Dynamic Image Quantization Using Leaky Integrate-And-Fire Neurons
IEEE TRANSACTIONS ON IMAGE PROCESSING(2021)
摘要
This paper introduces a novel coding/decoding mechanism that mimics one of the most important properties of the human visual system: its ability to enhance the visual perception quality in time. In other words, the brain takes advantage of time to process and clarify the details of the visual scene. This characteristic is yet to be considered by the state-of-the-art quantization mechanisms that process the visual information regardless the duration of time it appears in the visual scene. We propose a compression architecture built of neuroscience models; it first uses the leaky integrate-and-fire (LIF) model to transform the visual stimulus into a spike train and then it combines two different kinds of spike interpretation mechanisms (SIM), the time-SIM and the rate-SIM for the encoding of the spike train. The time-SIM allows a high quality interpretation of the neural code and the rate-SIM allows a simple decoding mechanism by counting the spikes. For that reason, the proposed mechanisms is called Dual-SIM quantizer (Dual-SIMQ). We show that (i) the time-dependency of Dual-SIMQ automatically controls the reconstruction accuracy of the visual stimulus, (ii) the numerical comparison of Dual-SIMQ to the state-of-the-art shows that the performance of the proposed algorithm is similar to the uniform quantization schema while it approximates the optimal behavior of the non-uniform quantization schema and (iii) from the perceptual point of view the reconstruction quality using the Dual-SIMQ is higher than the state-of-the-art.
更多查看译文
关键词
Quantization (signal), Image reconstruction, Neurons, Visualization, Image coding, Transforms, Mathematical model, Uniform quantization, non-uniform quantization, leaky integrate-and-fire model, spikes, rate coding, time coding
AI 理解论文
溯源树
样例
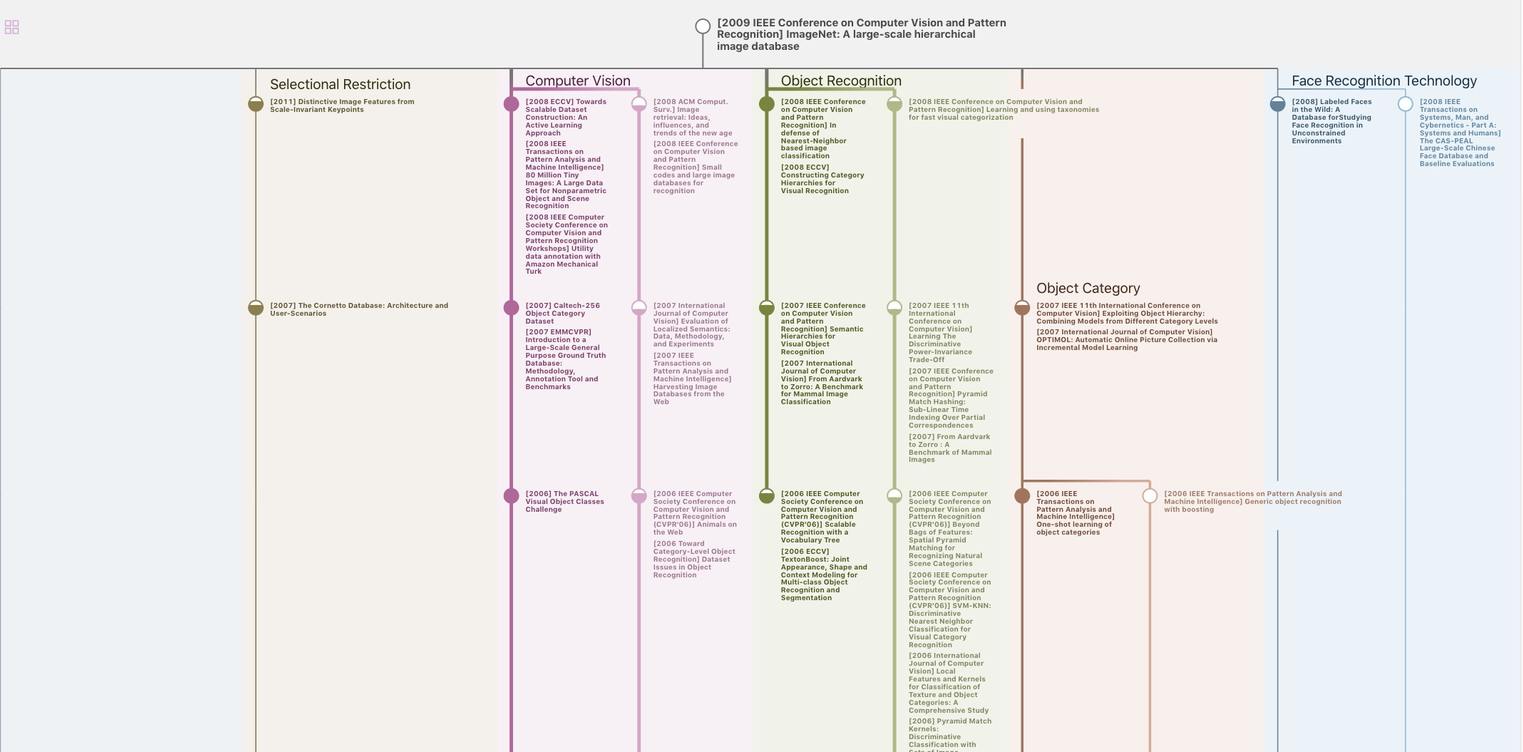
生成溯源树,研究论文发展脉络
Chat Paper
正在生成论文摘要