3D conditional generative adversarial network-based synthetic medical image augmentation for lung nodule detection
INTERNATIONAL JOURNAL OF IMAGING SYSTEMS AND TECHNOLOGY(2021)
Abstract
A computer-aided detection (CADe) scheme, relying on a large number of high-quality images with annotations, could help radiologists effectively detect lung nodules. However, such medical data are generally difficult to obtain. To address this issue, this paper proposes a novel method based on a conditional generative adversarial network (CGAN) to generate new samples for data augmentation (DA). This method employs a 3D CGAN based on a squeeze-and-excitation mechanism and residual learning to synthesize realistic and diverse lung nodules in chest computed tomography (CT) images to improve the performance of the CADe system. To evaluate the proposed method, this paper uses synthetic samples for DA to train the lung nodule detection network. The results indicate that these synthetic samples, which cover a part of the data distribution unfilled by the original data, can boost the overall performance of the nodule detection network at fixed false positive (FP) rates.
MoreTranslated text
Key words
3D squeeze-and-excitation network, computer-aided detection, conditional generative adversarial network, data augmentation, lung nodules
AI Read Science
Must-Reading Tree
Example
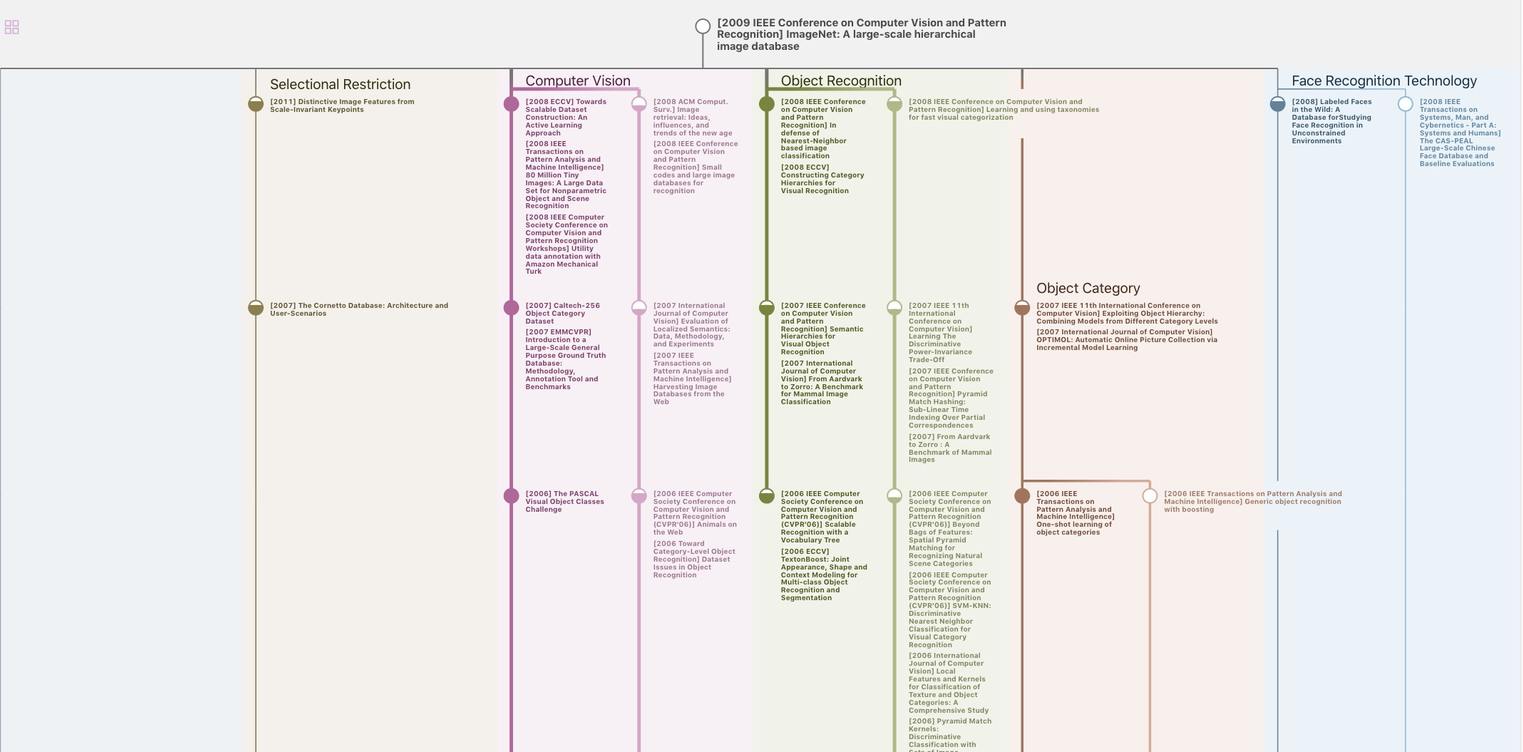
Generate MRT to find the research sequence of this paper
Chat Paper
Summary is being generated by the instructions you defined