Individual Explanations in Machine Learning Models: A Survey for Practitioners
arxiv(2021)
摘要
In recent years, the use of sophisticated statistical models that influence decisions in domains of high societal relevance is on the rise. Although these models can often bring substantial improvements in the accuracy and efficiency of organizations, many governments, institutions, and companies are reluctant to their adoption as their output is often difficult to explain in human-interpretable ways. Hence, these models are often regarded as black-boxes, in the sense that their internal mechanisms can be opaque to human audit. In real-world applications, particularly in domains where decisions can have a sensitive impact—e.g., criminal justice, estimating credit scores, insurance risk, health risks, etc.—model interpretability is desired. Recently, the academic literature has proposed a substantial amount of methods for providing interpretable explanations to machine learning models. This survey reviews the most relevant and novel methods that form the state-of-the-art for addressing the particular problem of explaining individual instances in machine learning. It seeks to provide a succinct review that can guide data science and machine learning practitioners in the search for appropriate methods to their problem domain. Keywords— applied machine learning, interpretability, explainability, local explanations
更多查看译文
关键词
individual explanations,machine learning models
AI 理解论文
溯源树
样例
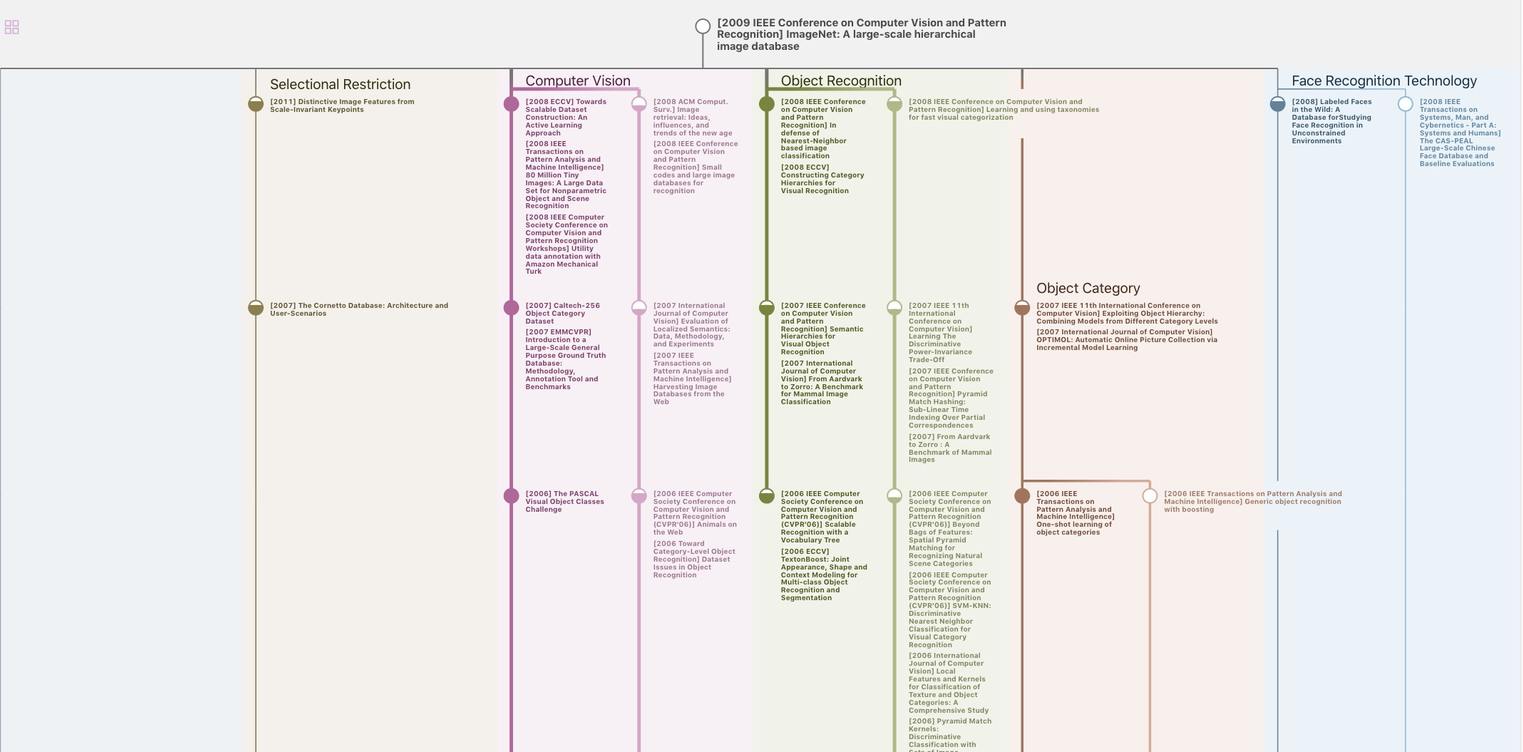
生成溯源树,研究论文发展脉络
Chat Paper
正在生成论文摘要