On maximum likelihood estimation of competing risks using the cause-specific semi-parametric Cox model with time-varying covariates - An application to credit risk
JOURNAL OF THE OPERATIONAL RESEARCH SOCIETY(2022)
摘要
Credit-granting institutions need to estimate the probability of loan default, which represents the chance a customer fails to make repayments as promised. Critically this estimation is intertwined with the competing risk a customer fully repays their loan while also having key predictive drivers with values that change over time. A conventional model in this setting is a competing risks Cox Model with time-varying covariates. However partial likelihood estimation of this model has two shortcomings: (1) the baseline hazard is not estimated, so calculating probabilities requires a further estimation step; and (2) a covariance matrix for both regression coefficients and the baseline hazard is not produced. This paper caters for these shortcomings by devising a maximum likelihood technique to jointly estimate regression coefficients and the cause-specific baseline hazards using constrained optimisation to ensure the latter's non-negativity. We show via simulation our technique produces regression coefficients estimates with lower bias in small samples with heavy censoring. When applied to a real-world credit risk dataset consisting of home loan data our Maximum Likelihood approach produces a smoother estimate of the cause-specific baseline hazards for default and redemption than those obtained using the Partial Likelihood and Breslow approach. This provides better clarity of the shape of these functions through both a less volatile central estimate as well as quantifying the error of this central estimate. We implement our method in R.
更多查看译文
关键词
Competing risk cause specific Cox models, time-varying covariates, constrained maximum likelihood optimisation, credit risk
AI 理解论文
溯源树
样例
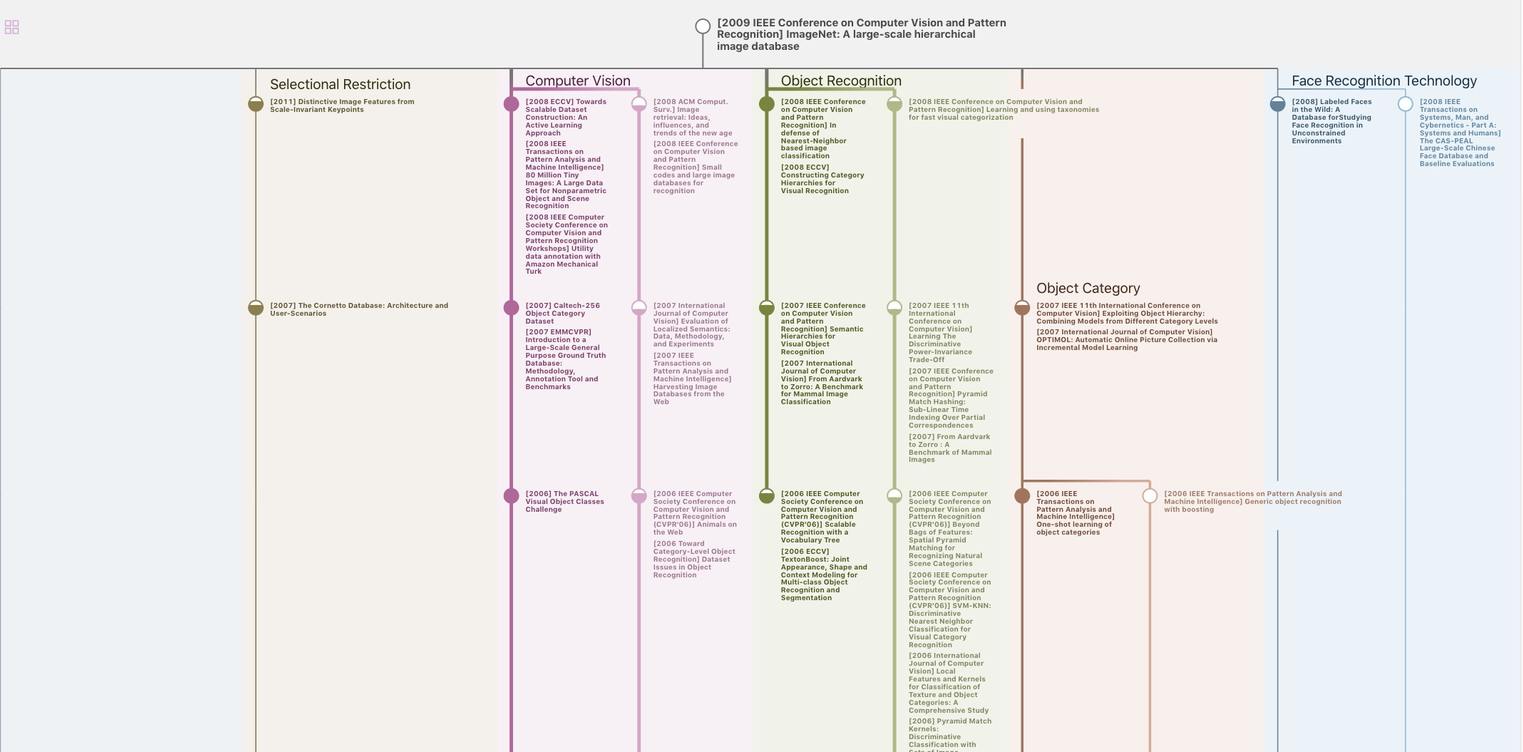
生成溯源树,研究论文发展脉络
Chat Paper
正在生成论文摘要