Inferring dynamic gene regulatory networks with low-order conditional independencies - an evaluation of the method
STATISTICAL APPLICATIONS IN GENETICS AND MOLECULAR BIOLOGY(2020)
摘要
Over a decade ago, Lebre (2009) proposed an inference method, G1DBN, to learn the structure of gene regulatory networks (GRNs) from high dimensional, sparse time-series gene expression data. Their approach is based on concept of low-order conditional independence graphs that they extend to dynamic Bayesian networks (DBNs). They present results to demonstrate that their method yields better structural accuracy compared to the related Lasso and Shrinkage methods, particularly where the data is sparse, that is, the number of time measurements n is much smaller than the number of genes p. This paper challenges these claims using a careful experimental analysis, to show that the GRNs reverse engineered from time-series data using the G1DBN approach are less accurate than claimed by Lebre (2009). We also show that the Lasso method yields higher structural accuracy for graphs learned from the simulated data, compared to the G1DBN method, particularly when the data is sparse (n < < p). The Lasso method is also better than G1DBN at identifying the transcription factors (TFs) involved in the cell cycle of Saccharomyces cerevisiae.
更多查看译文
关键词
conditional independence,dynamic Bayesian networks,gene networks,gene regulatory networks
AI 理解论文
溯源树
样例
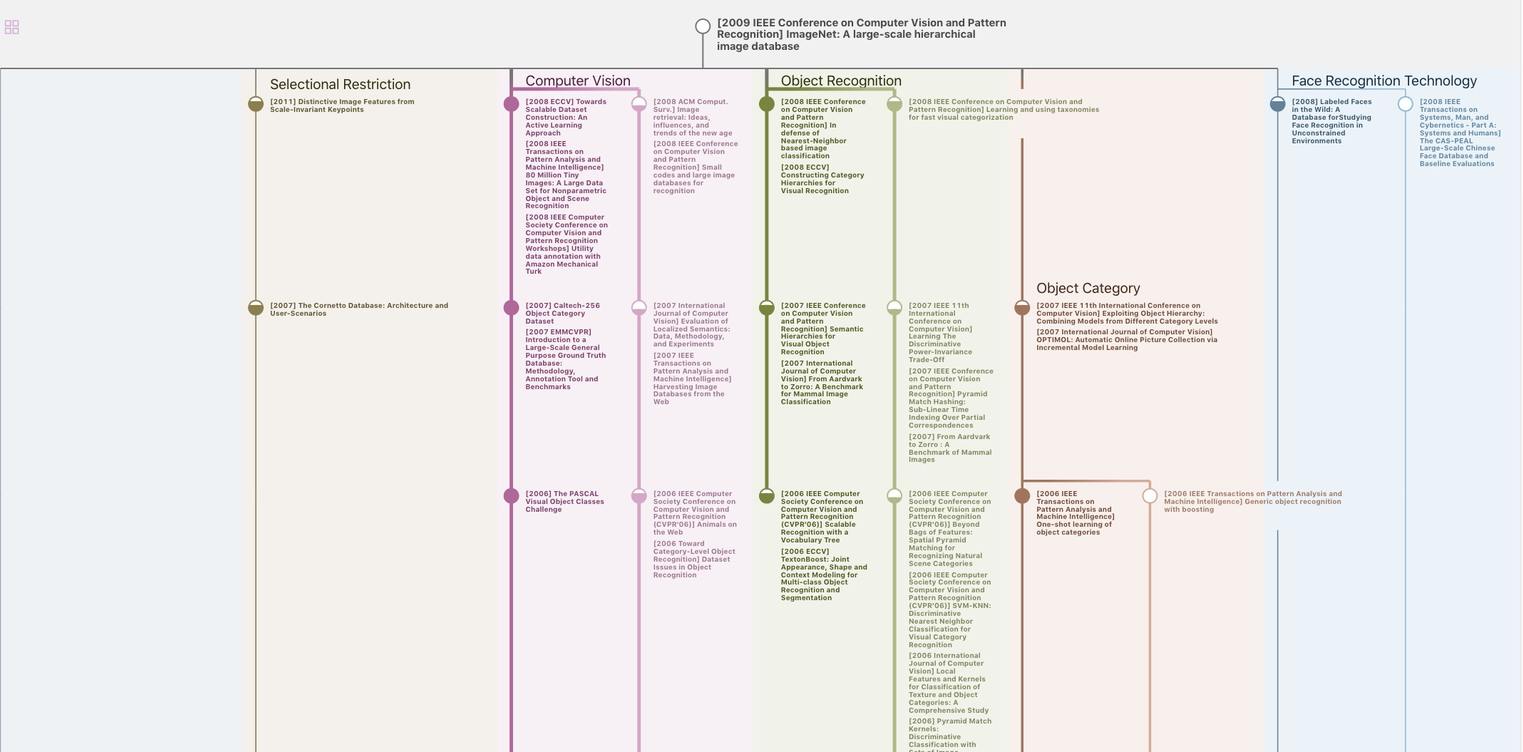
生成溯源树,研究论文发展脉络
Chat Paper
正在生成论文摘要