Bayesian Inference Over The Stiefel Manifold Via The Givens Representation
BAYESIAN ANALYSIS(2021)
摘要
We introduce an approach based on the Givens representation for posterior inference in statistical models with orthogonal matrix parameters, such as factor models and probabilistic principal component analysis (PPCA). We show how the Givens representation can be used to develop practical methods for transforming densities over the Stiefel manifold into densities over subsets of Euclidean space. We show how to deal with issues arising from the topology of the Stiefel manifold and how to inexpensively compute the change-of-measure terms. We introduce an auxiliary parameter approach that limits the impact of topological issues. We provide both analysis of our methods and numerical examples demonstrating the effectiveness of the approach. We also discuss how our Givens representation can be used to define general classes of distributions over the space of orthogonal matrices. We then give demonstrations on several examples showing how the Givens approach performs in practice in comparison with other methods.
更多查看译文
关键词
orthogonal matrix, transformation, dimensionality reduction, principal component analysis
AI 理解论文
溯源树
样例
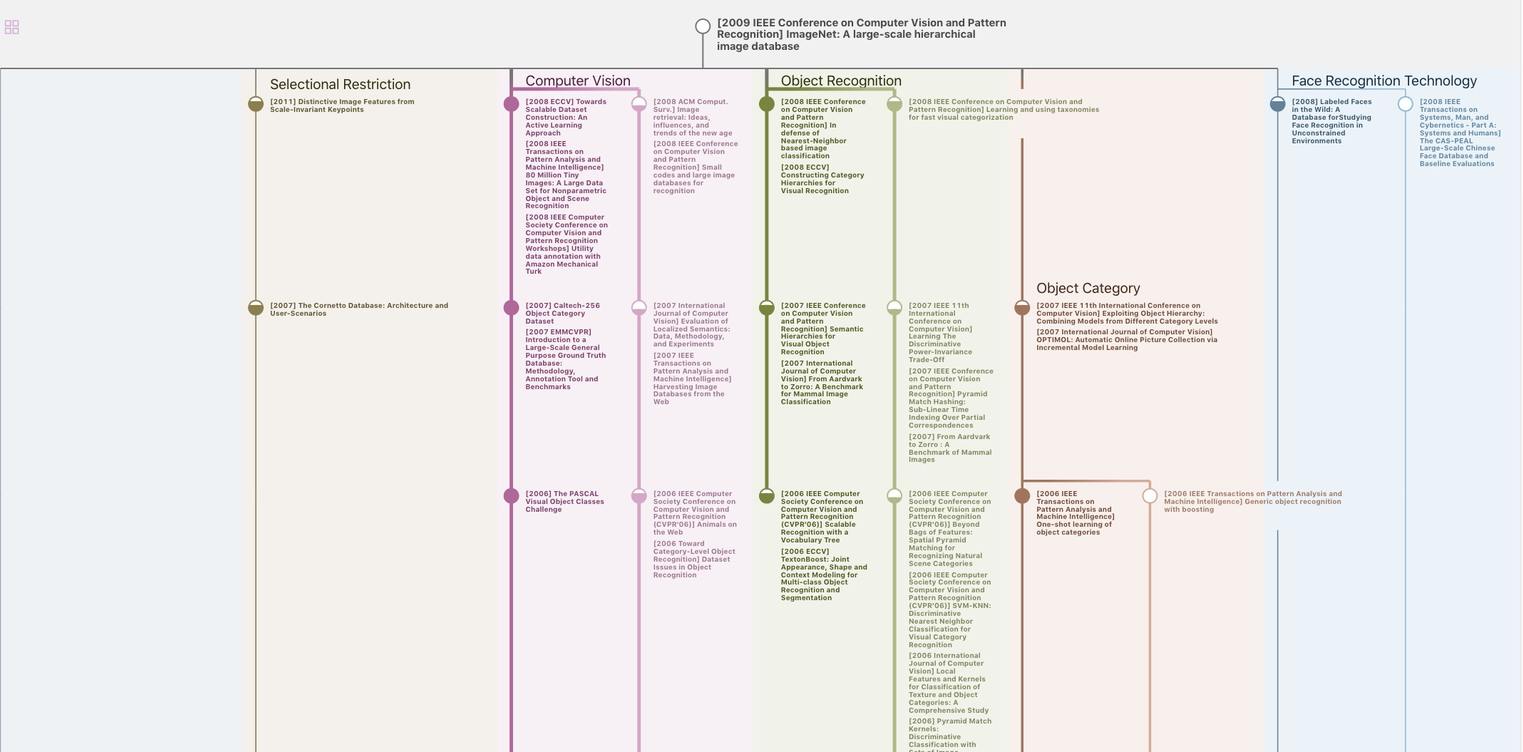
生成溯源树,研究论文发展脉络
Chat Paper
正在生成论文摘要