Optimization Of Information Gain In Multifidelity High-Speed Pressure Predictions
AIAA JOURNAL(2021)
摘要
The objective of multifidelity modeling is to achieve both accurate and efficient predictions by combining high- and low-fidelity models. A flexible approach considering additive, multiplicative, and input correction factors is proposed to improve the low-fidelity model using high-fidelity data. The correction factors are estimated using a Bayesian approach. Collection of high-fidelity data is optimized with two different objectives for comparison: by minimizing the error between synthetic data and the multifidelity prediction; and by maximizing the expected information gain, where the information gain is measured between the prior and posterior distributions. Once the multifidelity model is trained with optimal high-fidelity simulations, it is used to optimize the placement of sensors on a wind-tunnel test specimen, again by comparing the minimum expected error and the maximum expected information gain from the experiment. In this work, the prediction and measurement quantity of interest is aerodynamic pressure on rigid panel geometries in high-speed flow, and the multifidelity prediction model combines piston theory (low-fidelity) and Reynolds-averaged Navier-Stokes (high-fidelity) models.
更多查看译文
关键词
pressure,information gain,optimization,multi-fidelity,high-speed
AI 理解论文
溯源树
样例
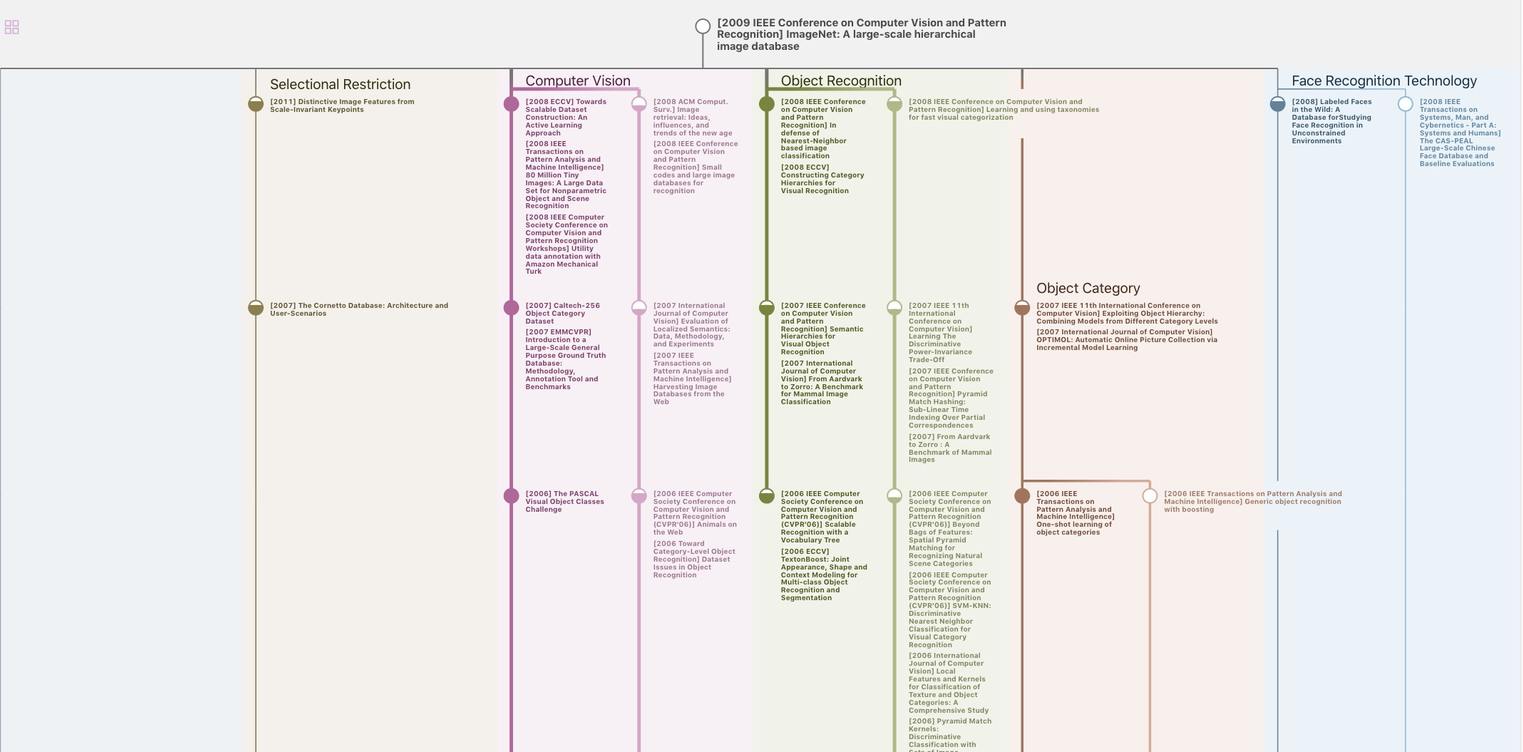
生成溯源树,研究论文发展脉络
Chat Paper
正在生成论文摘要