Performance of Predictive Indoor mmWave Networks With Dynamic Blockers
IEEE Transactions on Cognitive Communications and Networking(2022)
摘要
In this paper, we consider millimeter Wave (mmWave) technology to provide reliable wireless network service within factories where links may experience rapid and temporary fluctuations of the received signal power due to dynamic blockers, such as humans and robots, moving in the environment. We propose a novel beam recovery procedure that leverages Machine Learning (ML) tools to predict the starting and finishing of blockage events. This erases the delay introduced by current 5G New Radio (5G-NR) procedures when switching to an alternative serving base station and beam, and then re-establish the primary connection after the blocker has moved away. Firstly, we generate synthetic data using a detailed system-level simulator that integrates the most recent 3GPP 3D Indoor channel models and the geometric blockage Model-B. Then, we use the generated data to train offline a set of beam-specific Deep Neural Network (DNN) models that provide predictions about the beams’ blockage states. Finally, we deploy the DNN models online into the system-level simulator to evaluate the benefits of the proposed solution. Our prediction-based beam recovery procedure guarantees higher signal level stability and up to 82% data rate improvement with respect a detection-based method when blockers move at speed of 2 m/s.
更多查看译文
关键词
Millimeter-wave,5G new radio,dynamic blockage,machine learning,deep neural network
AI 理解论文
溯源树
样例
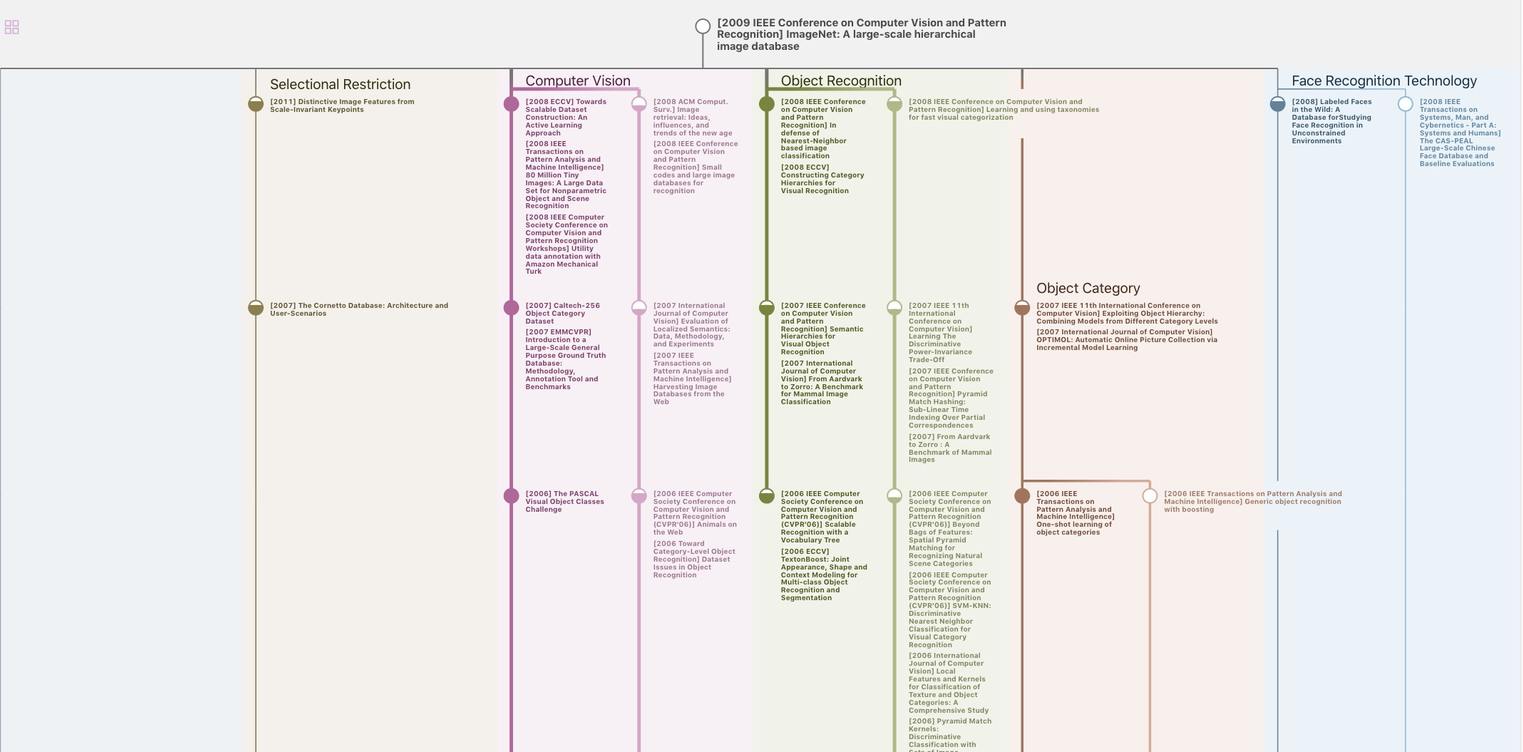
生成溯源树,研究论文发展脉络
Chat Paper
正在生成论文摘要