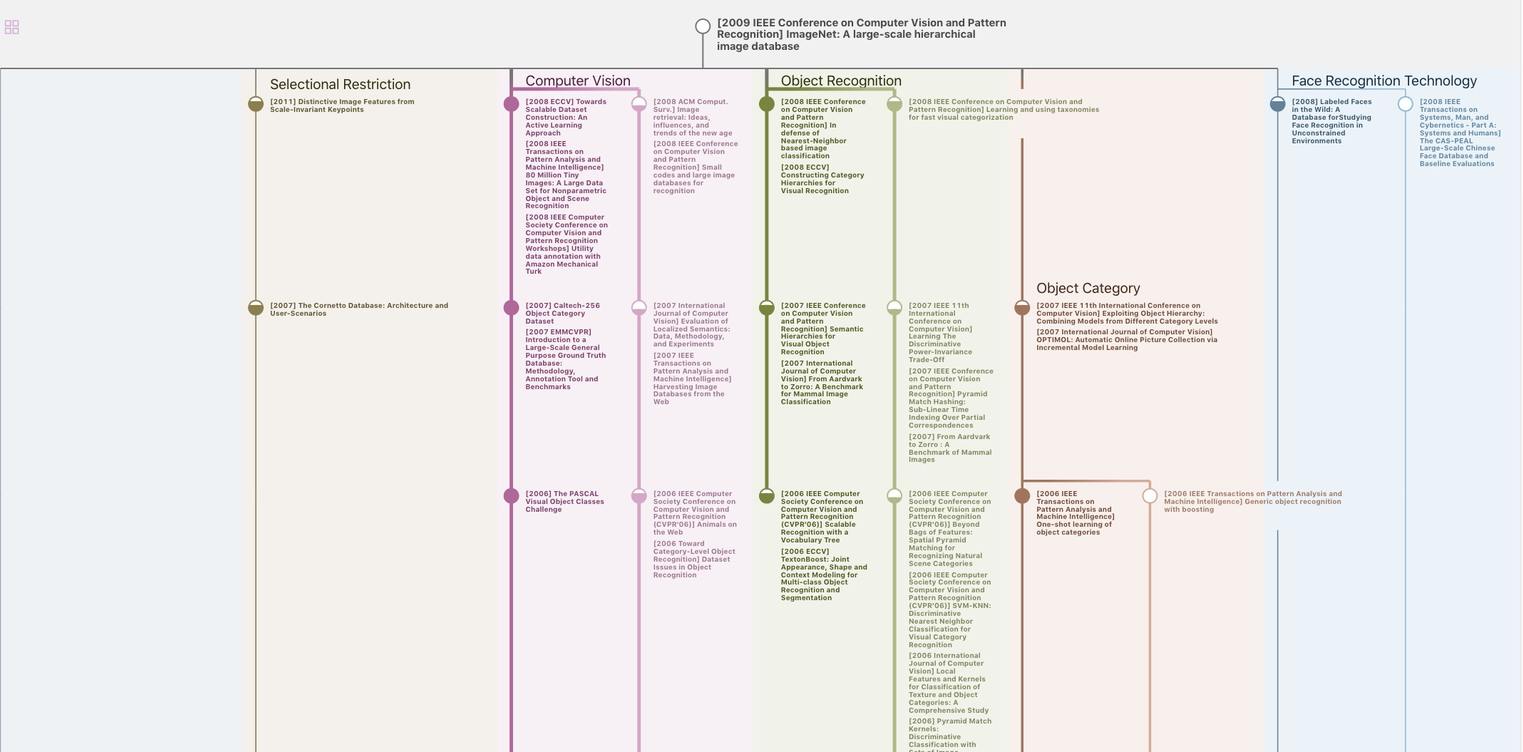
生成溯源树,研究论文发展脉络
Chat Paper
正在生成论文摘要