Individual Explanations in Machine Learning Models: A Case Study on Poverty Estimation
arxiv(2021)
摘要
Machine learning methods are being increasingly applied in sensitive societal contexts, where decisions impact human lives. Hence it has become necessary to build capabilities for providing easily-interpretable explanations of models' predictions. Recently in academic literature, a vast number of explanations methods have been proposed. Unfortunately, to our knowledge, little has been documented about the challenges machine learning practitioners most often face when applying them in real-world scenarios. For example, a typical procedure such as feature engineering can make some methodologies no longer applicable. The present case study has two main objectives. First, to expose these challenges and how they affect the use of relevant and novel explanations methods. And second, to present a set of strategies that mitigate such challenges, as faced when implementing explanation methods in a relevant application domain -- poverty estimation and its use for prioritizing access to social policies.
更多查看译文
关键词
individual explanations,poverty estimation,machine learning models
AI 理解论文
溯源树
样例
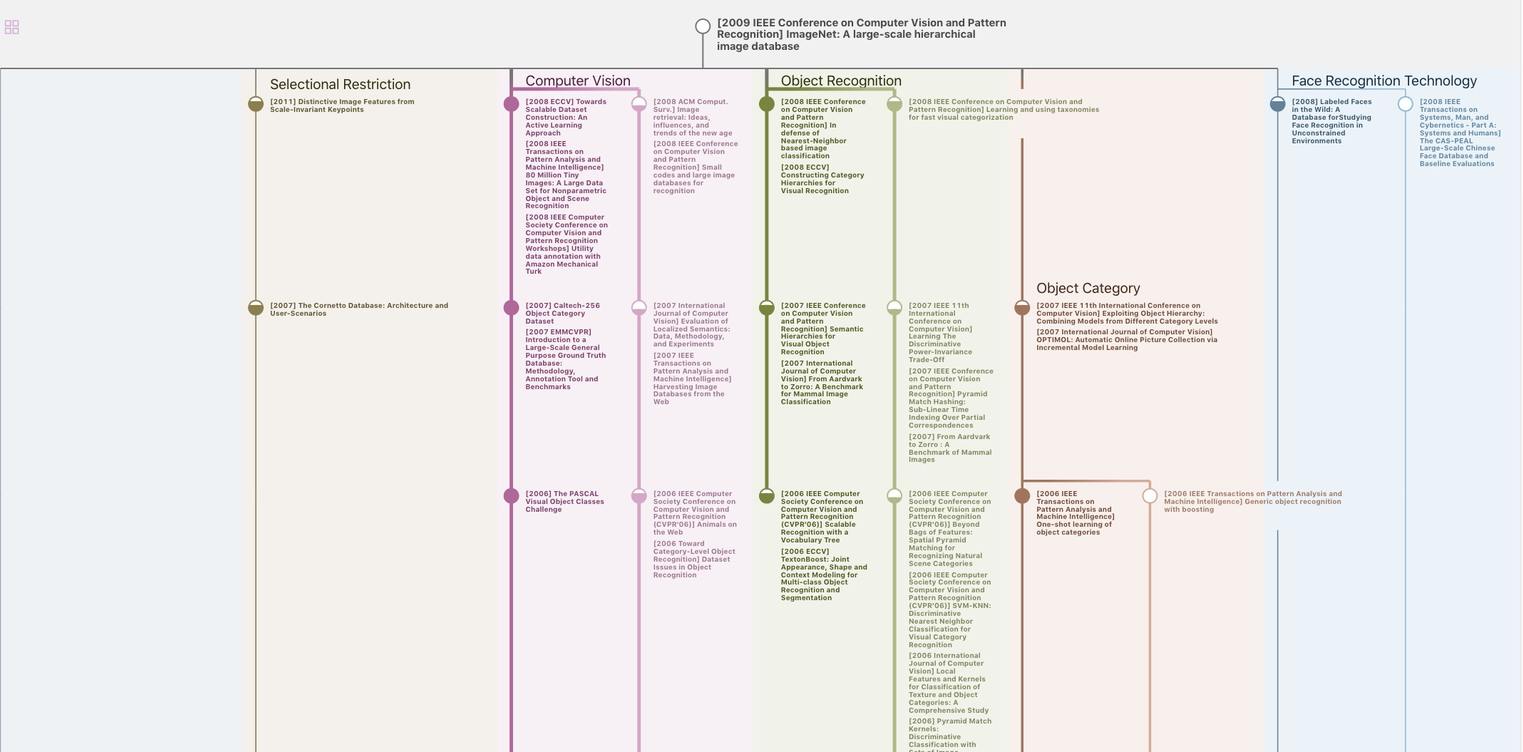
生成溯源树,研究论文发展脉络
Chat Paper
正在生成论文摘要