Assessment Of High-Resolution Dynamical And Machine Learning Models For Prediction Of Sea Ice Concentration In A Regional Application
JOURNAL OF GEOPHYSICAL RESEARCH-OCEANS(2020)
摘要
In this study, the potential for sea ice concentration prediction using machine-learning methods is investigated. Three different sea ice prediction models are compared: one high-resolution dynamical assimilative model and two statistical machine-learning models. The properties of all three models are explored, and the quality of their forecasts is compared. The dynamical model is a state-of-the-art coupled ocean and sea ice ensemble-prediction system with assimilation. The observations assimilated are high-resolution sea ice concentration from synthetic aperture radar (SAR) and sea surface temperature from infrared instruments. The machine-learning prediction models are a fully convolutional network and a k-nearest neighbors method. These methods use several variables as input for the prediction: sea ice concentration, sea surface temperature, and 2-m air temperature. Earlier studies have applied machine-learning approaches primarily for seasonal ice forecast. Here we focus on short-term predictions with a length of 1-4 weeks, which are of high interest for marine operations. The goal is to predict the future state of the sea ice using the same categories as traditional ice charts. The machine-learning forecasts were compared to persistence, which is the assumption that the sea ice does not change over the forecasting period. The machine-learning forecasts were found to improve upon persistence in periods of substantial change. In addition, compared to the dynamical model, the k-nearest neighbor algorithm was found to improve upon the 7-day forecast during a period of small sea ice variations. The fully convolutional network provided similar quality as the dynamical forecast. The study shows that there is a potential for sea ice predictions using machine-learning methods.
更多查看译文
关键词
Data Assimilation, Sea ice, Machine learning, Dynamical model, Forecast
AI 理解论文
溯源树
样例
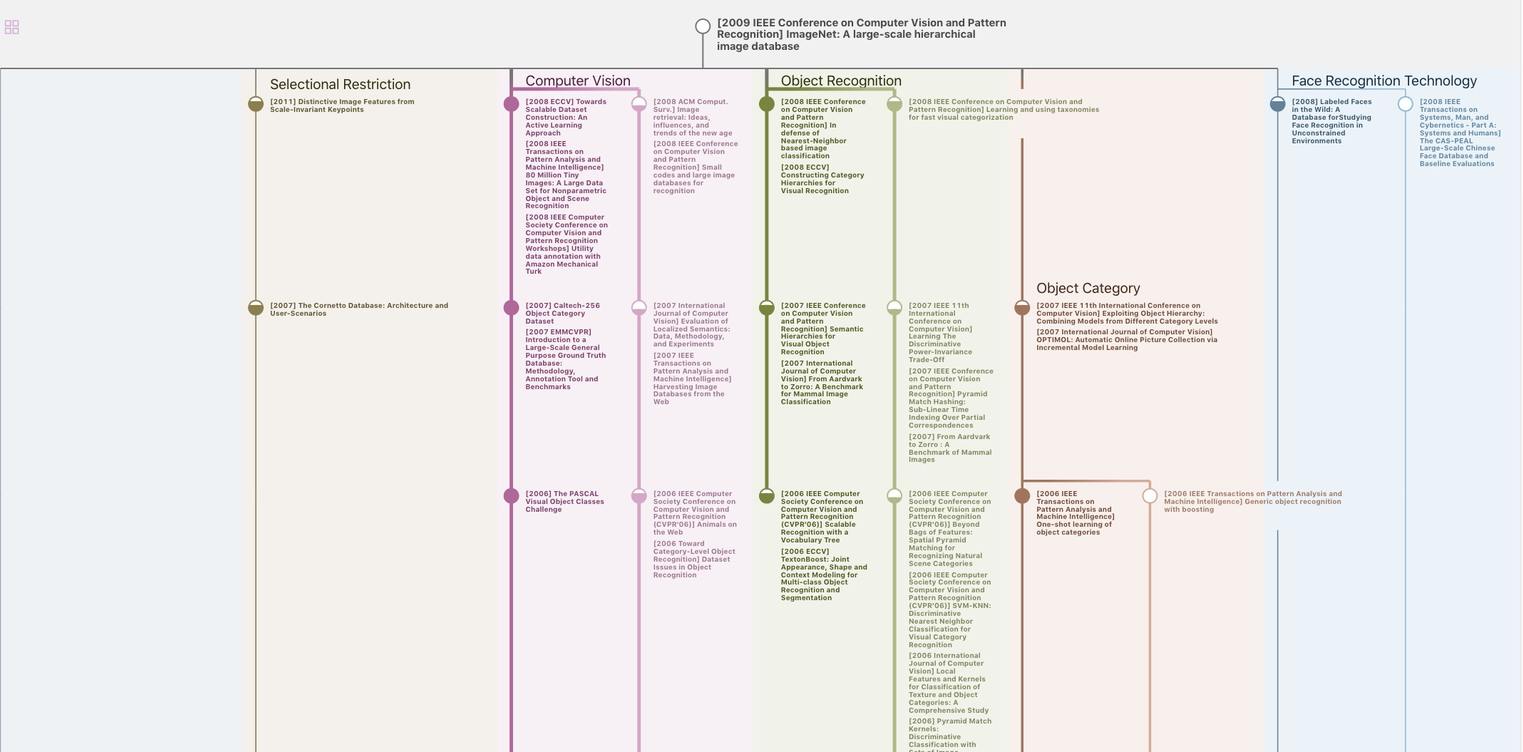
生成溯源树,研究论文发展脉络
Chat Paper
正在生成论文摘要