Forecasting the ionospheric F2 Parameters over Jeju Station (33.43°N, 126.30°E) by Using Long Short-Term Memory
Journal of the Korean Physical Society(2020)
摘要
Ionospheric models aim to simulate and predict variations in the ionosphere. In this study, we attempt to develop a regional ionospheric model to predict hourly the foF2s (peak frequencies of the F2 layer) and the hmF2s (peak heights of the F2 layer) over the Jeju station (33.43°N, 126.30°E) for up to 24 hours by using a deep learning method. For forecasting the F2 parameters, we adopt the method of long short-term memory (LSTM), which, unlike traditional neural networks, can shed light on sequential variation in time-series data. The inputs of the LSTM model are sequential data for the past 24 hours, which includes the sunspot number (SSN), the daily F10.7 solar flux, geomagnetic the Ap and Kp indices, the foF2 and the hmF2. The foF2 and the hmF2 data used to train the model are measured by the Jeju ionosonde, operated by the Korean Space Weather Center (KSWC) during the period from 2011 through 2016. To evaluate our model performance, we utilized the root mean square error (RMSE) and the correlation coefficient (CC) as the performance skill scores between the model prediction and the measurement during the 338 days of 2017–2018. The best foF2 model, having 21 hidden neurons and 3 batch sizes, performed with an RMSE of 0.65 MHz and a CC of 0.92 when compared with the value measured during the next hour. The best hmF2 model, having 41 hidden neurons and 24 batch sizes, preformed with an RMSE of 23.8 km and a CC of 0.80. When the forecasting steps were increased, the performances gradually deteriorated. The LSTM model results were compared with the results of the TIE-GCM and the IRI-2016 model for geomagnetically quiet and active periods. On geomagnetic quiet days, the LSTM model showed the best performance among the three models. When the RMSEs of the foF2 (hmF2) predictions were compared, the percentage improvements of the LSTM model were 45% (30%) or more than those of the other models during geomagnetically quiet days. On geomagnetic storm days, however, the LSTM model did not improve predictions any better than the IRI-2016 model, which may be due to the limited amount of training data under the storm condition.
更多查看译文
关键词
Ionospheric model,Forecasting,Long short-term memory
AI 理解论文
溯源树
样例
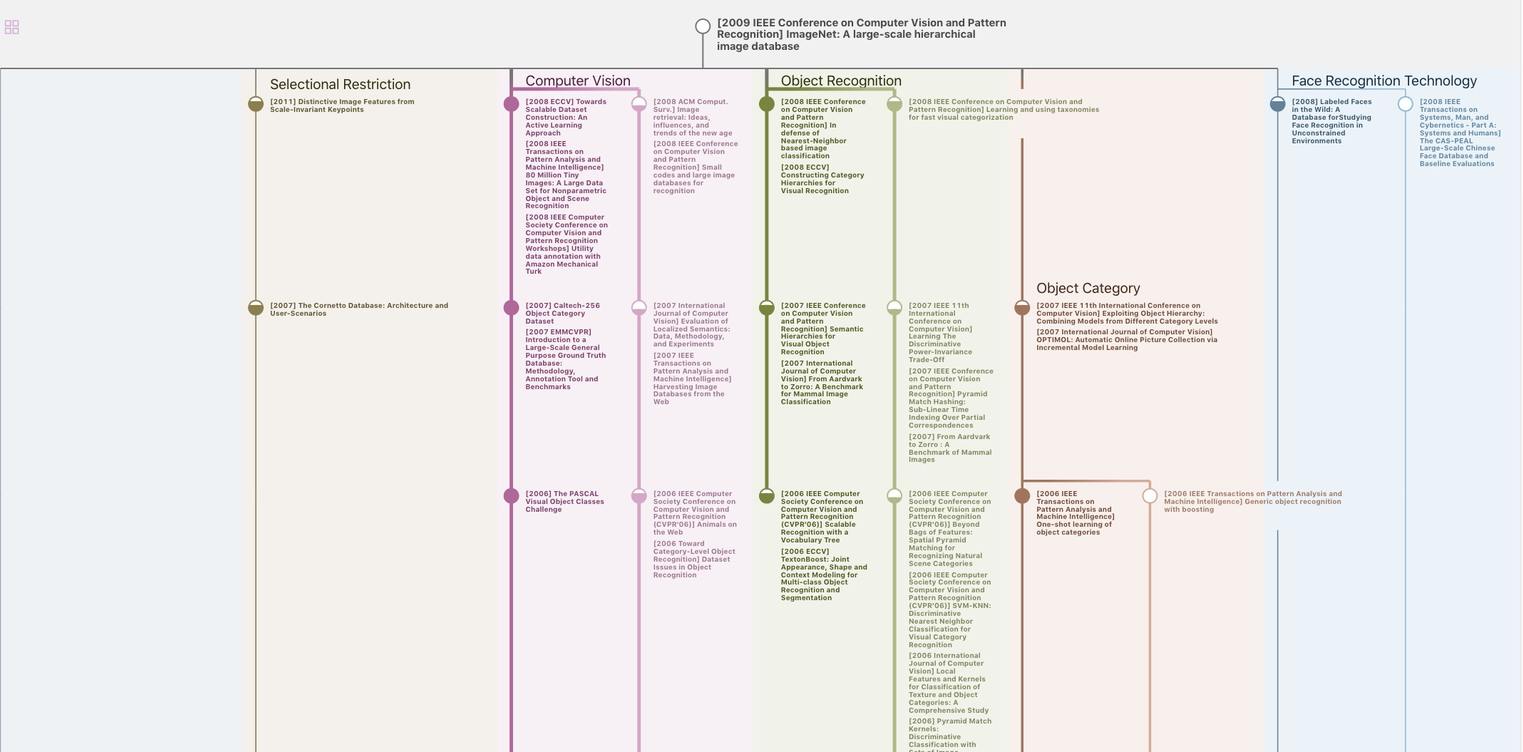
生成溯源树,研究论文发展脉络
Chat Paper
正在生成论文摘要